An Accurate Defect Detection Model for Photosensitive Elements Based on Improved YOLOV4 Deep Neural Network
2022 5th International Conference on Pattern Recognition and Artificial Intelligence (PRAI)(2022)
Abstract
As the main component of camera production, the quality of the photosensitive elements directly affects the imaging quality. To solve the problem of missed and difficult detection of the current photosensitive elements defect detection methods, this paper proposes a photosensitive elements defect detection algorithm based on transfer learning. First, the artificial synthetic defect is used to address the large number of samples requirement of deep learning modeling. Second, K-Means++ is used to select the optimal anchor box for the defect data set to increase the accuracy of the network location. Furthermore, the cosine annealing scheduler and a fixed learning rate are used to improve the convergence speed and overall performance of the network. Finally, a two-branch joint detection network is designed to detect stain and dead pixel effectively. The proposed method has achieved better results than the original algorithm: the average precision (AP) of the defects of dead pixels and stains is greatly improved from 81.08% to 96.92%, and 84.81% to 91.36%, respectively, and the mean average precision (mAP) is 94.14%.
MoreTranslated text
Key words
YOLOV4,photosensitive elements,deep learning,defect detection,transfer learning
AI Read Science
Must-Reading Tree
Example
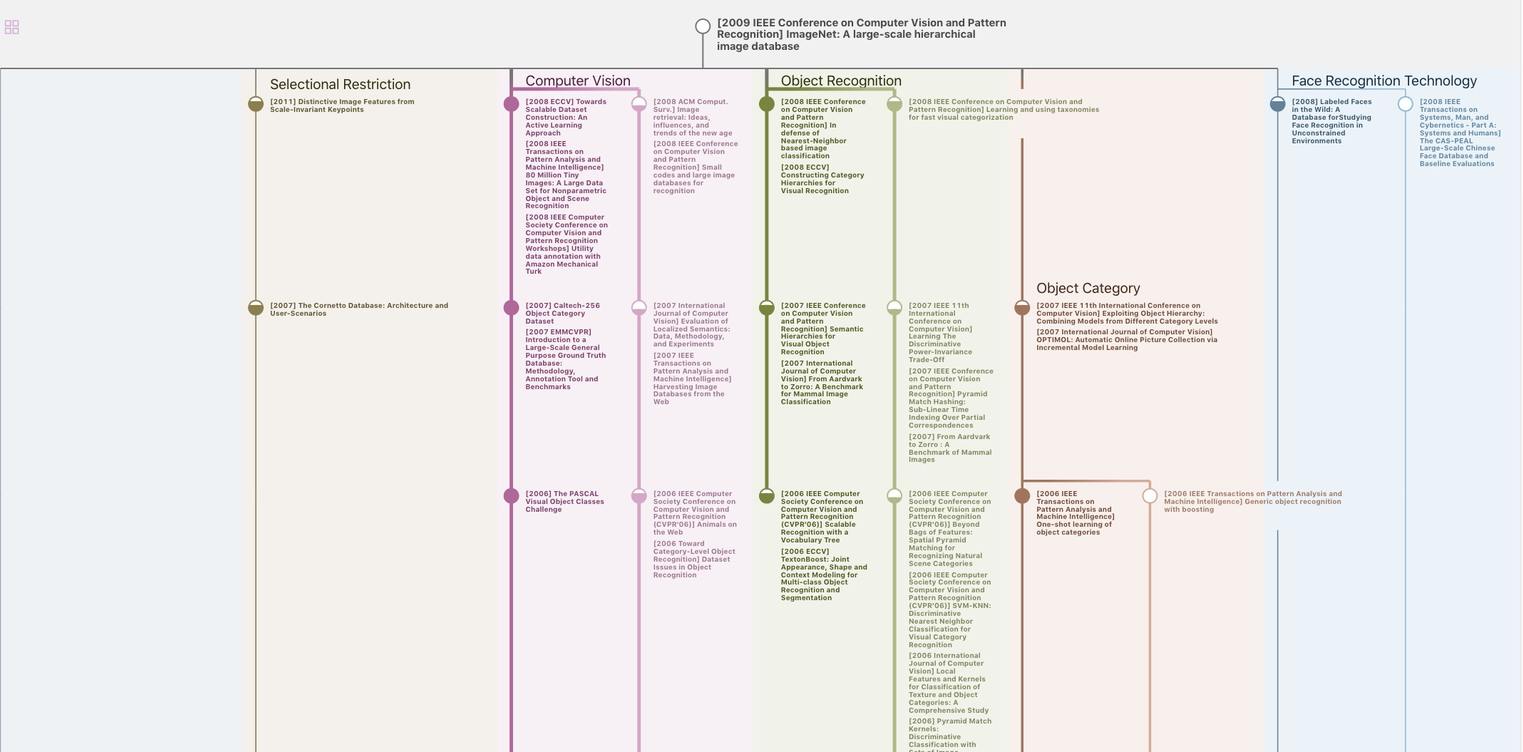
Generate MRT to find the research sequence of this paper
Chat Paper
Summary is being generated by the instructions you defined