HVLM: Exploring Human-Like Visual Cognition and Language-Memory Network for Visual Dialog
Information Processing & Management(2022)
摘要
Visual dialog, a visual-language task, enables an AI agent to engage in conversation with humans grounded in a given image. To generate appropriate answers for a series of questions in the dialog, the agent is required to understand the comprehensive visual content of an image and the fine-grained textual context of the dialog. However, previous studies typically utilized the object-level visual feature to represent a whole image, which only focuses on the local perspective of an image but ignores the importance of the global information in an image. In this paper, we proposed a novel model Human-Like Visual Cognitive and Language-Memory Network for Visual Dialog (HVLM), to simulate global and local dual-perspective cognitions in the human visual system and understand an image comprehensively. HVLM consists of two key modules, Local-to-Global Graph Convolutional Visual Cognition (LG-GCVC) and Question-guided Language Topic Memory (T-Mem). Specifically, in the LG-GCVC module, we design a question-guided dual-perspective reasoning to jointly learn visual contents from both local and global perspectives through a simple spectral graph convolution network. Furthermore, in the T-Mem module, we design an iterative learning strategy to gradually enhance fine-grained textual context details via an attention mechanism. Experimental results demonstrate the superiority of our proposed model, which obtains the comparable performance on benchmark datasets VisDial v1.0 and VisDial v0.9.
更多查看译文
关键词
Visual Dialog,Visual-language understanding,Dual-perspective reasoning,Simple spectral graph convolution network
AI 理解论文
溯源树
样例
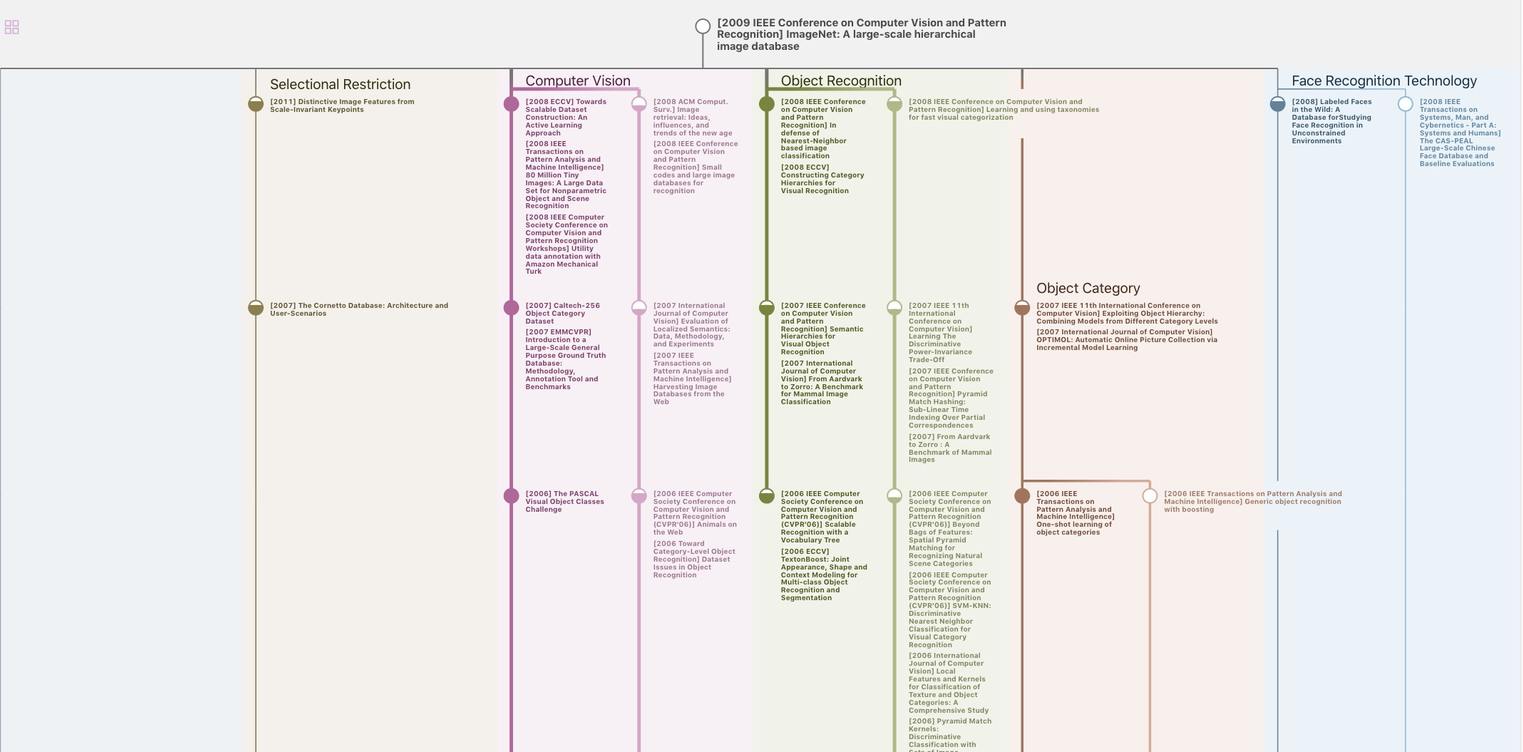
生成溯源树,研究论文发展脉络
Chat Paper
正在生成论文摘要