Aggregative feedback optimization for distributed cooperative robotics
IFAC-PapersOnLine(2022)
摘要
In this paper we propose Aggregative Tracking Feedback, i.e., a novel distributed feedback optimization law that steers network systems to a steady state, which is optimal according to an aggregative optimization problem. Aggregative optimization is a recently emerged distributed optimization framework in which the agents of a network minimize the sum of local objective functions. These functions depend both on local and aggregate decision variables (e.g., the barycenter). Motivated by this problem setup, we design a distributed feedback optimization law in which each agent reconstructs information not locally available while concurrently steering the network to an optimal steady state. We perform a system theoretical analysis based on a singular perturbation approach to show that Aggregative Tracking Feedback, in case of strongly convex objective functions, steers the network with a linear convergence rate to the problem minimum. Finally, we show some numerical simulations on a multi-robot surveillance scenario to validate the effectiveness of the proposed method.
更多查看译文
关键词
Distributed optimization,Decentralized Control,Large-Scale Systems,Robotics,multi-agent systems
AI 理解论文
溯源树
样例
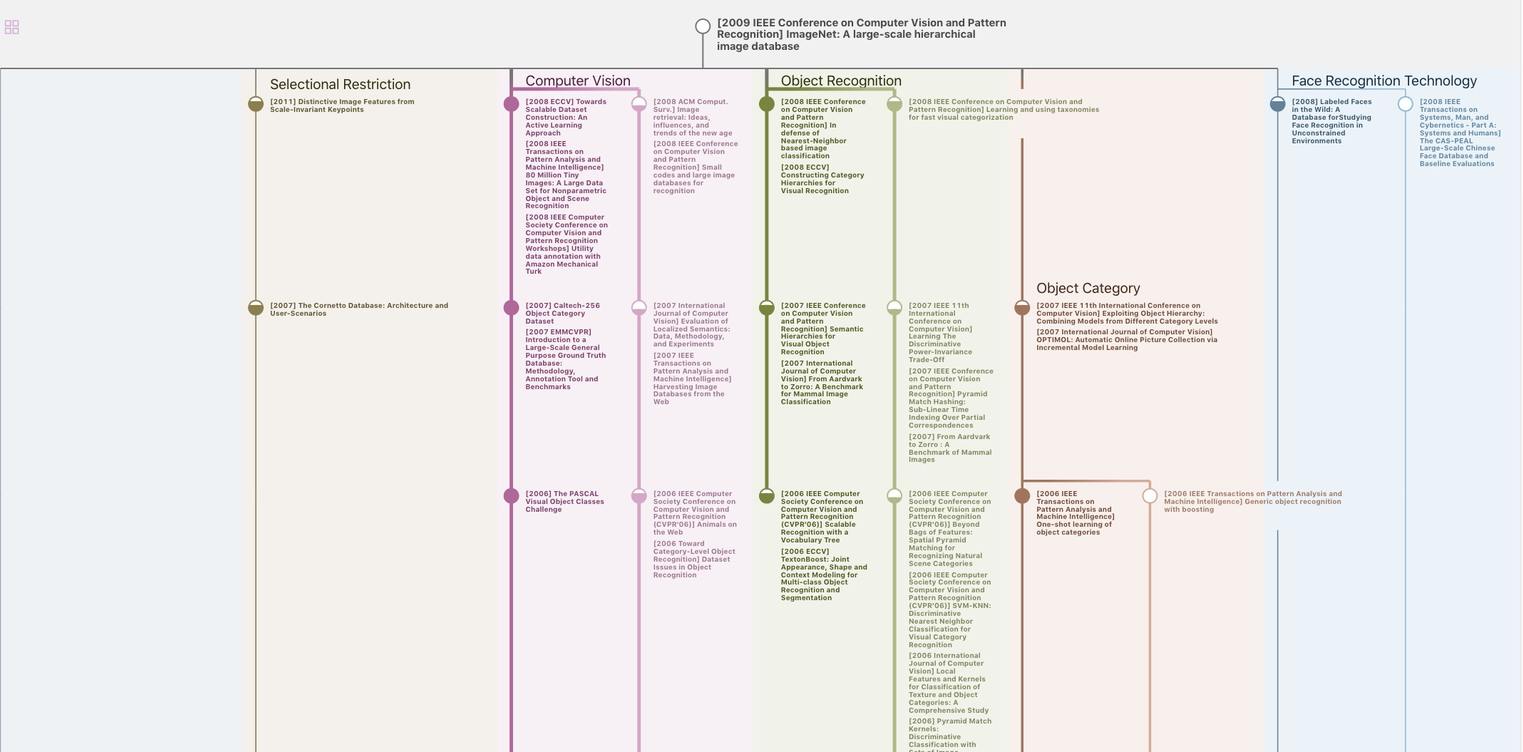
生成溯源树,研究论文发展脉络
Chat Paper
正在生成论文摘要