Cloud White: Detecting and Estimating QoS Degradation of Latency-Critical Workloads in the Public Cloud
Future Generation Computer Systems(2023)
摘要
The increasing popularity of cloud computing has forced cloud providers to build economies of scale to meet the growing demand. Nowadays, data-centers include thousands of physical machines, each hosting many virtual machines (VMs), which share the main system resources, causing interference that can significantly impact on performance. Frequently, these data-centers run latency-critical workloads, whose performance is determined by tail latency, which is very sensitive to the interference of co-running workloads. To prevent QoS violations, cloud providers adopt overprovisioning strategies but they reduce the server utilization and increase the costs. A mechanism that accurately estimates performance degradation dynamically in a production system would allow cloud providers to improve the servers’ utilization. In this work we propose Cloud White, an approach that is able to detect the inter-VM interference in scenarios with multiple co-located latency-critical VMs and estimate the performance degradation using multi-variable regression models. Unlike previous proposals, Cloud White is built taking into account the limitations of a public cloud production system. Experimental results show that Cloud White is able to estimate performance degradation with a small overall prediction error of 5%.
更多查看译文
关键词
Cloud computing,Public cloud,Virtualization,Interference,Performance estimation,QoS,Tail latency,Latency-critical workloads
AI 理解论文
溯源树
样例
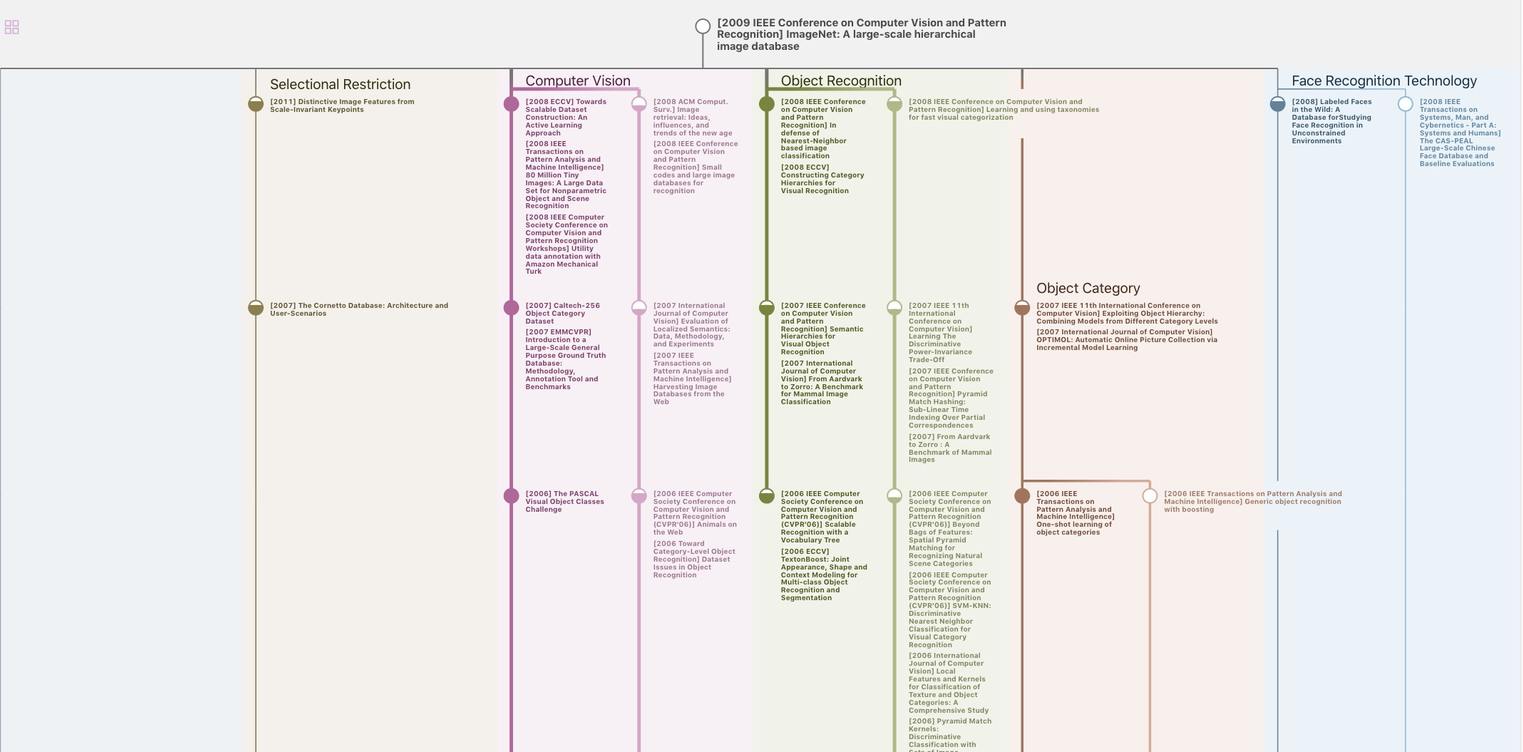
生成溯源树,研究论文发展脉络
Chat Paper
正在生成论文摘要