A complex Jensen–Shannon divergence in complex evidence theory with its application in multi-source information fusion
Engineering Applications of Artificial Intelligence(2022)
摘要
Multi-source information fusion has attracted considerable attention in the few past years and plays a great role in real applications. However, the uncertainty or conflict will make the fusion results unreasonable. Furthermore, the information may be collected in the form of complex number that cannot be processed by existing methods. In this article, to handle the above issues, the complex evidence theory (CET) is exploited. CET is the generalization of Dempster–Shafer evidence theory, where the mass function is modeled by complex number, called complex mass function (CMF). In order to deal with multi-source information fusion from the perspective of the complex plane, a new Complex Jensen–Shannon divergence (CJS divergence) is presented in this article. The proposed CJS divergence can effectively measure the conflict between two CMFs, and it satisfies the properties of boundedness, symmetry and nondegeneracy. In addition, for a better combination result, we have adjusted the complex Dempster’s rule of combination, which is called the reinforced complex evidence combination rule (RCECR). Then an algorithm for multi-source information fusion is proposed based on the CJS divergence and the RCECR. Some numerical examples and two applications in target identification and medical diagnosis illustrate the effectiveness of the new approach.
更多查看译文
关键词
Complex evidence theory,Complex mass functions,Complex Jensen–Shannon divergence,Multi-source information fusion
AI 理解论文
溯源树
样例
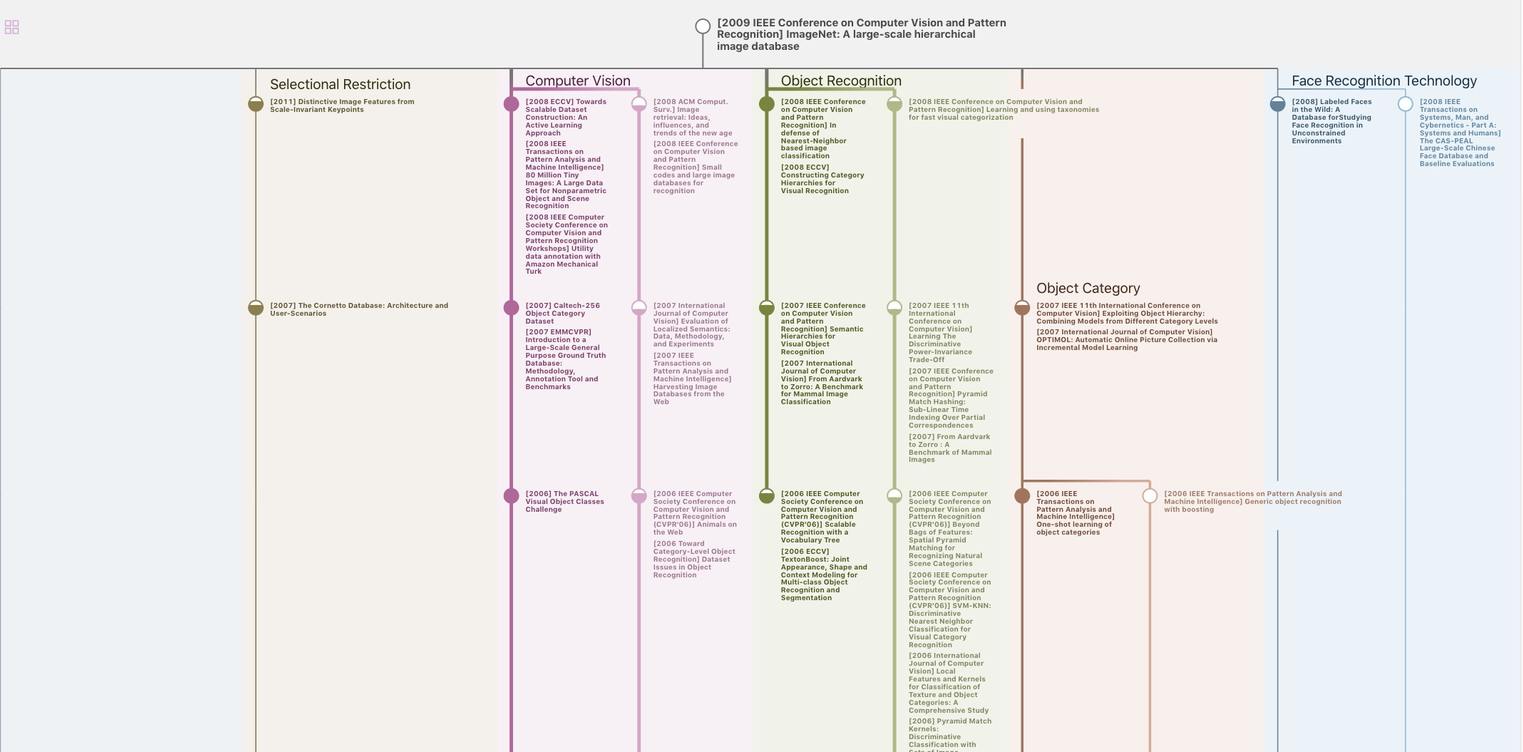
生成溯源树,研究论文发展脉络
Chat Paper
正在生成论文摘要