Attentional inhibition ability predicts neural representation during challenging auditory streaming
biorxiv(2022)
摘要
Focusing on a single source within a complex auditory scene is challenging. M/EEG-based auditory attention detection allows to detect which stream, within a set of multiple concurrent streams, an individual is attending to. The high inter-individual variability in the AAD performance is most often attributed to physiological factors and signal to noise ratio of neural data. Here we address the hypothesis that cognitive factors and in particular sustained attention, WM and attentional inhibition, may also partly explain the variability in AAD performance, because they support the cognitive processes required when listening to complex auditory scenes. Here, we chose a particularly challenging auditory scene, by presenting dichotically polyphonic classical piano excerpts lasting one minute each. Two different excerpts were presented simultaneously in each ear. Forty-one participants, with different degrees of musical expertise, listened to these complex auditory scenes focussing on one ear while we recorded the EEG. Participants also completed several tasks assessing executive functions. As expected, attended stimuli were better decoded than unattended stimuli. Importantly, attentional inhibition ability did explain around 10% of the reconstruction accuracy and around 8% of the classification accuracy. No other cognitive function was a significant predictor of reconstruction or of classification accuracies. No clear effect of musical expertise was found on reconstruction and classification performances. In conclusion, cognitive factors seem to impact the robustness of the auditory representation and hence the performance of neural based decoding approaches. Taking advantage of this relation could be useful to improve next-generation hearing aids.
### Competing Interest Statement
The authors have declared no competing interest.
更多查看译文
关键词
attentional inhibition ability,neural representation
AI 理解论文
溯源树
样例
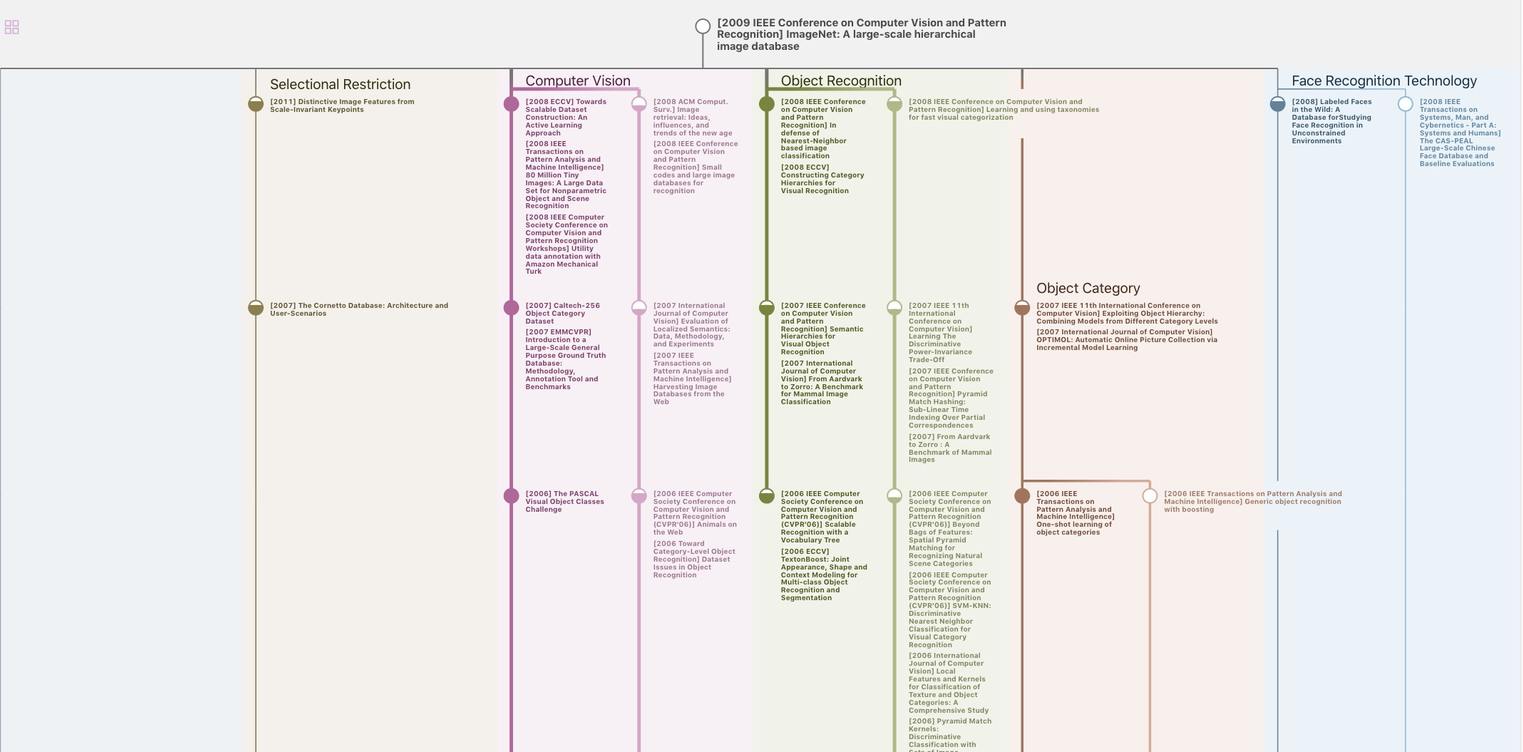
生成溯源树,研究论文发展脉络
Chat Paper
正在生成论文摘要