An Accurate and Interpretable Lifetime Prediction Method for Batteries using Extreme Gradient Boosting Tree and TreeExplainer.
IEEE International Conference on High Performance Computing and Communications(2021)
摘要
Lithium-ion batteries have been widely used in many fields such as electric vehicles and smart grid. Accurately predicting its lifetime is crucial for ensuring safety and accelerating battery technological development. This study aims to develop an interpretable battery lifetime prediction method based on a machine learning model by explaining the features used in the model. Firstly, the battery charge-discharge cycle data is analyzed, and five key features related to lifetime are extracted from the discharge curve of the first 100 cycles. Then, extreme gradient boosting tree (XGBoost) is built to learn the relationship between the features and the lifetime, and its optimal parameters are obtained through grid search and five-fold cross-validation. TreeExplainer is used to calculate shapley value based on game theory to quantitatively interpret and reveal the important features contributing to lifetime. Experimental results on the latest battery dataset demonstrate that XGBoost can effectively predict battery lifetime. At the same time, quantitative analysis and interpretation provide the reasons for the model decision, which improves the credibility of the prediction method and even helps to have a deeper understanding of the battery degradation mechanism.
更多查看译文
关键词
lithium-ion batteries,lifetime,interpretability,extreme gradient boosting tree,shapley value
AI 理解论文
溯源树
样例
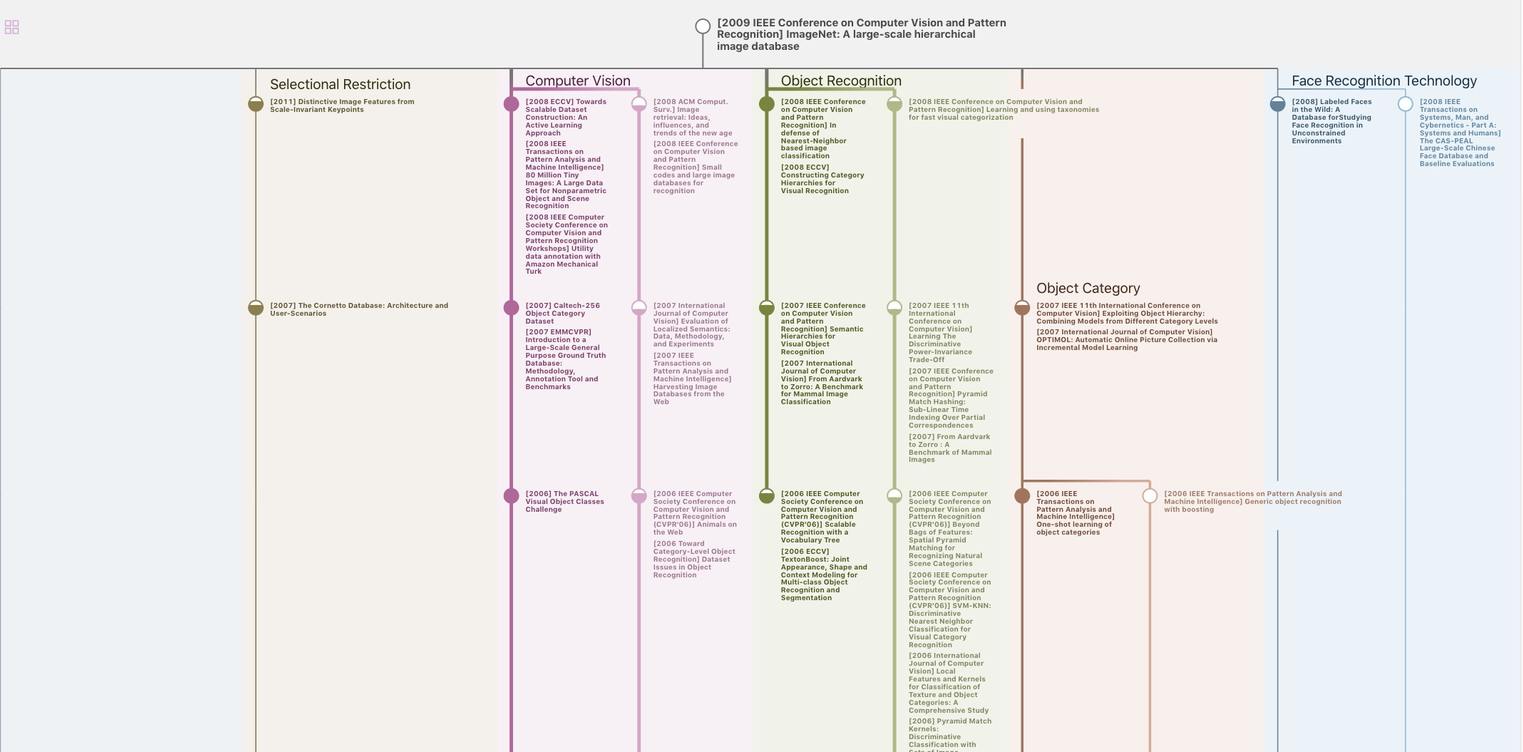
生成溯源树,研究论文发展脉络
Chat Paper
正在生成论文摘要