Investigations of Object Detectors with Deep Learning Methods: A Review
2022 5th International Conference on Pattern Recognition and Artificial Intelligence (PRAI)(2022)
摘要
Over the past ten years, deep learning methods has achieved great progress in the field of computer vision (CV), especially in object detection. In contrast with traditional detection methods, deep learning methods significantly outperform those in real-time performance and accuracy without any complicated hand-crafted process of feature extraction. The powerful ability of convolutional neural networks (CNNs) to extract features was realized as image classification task made breakthroughs by employing it. Therefore, many researchers attempt to apply CNNs which can learn high-level semantic features to object detection, producing some representative models like classical one-stage detectors and two-stage detectors. Besides, in recent years, Transformer which shows extraordinary talents in the field of natural language processing (NLP) has also been utilized in object detection models. A kind of novel detectors has been proposed based on transformer encoder-decoder architecture without usage of anchor generation and non-maximum suppression (NMS) postprocessing, which starts a new detection mode—set prediction, and gains great performance. In this paper, we summarize various detectors from different detection modes. To begin with, our review shows architecture of classical two-stage detectors and one-stage detectors. Then, transformer-based detectors are introduced in detail. Experimental evaluation is also provided to compare performance from various detectors. Finally, we raise a conclusion and future prospects to serve as a guideline for future work.
更多查看译文
关键词
computer vision,deep learning,object detection,CNN,transformer
AI 理解论文
溯源树
样例
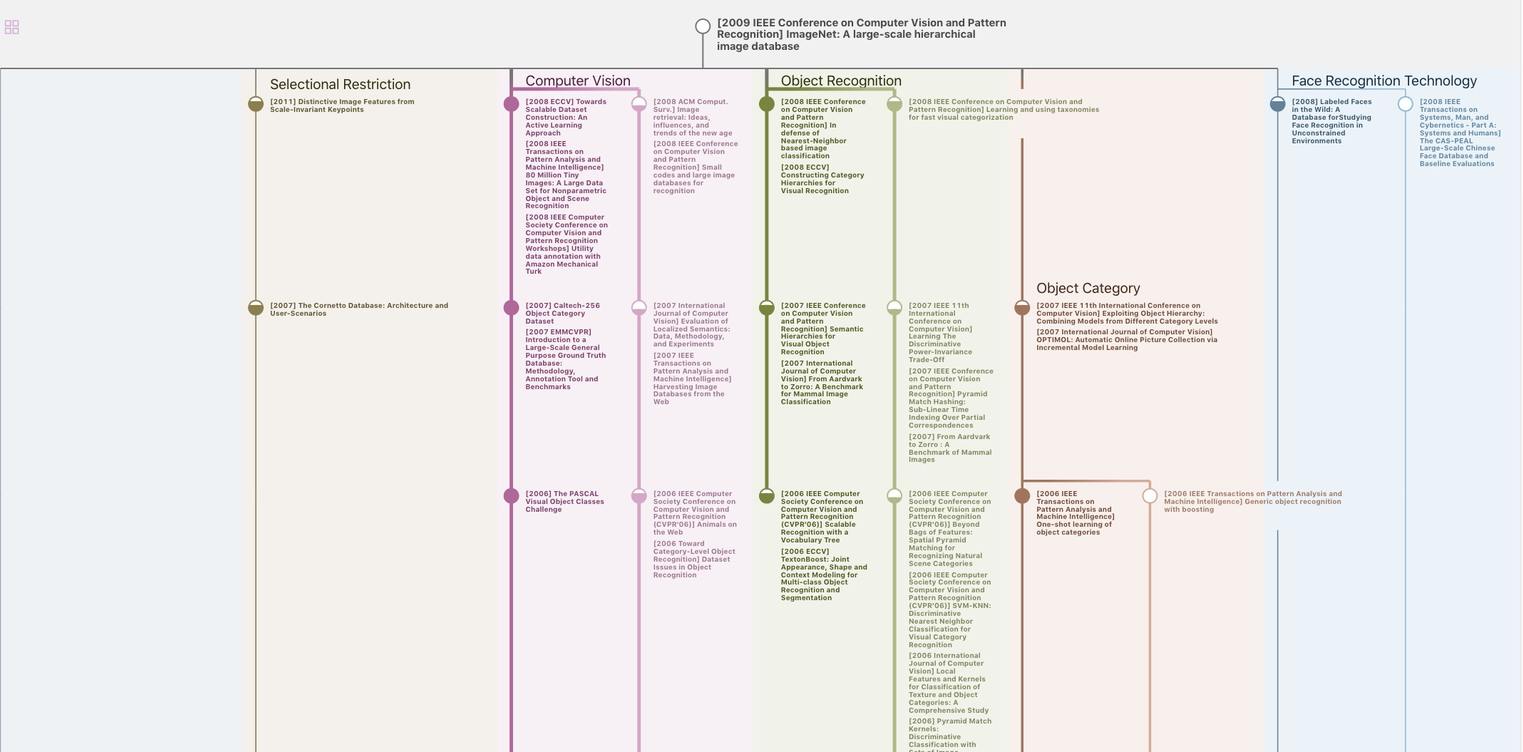
生成溯源树,研究论文发展脉络
Chat Paper
正在生成论文摘要