Short-term Power Load Forecasting based on Feature Fusion of Parallel LSTM-CNN
2022 IEEE 4th International Conference on Power, Intelligent Computing and Systems (ICPICS)(2022)
摘要
Accurate power load forecasting is beneficial to grid scheduling and operation. In order to fully exploit the potential relationship between load and feature. This research suggests a short-term power load forecasting strategy based on feature fusion to increase prediction accuracy, which combines parallel LSTM and CNN. The model first determines the optimal features of the model input through the random forest method and mutual information method, then classifies the features, and inputs the classified categories into the parallel LSTM modules respectively, and then uses the CNN network to output the neural network of different LSTM modules at the same time. The elements are convolved to extract the features of each moment, finally get the prediction result through the fully connected layer. The results of two short-term power load forecasting cases show that the proposed method's prediction effect outclasses existing methods. with model evaluation parameter MAPE of 1.367% and 0.974% respectively.
更多查看译文
关键词
deep learning,LSTM,CNN,feature extraction,short-term load forecasting
AI 理解论文
溯源树
样例
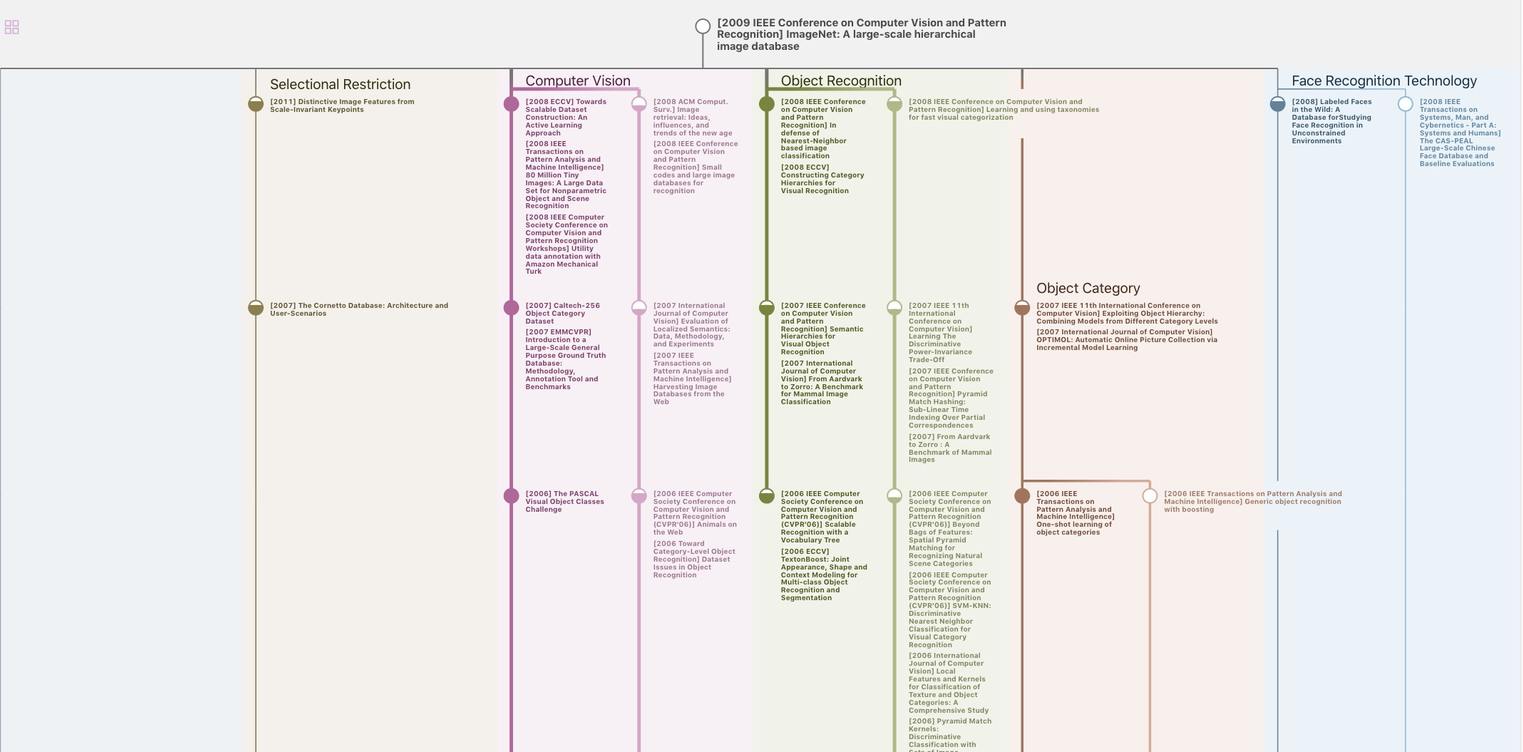
生成溯源树,研究论文发展脉络
Chat Paper
正在生成论文摘要