Iterative Rule-Guided Reasoning over Sparse Knowledge Graphs with Deep Reinforcement Learning
Information processing & management(2022)
摘要
In recent years, reasoning over knowledge graphs (KGs) has been widely adapted to empower retrieval systems, recommender systems, and question answering systems, generating a surge in research interest. Recently developed reasoning methods usually suffer from poor performance when applied to incomplete or sparse KGs, due to the lack of evidential paths that can reach target entities. To solve this problem, we propose a hybrid multi-hop reasoning model with reinforcement learning (RL) called SparKGR, which implements dynamic path completion and iterative rule guidance strategies to increase reasoning performance over sparse KGs. Firstly, the model dynamically completes the missing paths using rule guidance to augment the action space for the RL agent; this strategy effectively reduces the sparsity of KGs, thus increasing path search efficiency. Secondly, an iterative optimization of rule induction and fact inference is designed to incorporate global information from KGs to guide the RL agent exploration; this optimization iteratively improves overall training performance. We further evaluated the SparKGR model through different tasks on five real world datasets extracted from Freebase, Wikidata and NELL. The experimental results indicate that SparKGR outperforms state-of-the-art baseline models without losing interpretability.
更多查看译文
关键词
Knowledge graphs,Deep reinforcement learning,Rule guidance,Iterative reasoning strategy
AI 理解论文
溯源树
样例
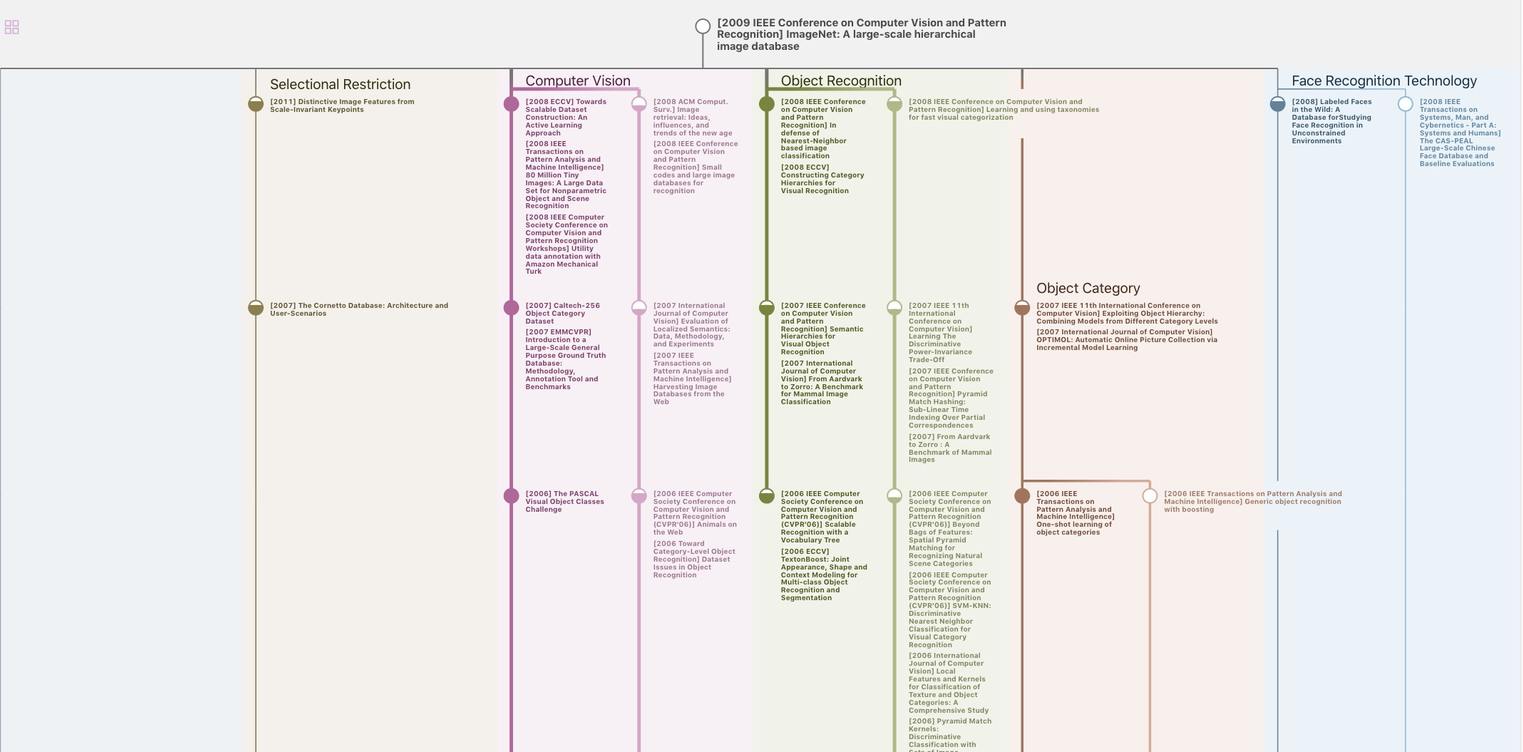
生成溯源树,研究论文发展脉络
Chat Paper
正在生成论文摘要