Convolutional neural networks for ultrasound corrosion profile time series regression
NDT & E International(2022)
摘要
A customised convolutional neural network (CNN) architecture is proposed in this paper to make estimations about the thickness values (minimum and mean) of corroded profiles from an ultrasonic time-series measurement (A-scan). The CNN architecture is determined after a hyper-parameter optimisation which leads to the best performing network. The model is trained using synthetic data and tested on both synthetic and experimental datasets. A comparison is made with (1) a state-of-the-art network for time series analysis and (2) conventional thickness estimation techniques such as peak envelope and threshold crossing. The proposed network provides an accurate estimation of the thickness values and outperforms the conventional techniques in both synthetic and experimental datasets. When compared to a conventional threshold-crossing technique for minimum thickness prediction, the proposed network is more consistent and less sensitive to changes in the threshold.
更多查看译文
关键词
Non-destructive evaluation,Deep learning,Ultrasound,Convolutional neural networks,Corrosion,Wall thickness
AI 理解论文
溯源树
样例
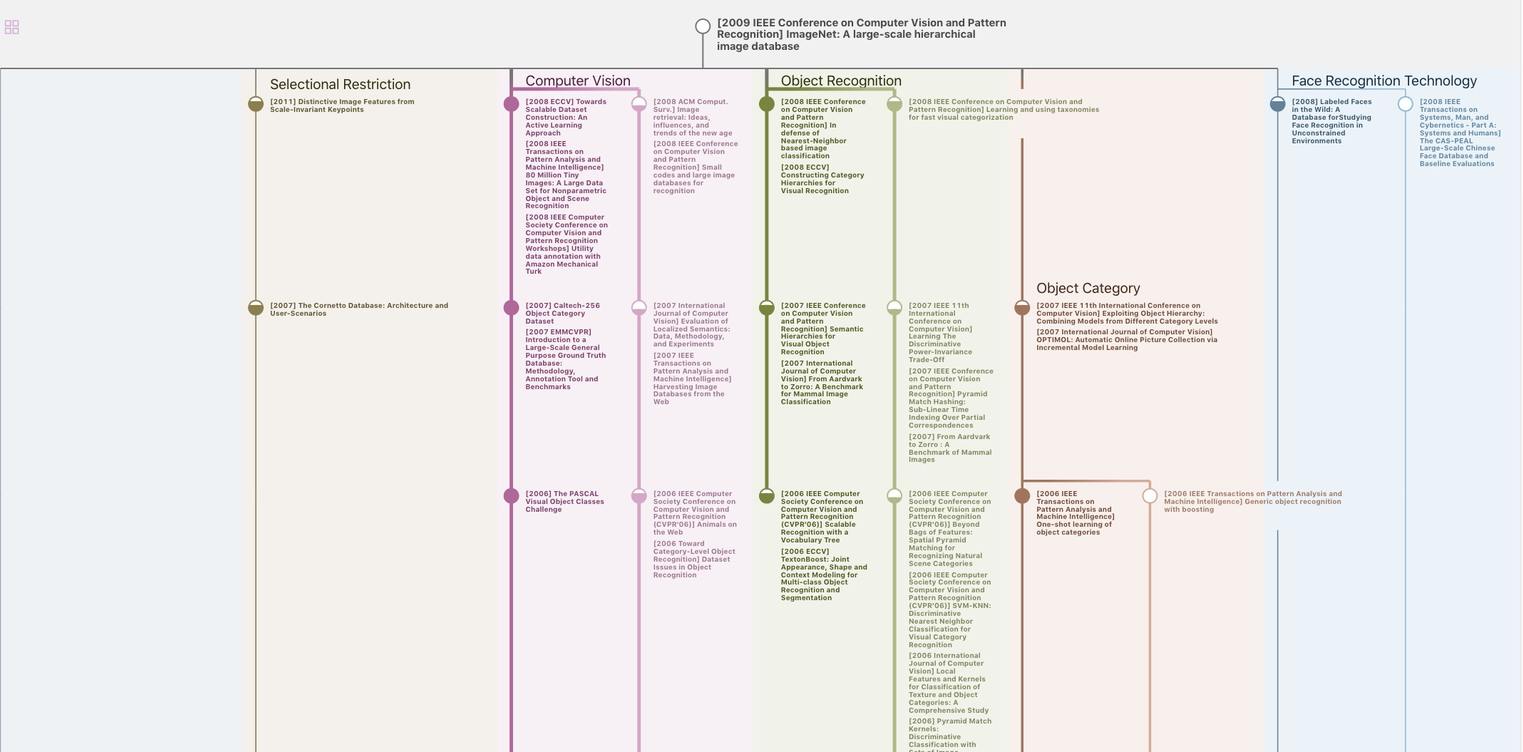
生成溯源树,研究论文发展脉络
Chat Paper
正在生成论文摘要