Derivation and Validation of a Machine Learning Algorithm for Predicting Venous Thromboembolism in Injured Children
Journal of Pediatric Surgery(2023)
摘要
INTRODUCTION: Venous thromboembolism (VTE) causes significant morbidity in pediatric trauma patients. There is a lack of consensus for VTE prophylaxis guidelines. We applied machine learning algorithms to the Trauma Quality Improvement Program (TQIP) database to develop and validate a risk prediction model for VTE in injured children. METHODS: Patients 18 years or younger were identified from TQIP (2017 to 2019, n = 383,814). Those administered VTE prophylaxis within 24 hours and those missing the outcome (VTE) were removed, leaving 363,987. With factor selection, 14 predictors were identified: intubation, need for supplemental oxygen, spinal cord injury, pelvic fractures, major surgery (neurosurgery, thoracic, abdominal, vascular), age, transfusion requirement, intracranial pressure monitor or external ventricular drain placement, low Glasgow Coma Scale score, and central line placement. The final data set was split into training (n = 251,409) and testing (n = 123,755) subsets. Machine learning algorithms (lasso regression [LR], random forest [RF], and generalized boosted machine [GBM]) were trained, compared, and tested. RESULTS: Low-risk prediction: For the testing subset, all 3 models outperformed the baseline rate of VTE (0.13%) with a predicted rate of 0.008% to 0.02% (p < 2.2e-16). RF and GBM outperformed LR (p = 0.0016); 86% to 87% of patients were classified as low risk by the models. High-risk prediction: All 3 models outperformed baseline with a predicted rate of VTE ranging from 1.20% to 1.46% (p < 2.2e-16). The performance of the 3 models was not significantly different. CONCLUSION: We successfully developed a predictive model that differentiates low- and high-risk injured children for development of VTE with high discrimination and can guide prophylaxis use.
更多查看译文
关键词
Venous thromboembolism,Pediatric trauma,TQIP,Machine learning
AI 理解论文
溯源树
样例
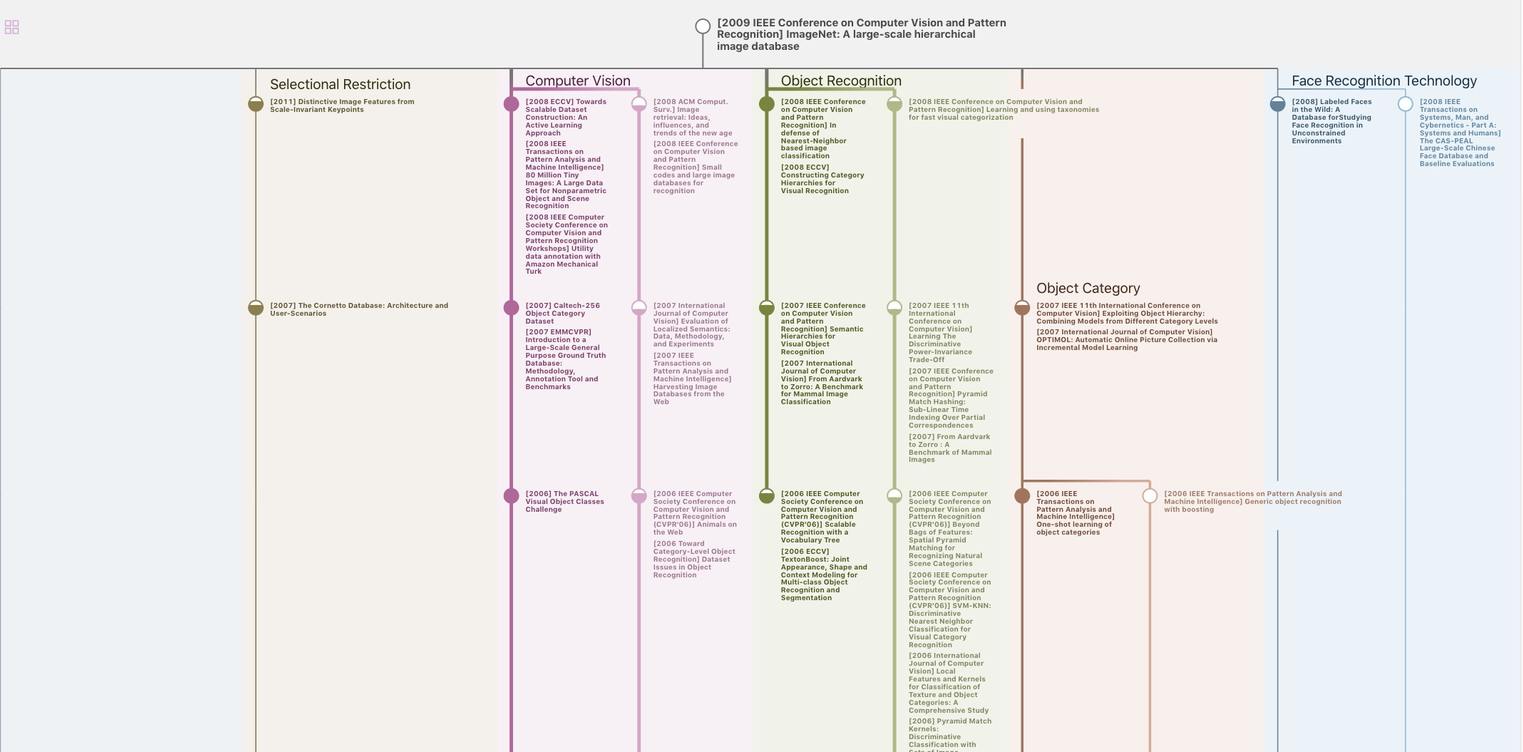
生成溯源树,研究论文发展脉络
Chat Paper
正在生成论文摘要