Joint Memory with Distance Recalculation for Unsupervised Person Re-Identification
2022 Prognostics and Health Management Conference (PHM-2022 London)(2022)
摘要
Most of the purely unsupervised person Re-Identification (Re-ID) methods use memory dictionary to calculate loss, and use clustering to create memory dictionary and generate pseudo labels. But most of these methods neglect the camera style of the training images, which will severely affect the results of clustering. This paper aims at this challenge, proposes the Joint Memory mechanism with Distance Recalculation. We make full use of and group the feature vectors according to the camera IDs of the training images, and use the clustering algorithm to generate pseudo labels and create memory dictionary inside the same camera. Further, we take advantage of this memory dictionary to recalculate the distances between the training images across all cameras and second time use clustering algorithm to generate pseudo labels and create a new memory dictionary. By jointly utilizing these two kinds of memory dictionary, we can train the network more robustly. Our method accomplishes excellent performance compared to most of state-of-the-art unsupervised Re-ID methods on many datasets, e.g., 91.7%, 84.2%, 59.1% rank-1 accuracy and 80.9%, 71.1%, 32.4% mAP on the Market, Duke and MSMT17 datasets. We achieve this performance when we train the network with a very small batch size, and it is very possible that we can reach a better, maybe surpass state-of-the-art, performance when using a bigger batch size.
更多查看译文
关键词
person re-identification,unsupervised learning,camera ID,memory dictionary
AI 理解论文
溯源树
样例
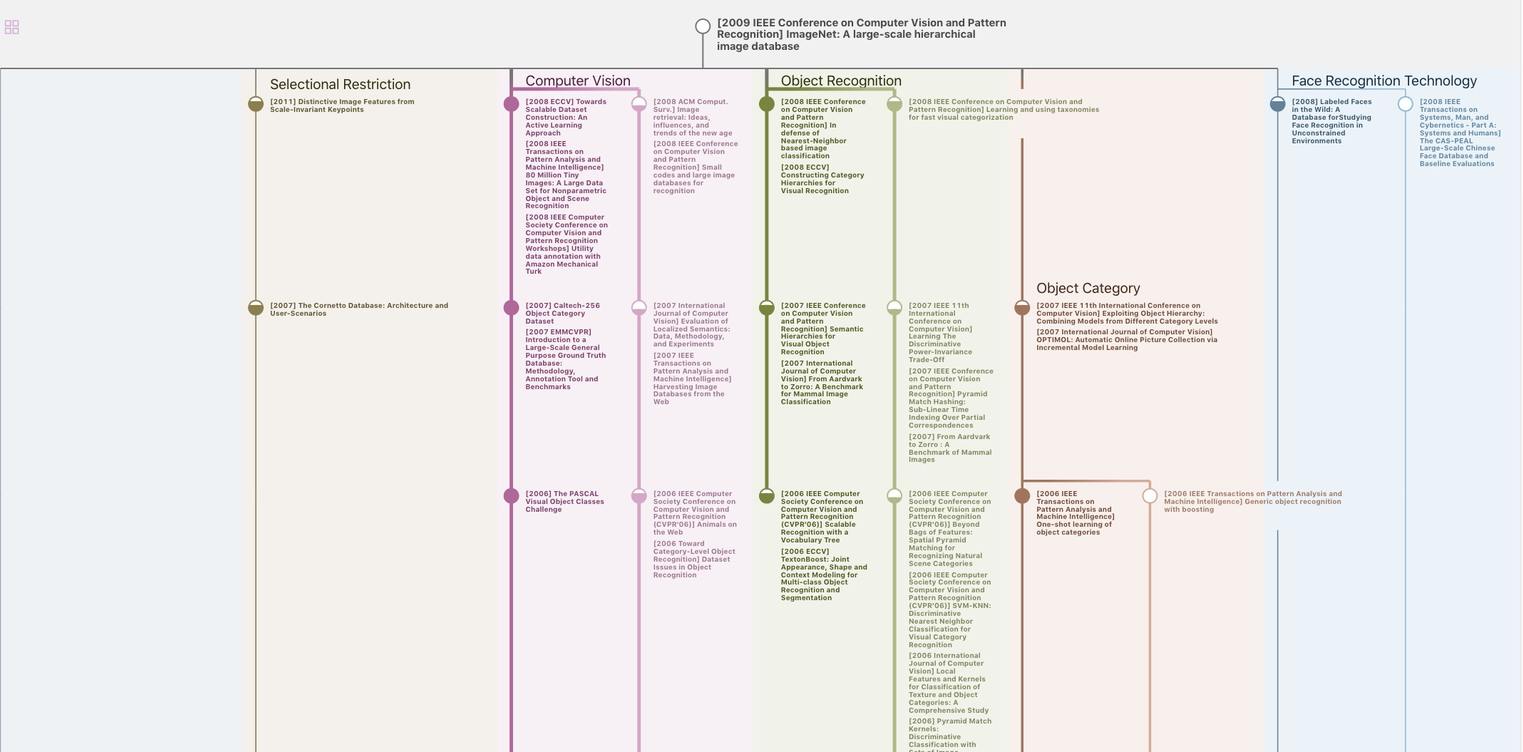
生成溯源树,研究论文发展脉络
Chat Paper
正在生成论文摘要