Smart City Traffic Intersection: Impact of Video Quality and Scene Complexity on Precision and Inference.
IEEE International Conference on High Performance Computing and Communications(2021)
摘要
Traffic intersections are prime locations for deployment of infrastructure sensors and edge computing nodes to realize the vision of a smart city. It is expected that the needs of a smart city, in regards to traffic and pedestrian traffic systems monitored by cameras/video, can be met by using state-of-the-art artificial-intelligence (AI) based object detectors and trackers. A critical component in designing an effective real-time object detection/tracking pipeline is the understanding of how object density, i.e., the number of objects in a scene, and image-resolution and frame rate influence the performance metrics. This study explores the accuracy and speed metrics with the goal of supporting pipelines that meet the precision and latency needs of a real-time environment. We examine the impact of varying image-resolution, frame rate and object-density on the object detection performance metrics. The experiments on the COSMOS testbed dataset show that varying the frame width from 416 pixels to 832 pixels, and cropping the images to a square resolution, result in the increase in average precision for all object classes. Decreasing the frame rate from 15 fps to 5 fps preserves more than 90% of the highest F1 score achieved for all object classes. The results inform the choice of video preprocessing stages, modifications to established AI-based object detection/tracking methods, and suggest optimal hyper-parameter values.
更多查看译文
关键词
Object Detection,Smart City,Video Resolution,Deep Learning Models
AI 理解论文
溯源树
样例
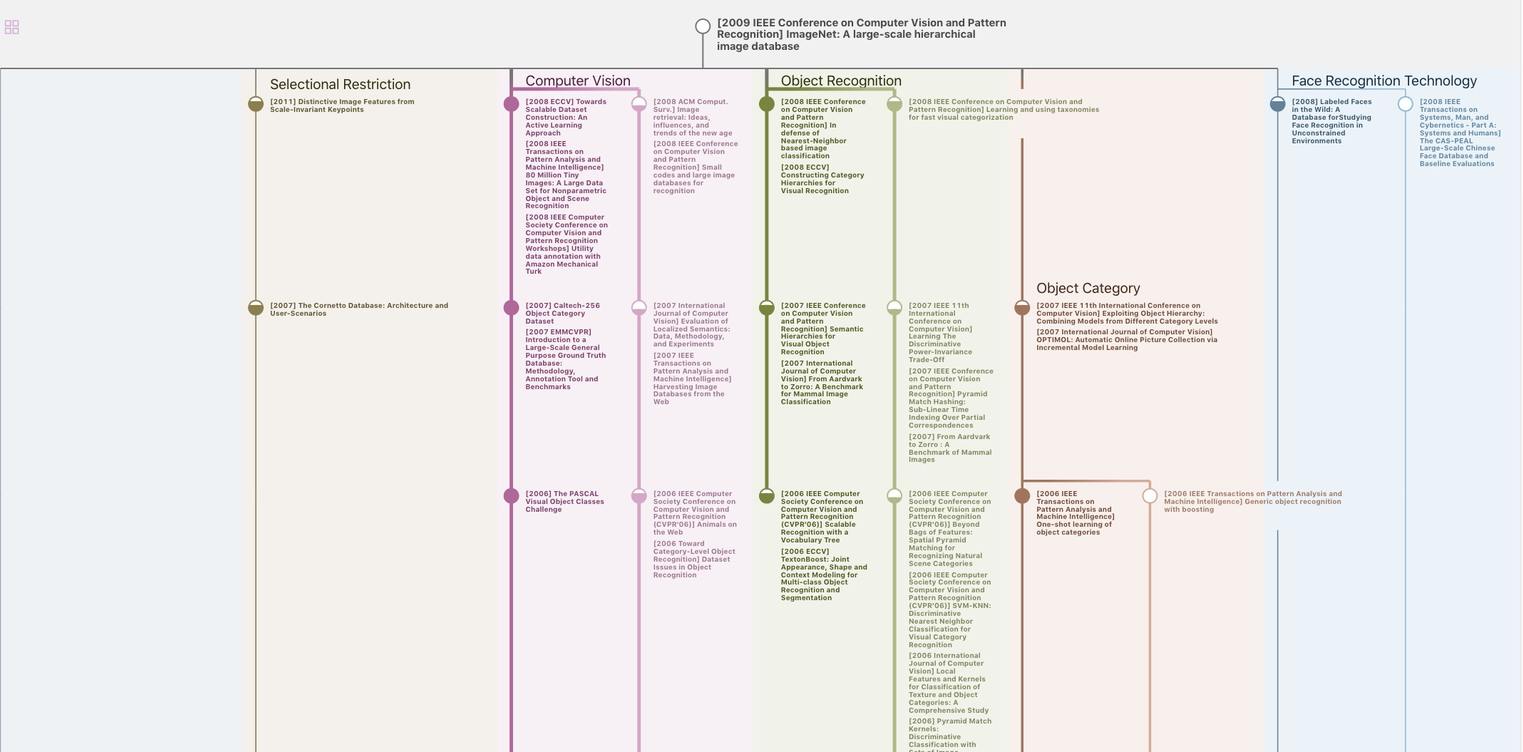
生成溯源树,研究论文发展脉络
Chat Paper
正在生成论文摘要