Effect of Trauma Mechanism of Injury on Post-Traumatic Stress Disorder and Depression Screening
Journal of the American College of Surgeons(2022)
摘要
INTRODUCTION: Few data exist guiding surgeons on the management of trauma-induced mental health issues. We hypothesize that the mechanism of injury (MoI) affects posttraumatic stress disorder (PTSD) and depression in trauma patients. METHODS: This is a retrospective cohort study of adult Level I trauma center patients from March 2018 to June 2021 completing a 3-month follow-up screening for PTSD with PCL-5 and depression with PHQ-9 scoring. Demographics and hospitalization details were culled from electronic medical records. MoI was categorized as blunt: low energy, blunt: high energy, and penetrating type by CDC Field Triage Guidelines. Baseline characteristics were compared. Scores were dichotomized before generating logistic and ordinal regression models. RESULTS: A total of 1,148 trauma patients underwent follow-up screening for PTSD and depression. MoI included 67.6% blunt: high energy, 23.2% blunt: low energy, and 9.2% penetrating trauma. Penetrating patients were more likely to screen PTSD positive (odds ratio [OR] 1.72, 95% CI 0.98 to 2.93) and blunt: low energy patients were less likely (OR 0.49, 95% CI 0.28 to 0.84). MoI did not significantly influence depression screening. Patients with previous depression history were more likely to screen positive for PTSD (OR 2.80, 95% CI 1.70 to 4.61) and experience higher depression scores (OR 2.36, 95% CI 1.61 to 3.47; Figure).Figure.: ISS, injury severity score; PTSD, posttraumatic stress disorder.CONCLUSION: Mechanism of injury influences psychiatric sequelae in trauma patients. Compared wth blunt mechanisms, penetrating trauma patients were more likely to screen positive for PTSD. Blunt: low energy provided a protective effect. Routine PTSD screening, especially after penetrating trauma, may identify patients requiring further investigation and intervention.
更多查看译文
AI 理解论文
溯源树
样例
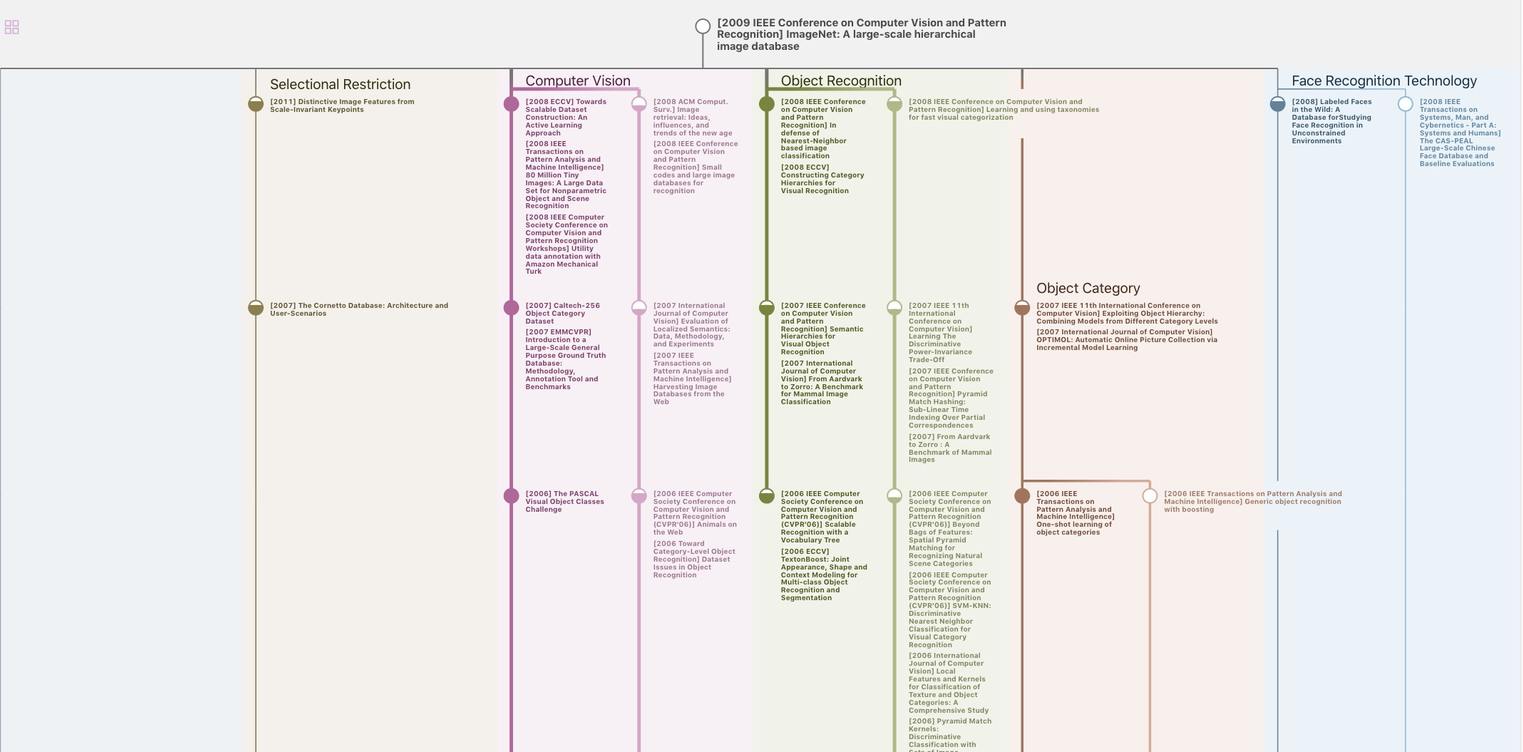
生成溯源树,研究论文发展脉络
Chat Paper
正在生成论文摘要