Digitizing paper based ECG files to foster deep learning based analysis of existing clinical datasets: An exploratory analysis
Intelligence-Based Medicine(2022)
摘要
Recently, a deep learning model was developed and validated for detecting left ventricular dysfunction based on a standard 12-lead ECG. However, this model largely depends on the availability of digital ECG data: 10 s for all 12 leads sampled at 500 Hz stored as a numeric array. This limits the ability to validate or scale this technology to institutions that store ECGs as PDF or image files (“paper” ECGs). Methods do exist to create digital signals from the archived paper copies of the ECGs. The primary objective of this study was to evaluate how well the AI-ECG model output obtained using digitized paper ECGs agreed with the predictions from the native digital ECGs for the detection of low ejection fraction. To address this objective, deep learning models that utilizes digitized data from a 12-lead ECG snapshot were needed. Two models were evaluated, Model A using data from a single lead with full 10-s recording (lead II) only and Model B using data from 3 leads with 10-s recordings (leads II, V1 and V5) in addition to 9 leads with partial (2.5-s) recordings. In a test sample of 10 patients with varying ECG features, Models A and B obtained intraclass correlation coefficients of 0.95 (95% CI: 0.82 to 0.99) and 0.58 (95% CI: 0.00 to 0.87). In an exploratory examination of model diagnostic performance to detect low ejection fraction, Model A achieved and AUC of 0.71 while Model B achieved an AUC of 0.91. Our study demonstrates an agreement between deep learning model predictions obtained from digitized paper-based ECGs and native digital ECGs and provides some insight into potential expandability of ECG-based deep learning models including the importance of captured duration (10-s vs. 2-5-s recordings) and ECG vectors (precordial leads vs. limb leads).
更多查看译文
关键词
ECG,Machine learning,Deep learning,Electronic health record
AI 理解论文
溯源树
样例
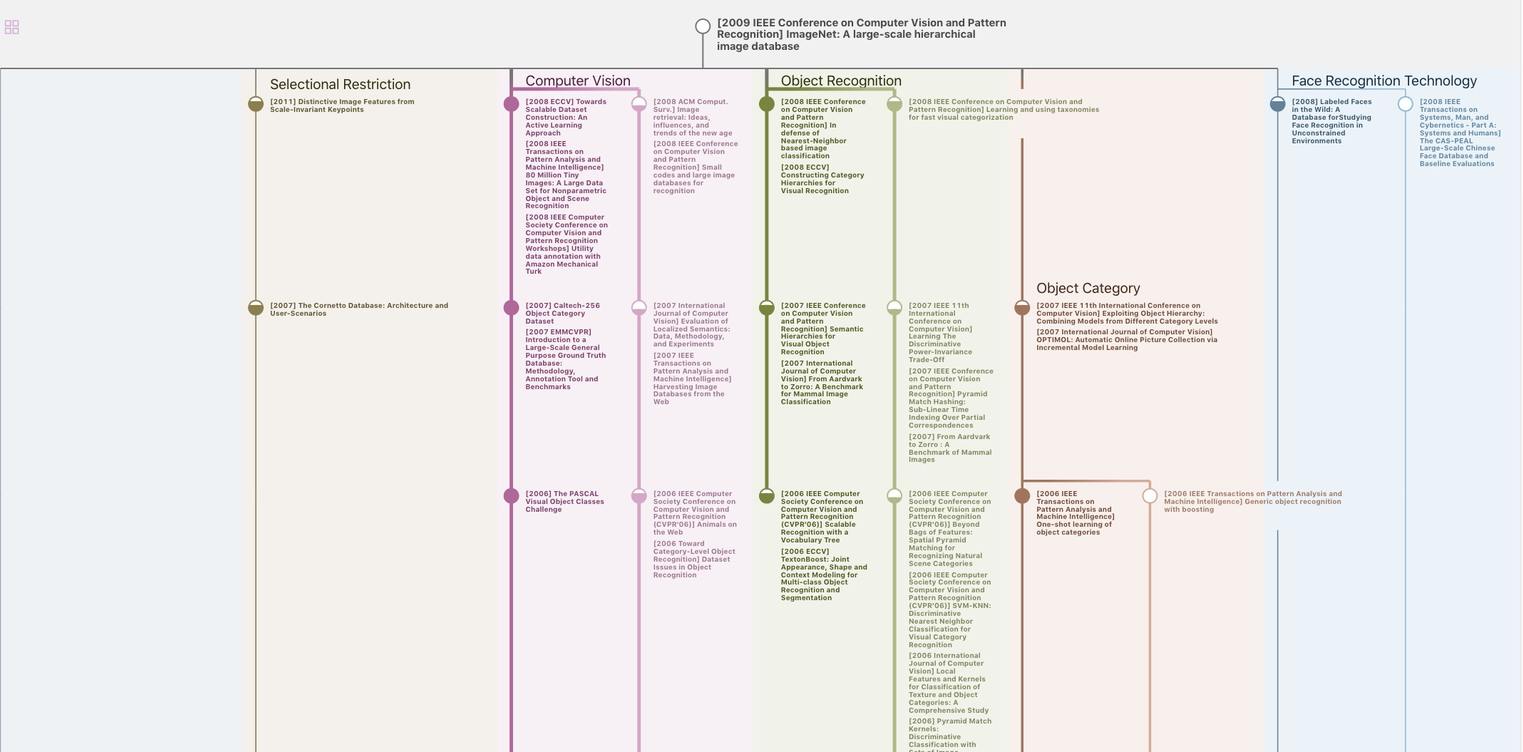
生成溯源树,研究论文发展脉络
Chat Paper
正在生成论文摘要