Large-scale mapping and systematic mutagenesis of human transcriptional effector domains
biorxiv(2022)
摘要
Human gene expression is regulated by over two thousand transcription factors and chromatin regulators[1][1],[2][2]. Effector domains within these proteins can activate or repress transcription. However, for many of these regulators we do not know what type of transcriptional effector domains they contain, their location in the protein, their activation and repression strengths, and the amino acids that are necessary for their functions. Here, we systematically measure the transcriptional effector activity of >100,000 protein fragments (each 80 amino acids long) tiling across most chromatin regulators and transcription factors in human cells (2,047 proteins). By testing the effect they have when recruited at reporter genes, we annotate 307 new activation domains and 592 new repression domains, a ∼5-fold increase over the number of previously annotated effectors[3][3],[4][4]. Complementary rational mutagenesis and deletion scans across all the effector domains reveal aromatic and/or leucine residues interspersed with acidic, proline, serine, and/or glutamine residues are necessary for activation domain activity. Additionally, the majority of repression domain sequences contain either sites for SUMOylation, short interaction motifs for recruiting co-repressors, or are structured binding domains for recruiting other repressive proteins. Surprisingly, we discover bifunctional domains that can both activate and repress and can dynamically split a cell population into high- and low-expression subpopulations. Our systematic annotation and characterization of transcriptional effector domains provides a rich resource for understanding the function of human transcription factors and chromatin regulators, engineering compact tools for controlling gene expression, and refining predictive computational models of effector domain function.
### Competing Interest Statement
Stanford has filed a provisional patent related to this work.
[1]: #ref-1
[2]: #ref-2
[3]: #ref-3
[4]: #ref-4
更多查看译文
AI 理解论文
溯源树
样例
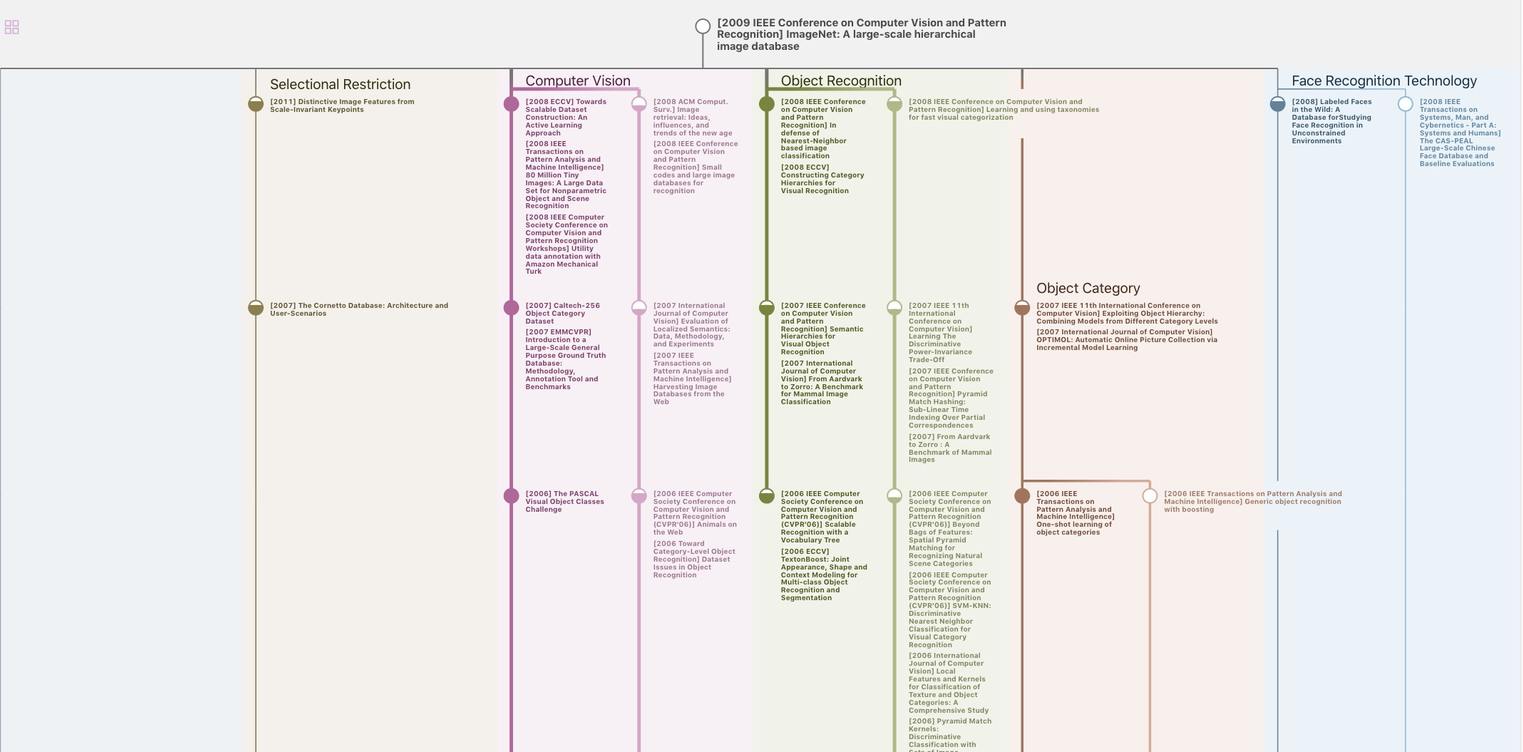
生成溯源树,研究论文发展脉络
Chat Paper
正在生成论文摘要