Seg2Link: an efficient and versatile solution for semi-automatic cell segmentation in 3D image stacks
biorxiv(2023)
摘要
Recent advances in microscopy techniques, especially in electron microscopy, are transforming biomedical studies by acquiring large quantities of high-precision 3D cell image stacks. To examine cell morphology and connectivity in organs such as the brain, scientists need to conduct cell segmentation, which extracts individual cell regions of different shapes and sizes from a 3D image. This is challenging due to the indistinct images often encountered in real biomedical research: in many cases, automatic segmentation methods inevitably contain numerous mistakes in the segmentation results, even when using advanced deep learning methods. To analyze 3D cell images effectively, a semi-automated software solution is needed that combines powerful deep learning techniques with the ability to perform post-processing, generate accurate segmentations, and incorporate manual corrections. To address this gap, we developed Seg2Link, which takes deep learning predictions as inputs and use watershed 2D + cross-slice linking to generate more accurate automatic segmentations than previous methods. Additionally, it provides various manual correction tools essential for correcting mistakes in 3D segmentation results. Moreover, our software has been optimized for efficiently processing large 3D images in diverse organisms. Thus, Seg2Link offers an practical solution for scientists to study cell morphology and connectivity in 3D image stacks.
更多查看译文
关键词
cell,3d,semi-automatic
AI 理解论文
溯源树
样例
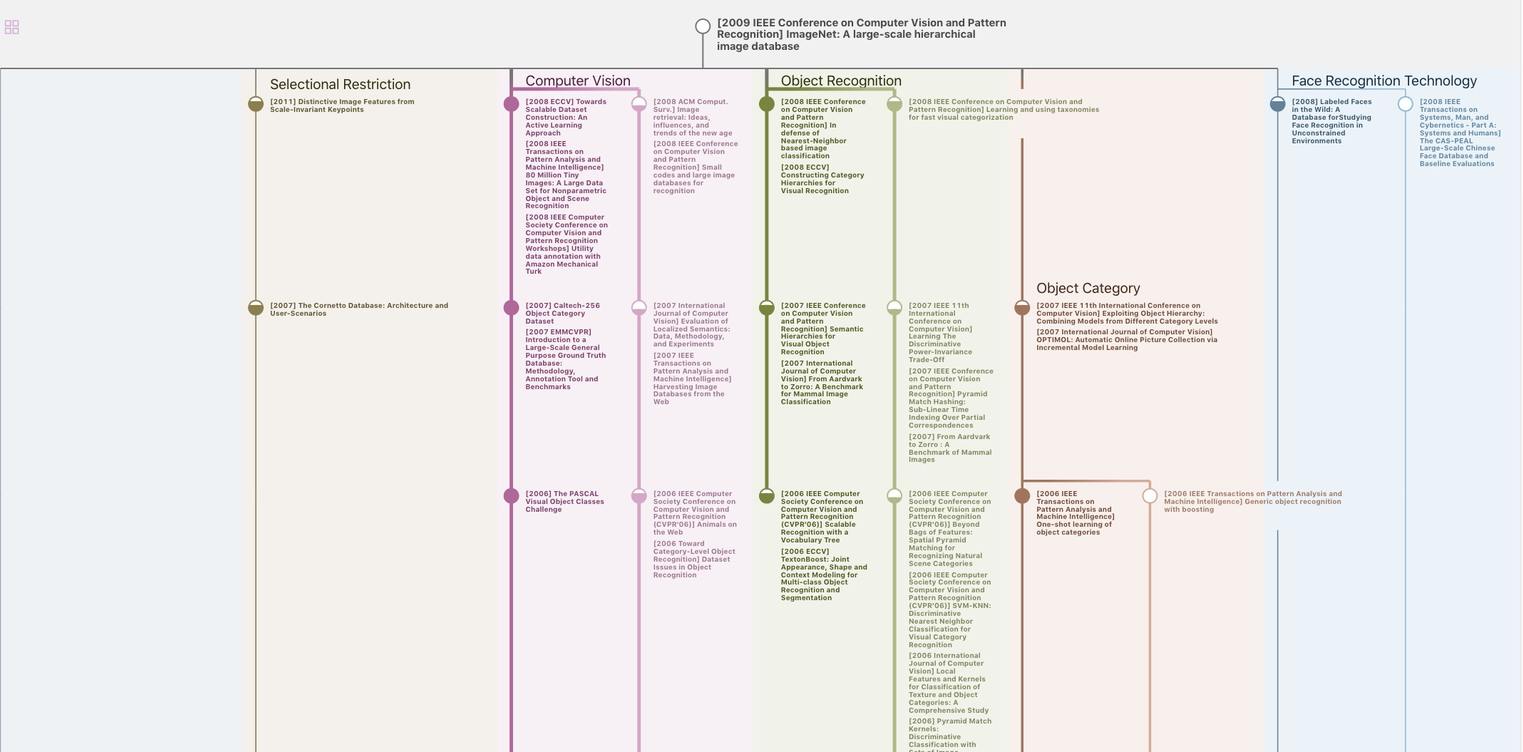
生成溯源树,研究论文发展脉络
Chat Paper
正在生成论文摘要