Transfer Learning-based SAE-CNN for Industrial Data Processing in Multiple working Conditions Recognition
2022 IEEE International Conference on Prognostics and Health Management (ICPHM)(2022)
摘要
In current industrial production, the acquisition and processing of large amounts of sensor monitoring data have become an important component of equipment monitoring. Artificial intelligence approaches have the advantage in the management ability of big data from manufacturing processes and are thus increasingly used in industry. However, the artificial intelligence methods are suitable for specific production lines and hardly generalizable to new situations. To solve these problems, this paper proposes a transfer learning-based Stacked Auto-encoder (SAE) with Convolutional Neural Network (CNN), which simplifies high-dimensional data into generalization and effective features that characterize the machine tool's working state. First, it uses an unsupervised SAE for general feature extraction for monitoring data from different production lines. Second, a few supervised data are used to fine-turning a working condition classifier composed of a one-dimensional Convolutional Neural Network to verify the performance of the model in the new production line. The proposed method reduces the amount of labeled training data while at the same time enhancing the accuracy. The experiment result shows a better performance of the proposed method, which suggests a strong potential for applications in pattern recognition during sheet metal forming processes.
更多查看译文
关键词
transfer learning,industrial data processing,stacked auto-encoder,data dimensionality reduce
AI 理解论文
溯源树
样例
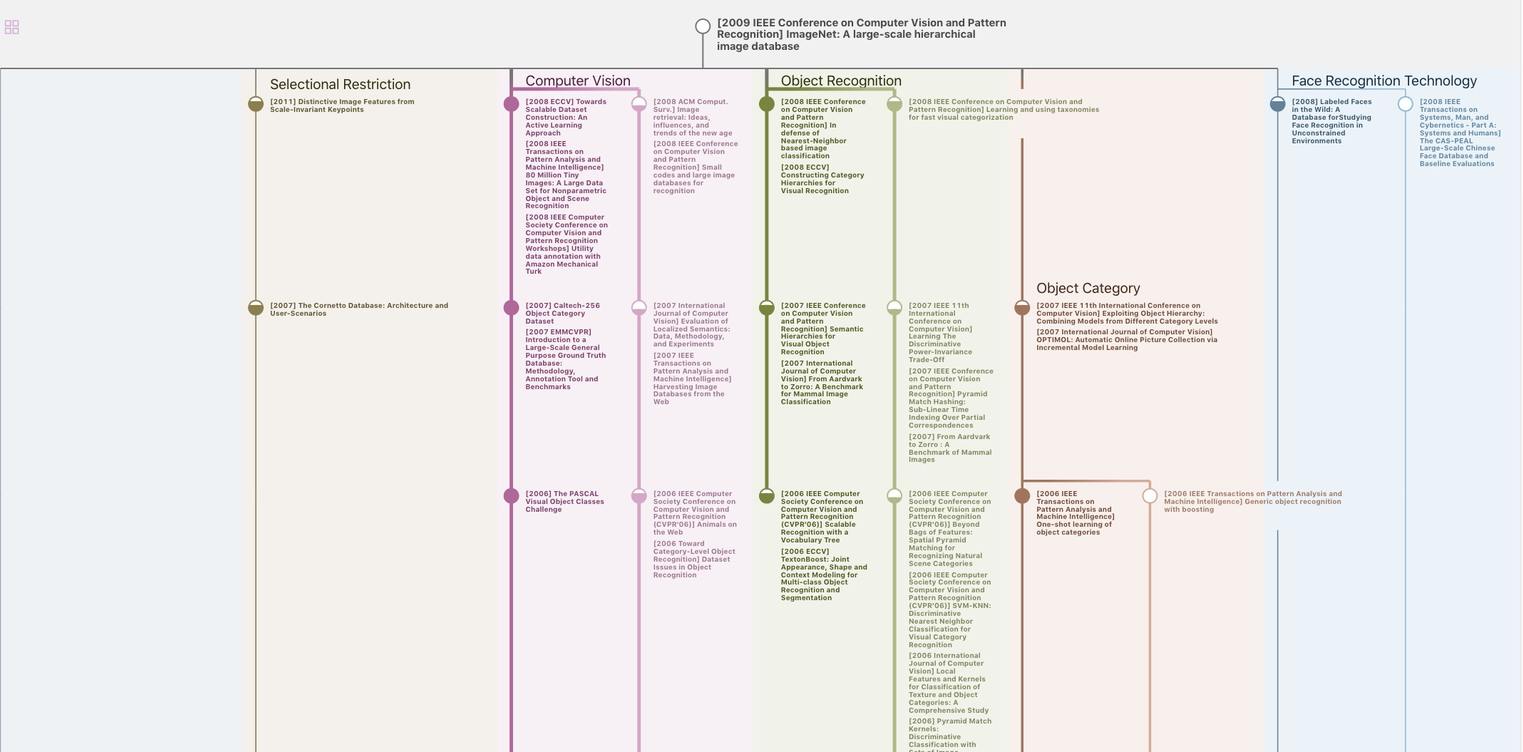
生成溯源树,研究论文发展脉络
Chat Paper
正在生成论文摘要