Best Image: Deep Learning for Saliency Estimation Using Expert Rankings
SPWLA 63rd Annual Symposium Transactions(2022)
摘要
When analyzing borehole image data, more is generally better. More sensors, higher resolution and multiple post-processing filters are typical strategies for ensuring that no important reservoir information is being missed. Handling large amounts of data can however be a hurdle. Storing the information at the time of measurement or sending large size data from downhole to the edge rig and then to the town geologist becomes a challenge. Analysis is another issue: How do you decide which channel to look at to extract the best information for reservoir characterization? In this work we propose a saliency score network, that given an interval of a borehole image assigns a score predicting how “interesting” a domain expert would find said interval. Having access to such scores brings several advantages and possibilities. Plotting scores versus depths provides a quick at-a-glance overview of a borehole image, for quality control, and for identifying and interpreting intervals that store the important information. The scores can also be used in a more direct way as a filter. Sections of a well containing no relevant information can be discarded at the point of collection to minimize data storage, and if using multiple sensors only the best sensor reading for each depth can be stored and sent to the interpreter. To create this scoring network two main problems had to be overcome: how to capture the preferences of the experts, and how to train a neural network to replicate these preferences. To capture the preferences of the experts we developed a labelling tool that allowed them to create a ranking from least interesting to most interesting, of more than 2000 images showing sections from different wells and depths. The expert made this ranking by comparing pairs of images and indicating which one was more interesting, or marking them as equal. A convolutional neural network, designed to capture the features and textures of borehole images, was then trained to assign a score to each image, using a pairwise loss that forces higher ranked images to get higher scores. We show that this type of training, where the network focuses on the relative scores between images, rather than predicting absolute scores, performs better in predicting their relative order. The trained network was able to identify relevant sections of wells, and where there was a discrepancy in quality between the sensors, select the better ones in agreement with expert evaluation.
更多查看译文
关键词
saliency estimation,expert rankings,deep learning,image
AI 理解论文
溯源树
样例
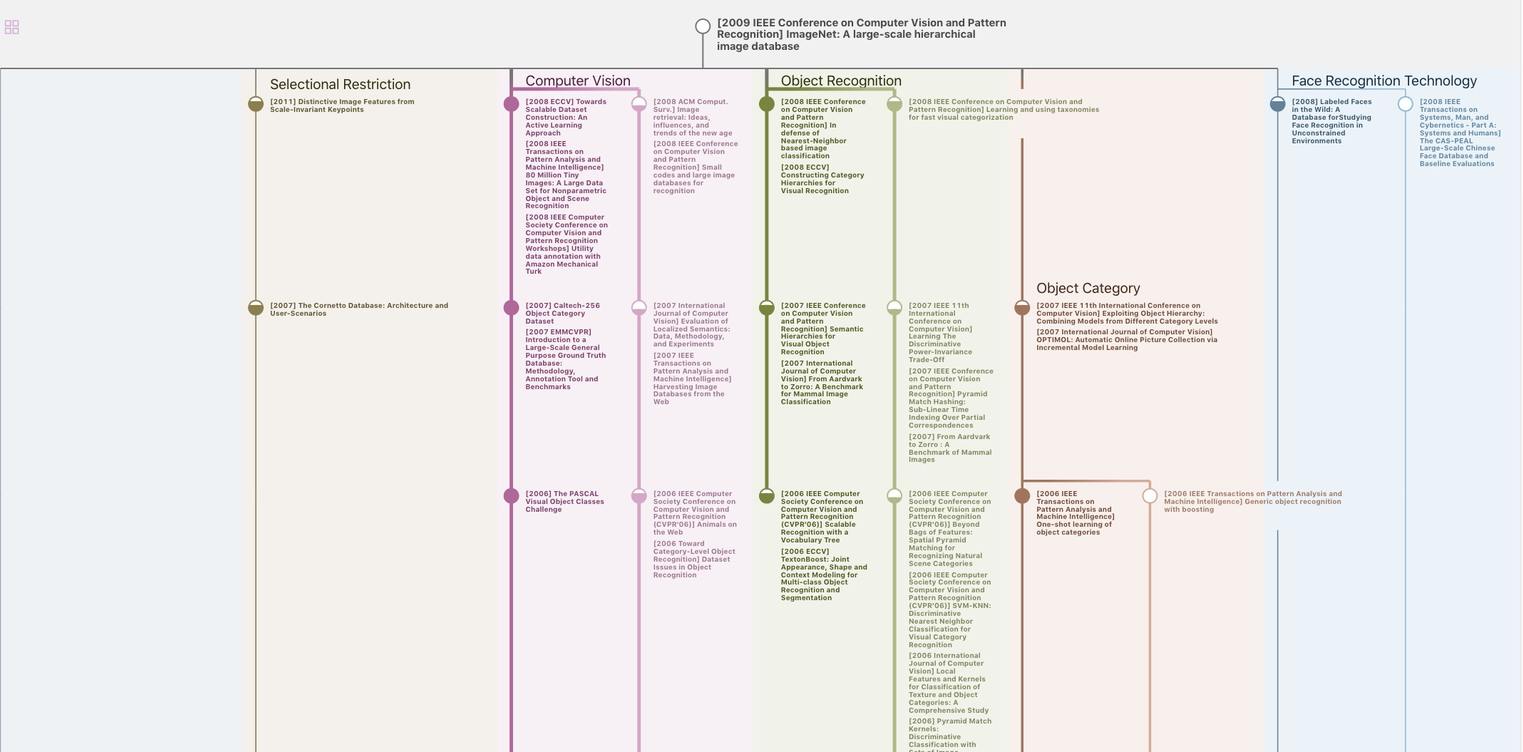
生成溯源树,研究论文发展脉络
Chat Paper
正在生成论文摘要