CAGSF: Optimization Algorithm for Network Representation Learning Based on the Community Structure
2022 5th International Conference on Pattern Recognition and Artificial Intelligence (PRAI)(2022)
摘要
Network representation learning is one of the research hotspots of complex network theory, which mainly solves the coupling problem of traditional complex network representations. Many classical network shallow representation learning algorithms have achieved good results in some downstream tasks. However, the node representation learned by these algorithms generally has the problem of noise because the structural information of the community is not considered. Therefore, this paper proposes a network representation learning optimization algorithm based on the structure of the community: CAGSF. Firstly, the classical community partition algorithm is used to obtain the community structure information of the network. And then we use this information to smooth the noise generated in the node representation process, so that the nodes in the same community can be represented as much as possible. Experimental results show that the algorithm improves the performance of the four classical network representation learning algorithms by up to 5% and 4.73% in the tasks of node classification and link prediction.
更多查看译文
关键词
network representation,coupling,community structure,noise,smoothing
AI 理解论文
溯源树
样例
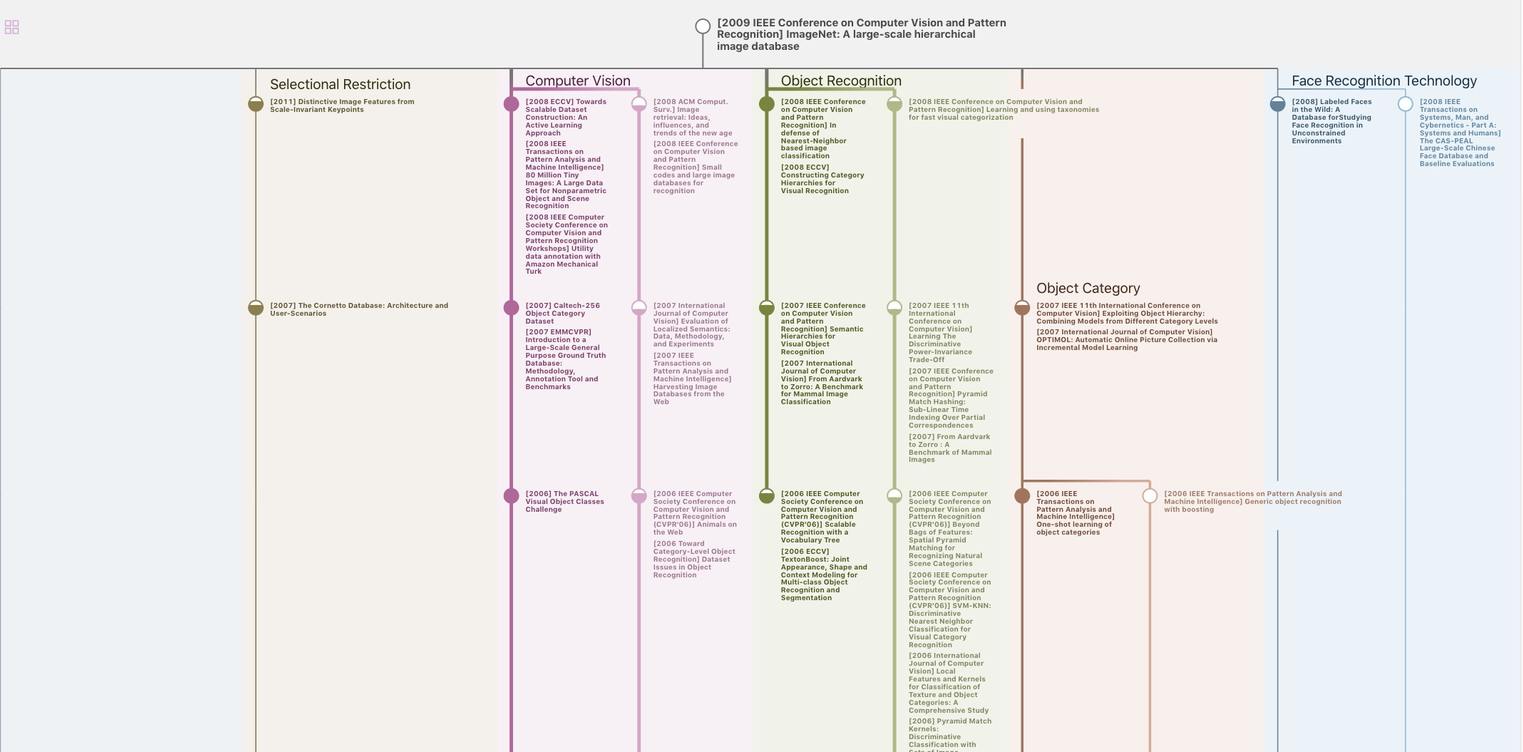
生成溯源树,研究论文发展脉络
Chat Paper
正在生成论文摘要