A Contextual and Content Features-Based Device Behavioral Fingerprinting Method in Smart Grid
2021 IEEE 23rd Int Conf on High Performance Computing & Communications; 7th Int Conf on Data Science & Systems; 19th Int Conf on Smart City; 7th Int Conf on Dependability in Sensor, Cloud & Big Data Systems & Application (HPCC/DSS/SmartCity/DependSys)(2021)
摘要
Smart grid system faces various novel attacks that are caused by increasing connection to other networks. Adversaries can easily fabricate and modify packets between devices and the SCADA workstation. Thus, it is essential to monitor the behavior features of the devices. However, the conventional device behavioral fingerprint methods, such as Rule-Based, Statistical-Based, and Knowledge-Based, overly rely on feature selection, which limits its application range. This paper proposes a novel device behavioral fingerprinting method that automatically extracts high dimension features from data packets using a deep neural network. The fingerprint is a vector composed of certain contextual and content features. The content features are extracted from the grayscale images that are reshaped from the packet payload. Besides, the contextual features are extracted from the packet header sequence matrix that consists of several packets header in a sliding window w. Finally, an unencrypted public dataset collected from the Electric Power and Intelligent Control(EPIC) testbed is used to verify the classification performance. Our experimental results indicate that the optimal sliding window size and the image size are 15 and 10. Besides, compared with the method using only one kind of feature (contextual or content feature), the proposed method can identify devices more efficiently and effectively, and it can be a unique identifier to describe the network behavior of devices in the smart grid.
更多查看译文
关键词
Contextual feature,content feature,device behavioral fingerprint,smart grid
AI 理解论文
溯源树
样例
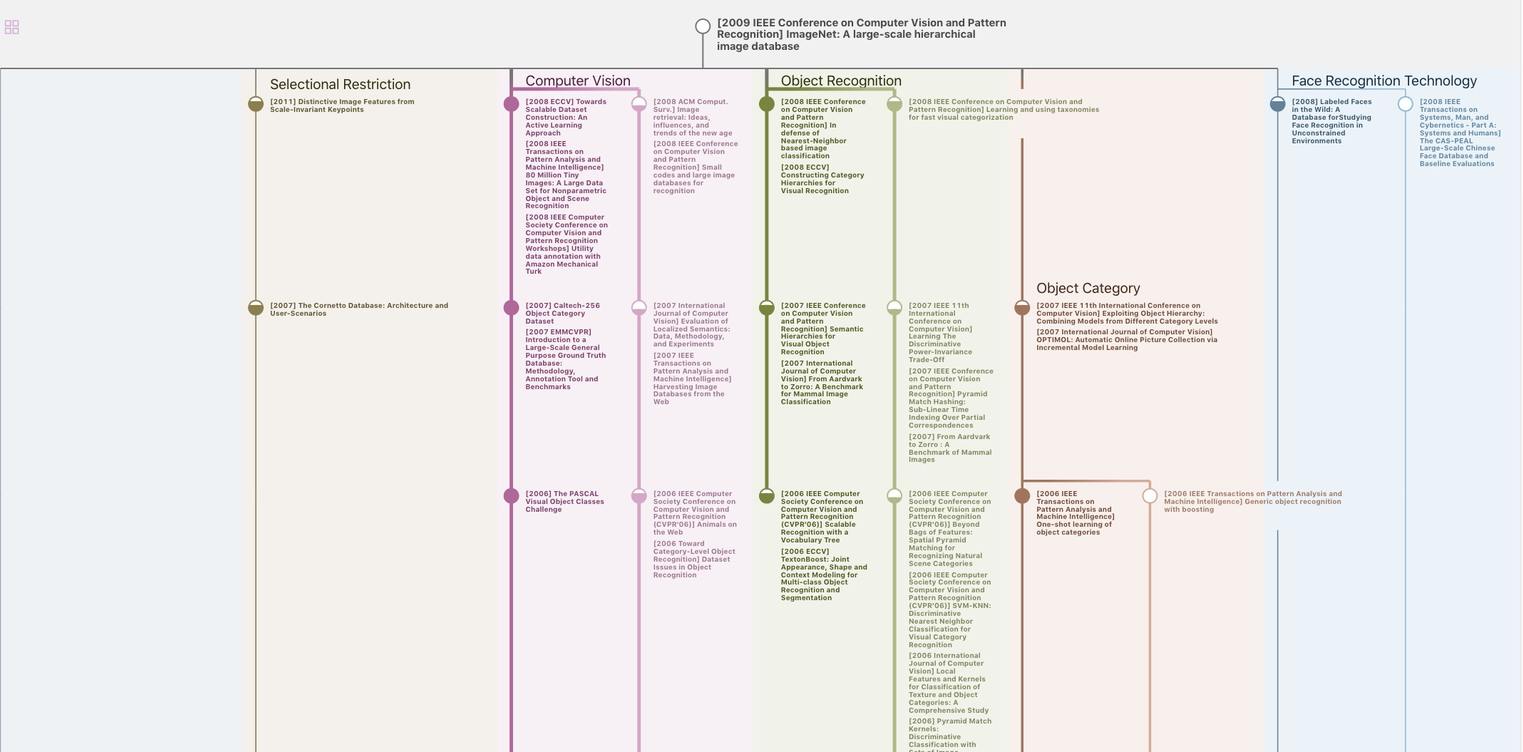
生成溯源树,研究论文发展脉络
Chat Paper
正在生成论文摘要