AWGAN: Unsupervised Spectrum Anomaly Detection with Wasserstein Generative Adversarial Network along with Random Reverse Mapping
2021 17th International Conference on Mobility, Sensing and Networking (MSN)(2021)
摘要
Automatic wireless spectrum anomaly detection is vital to intelligent management of electromagnetic spectrum, which aims to detect various jamming and anomalous working states, especially intentional jamming. The intentional jamming has evolved in a variety of ways, but the existing spectrum anomaly detection efforts give little consideration to the diverse intentional jamming. Here, we firstly generate a rich dataset consisting of five types of normal signals and four types of intentional jamming. In order to effectively detect anomalies, we propose AWGAN, a novel anomaly detection method based on Wasserstein generative adversarial network. AWGAN can not only learn the distribution of normal time-frequency waterfall images in a latent space, but also remember the detailed features of normal images, and generate same images as the normal images by adversarial training. To detect anomalies, we propose a random reverse mapping (RRM) method based on backpropagation, to map a new time-frequency waterfall image into the latent space, so as to find the vector closest to the distribution of the new image in the latent space. We also define a scoring criterion to score images indicating their fit into the learned distribution. The experimental results show that the comprehensive detection ability of our method is superior to other methods for detecting the four types of anomalies.
更多查看译文
关键词
anomaly spectrum detection,generative adversarial network,random reverse mapping
AI 理解论文
溯源树
样例
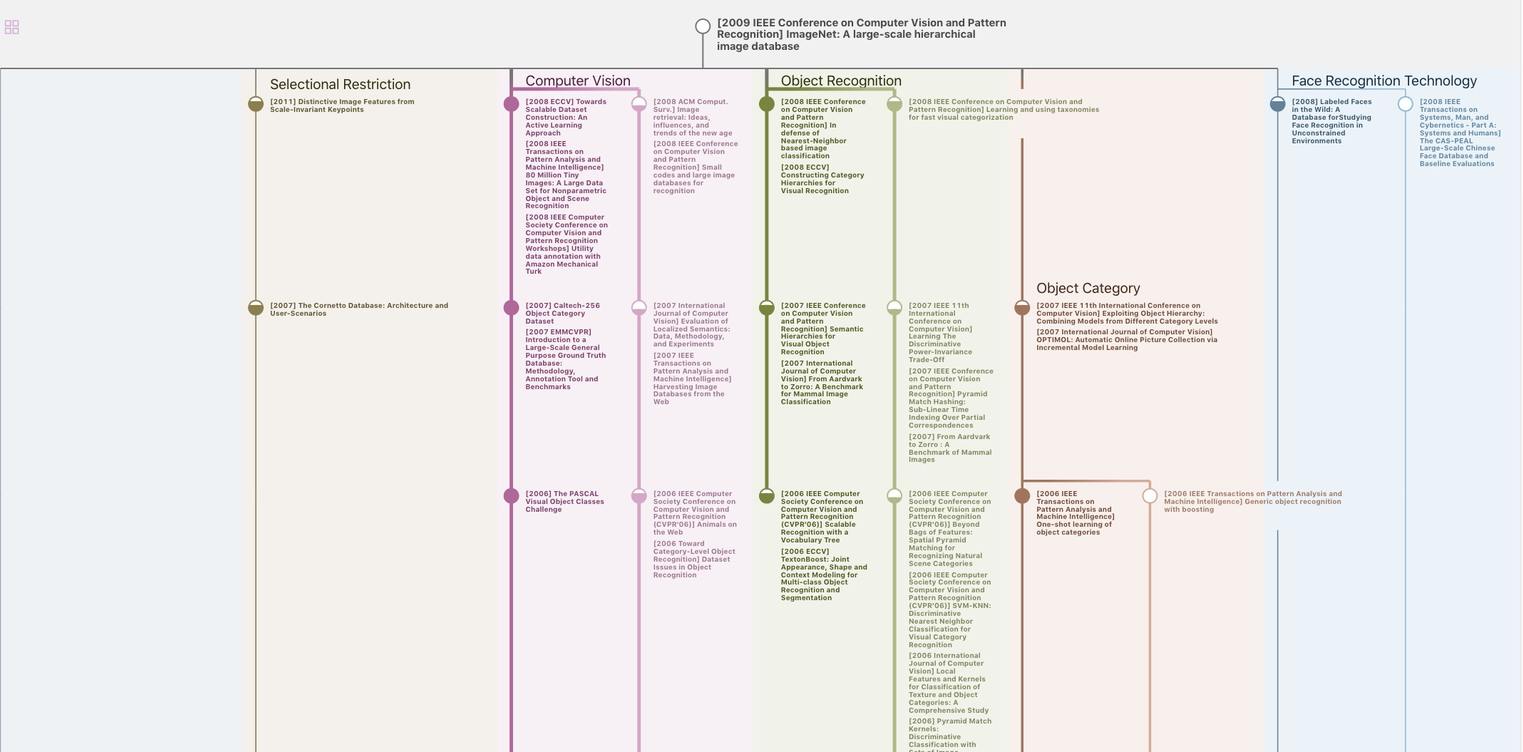
生成溯源树,研究论文发展脉络
Chat Paper
正在生成论文摘要