Multi-object Tracking with Noisy Labels
2022 5th International Conference on Pattern Recognition and Artificial Intelligence (PRAI)(2022)
摘要
Multi-Object Tracking (MOT) has achieved tremendous progress in the past few years. However, almost all existing joint detection and embedding (JDE) based methods have overlooked the ground-truth annotation qualities affect by occlusion. In this paper, we argue that MOT annotations are often effect by noisy labels. In addition, these noisy labels are actually harmful for the training process of JDE based method. To address this challenge, we propose a novel Multi-Object Tracking method for Noisy Labels, named MOTNL. MOTNL can not only diagnose noisy labels but also choose more high-quality clean labels for model training. Specifically, we first analyze the properties of occluded samples in multi-object tracking video datasets. And design a simple but effective selection mechanism in the Re-ID branch of our MOTNL. Extensive results on different baseline show that training with carefully selected samples leads to significant improvements for different MOT methods.
更多查看译文
关键词
multi-object tracking,noise sample,occlusion,re-identification
AI 理解论文
溯源树
样例
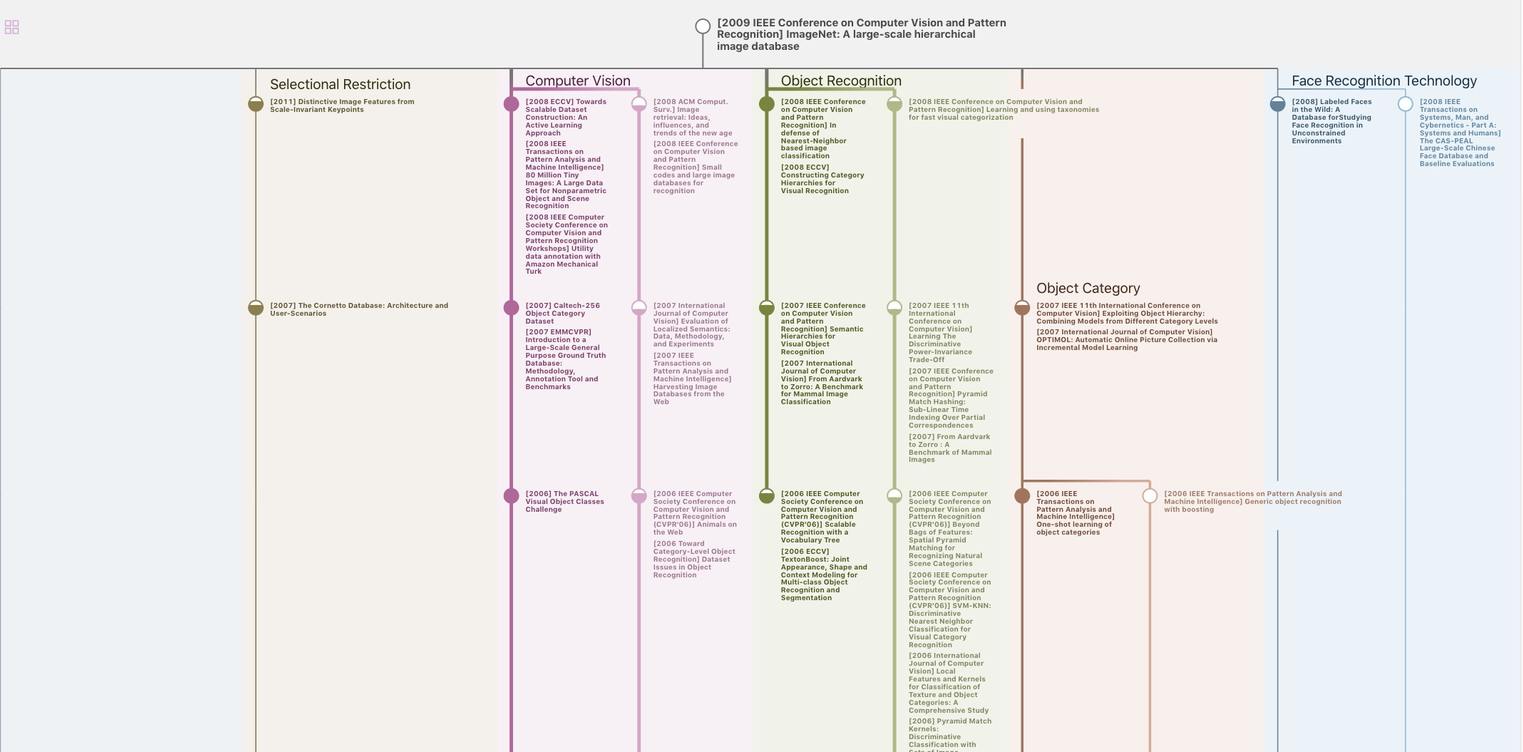
生成溯源树,研究论文发展脉络
Chat Paper
正在生成论文摘要