TCACNet: Temporal and channel attention convolutional network for motor imagery classification of EEG-based BCI
Information Processing & Management(2022)
摘要
Brain–computer interface (BCI) is a promising intelligent healthcare technology to improve human living quality across the lifespan, which enables assistance of movement and communication, rehabilitation of exercise and nerves, monitoring sleep quality, fatigue and emotion. Most BCI systems are based on motor imagery electroencephalogram (MI-EEG) due to its advantages of sensory organs affection, operation at free will and etc. However, MI-EEG classification, a core problem in BCI systems, suffers from two critical challenges: the EEG signal’s temporal non-stationarity and the nonuniform information distribution over different electrode channels. To address these two challenges, this paper proposes TCACNet, a temporal and channel attention convolutional network for MI-EEG classification. TCACNet leverages a novel attention mechanism module and a well-designed network architecture to process the EEG signals. The former enables the TCACNet to pay more attention to signals of task-related time slices and electrode channels, supporting the latter to make accurate classification decisions. We compare the proposed TCACNet with other state-of-the-art deep learning baselines on two open source EEG datasets. Experimental results show that TCACNet achieves 11.4% and 7.9% classification accuracy improvement on two datasets respectively. Additionally, TCACNet achieves the same accuracy as other baselines with about 50% less training data. In terms of classification accuracy and data efficiency, the superiority of the TCACNet over advanced baselines demonstrates its practical value for BCI systems.
更多查看译文
关键词
Brain–computer interface,Electroencephalogram,Motor imagery classification,Deep learning,Attention mechanism
AI 理解论文
溯源树
样例
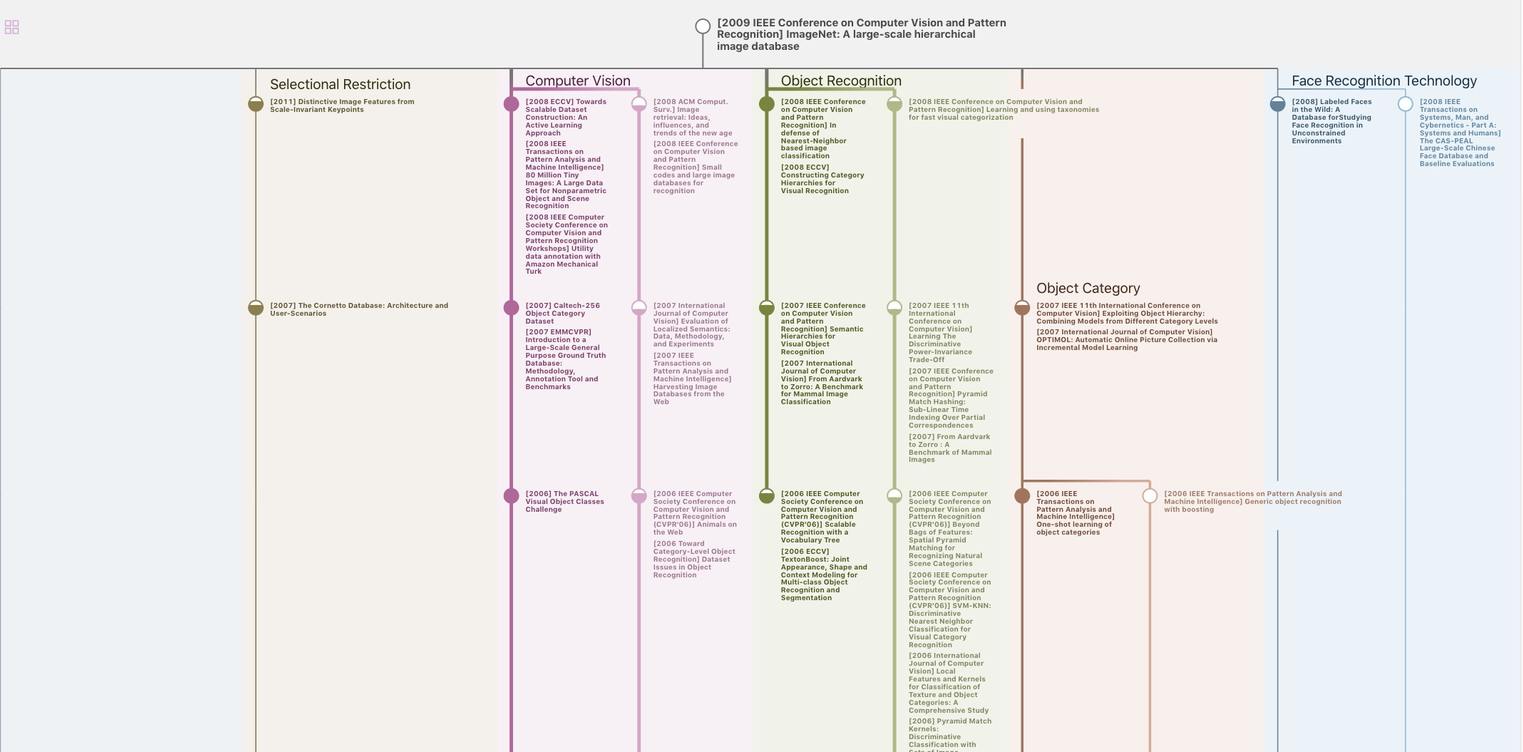
生成溯源树,研究论文发展脉络
Chat Paper
正在生成论文摘要