Prospective clinical evaluation of a machine-learning trained algorithm for detection of arterial pressure transducer drop
Intelligence-Based Medicine(2022)
摘要
Background
Invasive arterial pressure monitoring is frequently used in operating room and intensive care unit patients to provide continuous assessment of hemodynamics. We have previously developed an algorithm trained to detect error of >10 mmHg in leveling of the arterial pressure transducer from only the features of the waveform itself. In the present study we used the detection algorithm in real-time during surgical cases where the primary team had placed an arterial line for hemodynamic monitoring and tested its ability to detected introduced transducer drop errors.
Methods
Patients having surgery at UCI Medical Center who were 18 years or older and were expected to have invasive arterial pressure monitoring as part of their anesthetic care were eligible for enrollment. The arterial pressure waveform was captured during surgery and at random times the transducer was dropped from its secured location. The ability of the previously trained algorithm to detect these drops from the waveform alone was assessed in real-time as well as false-positives.
Results
A total of 50 patients were ultimately included for over 80 h of monitoring time. A total of 78 drop tests were performed in that time. Overall, the algorithm detected 69 of these drops (88%), however detection for errors of 10 mmHg or more was 98% and for >12 mmHg was 100%. The overall false positive rate was 0.65% and balanced accuracy was 0.99 for errors >10 mmHg.
Conclusion
The algorithm was highly sensitive for detection of transducer drops resulting in error of ≥10 mmHg in real-time, with a very low false-positive rate. This algorithm may be of value in applications utilizing the arterial line for input where safety is essential.
更多查看译文
关键词
Monitoring error,Invasive blood pressure measurement,Machine learning
AI 理解论文
溯源树
样例
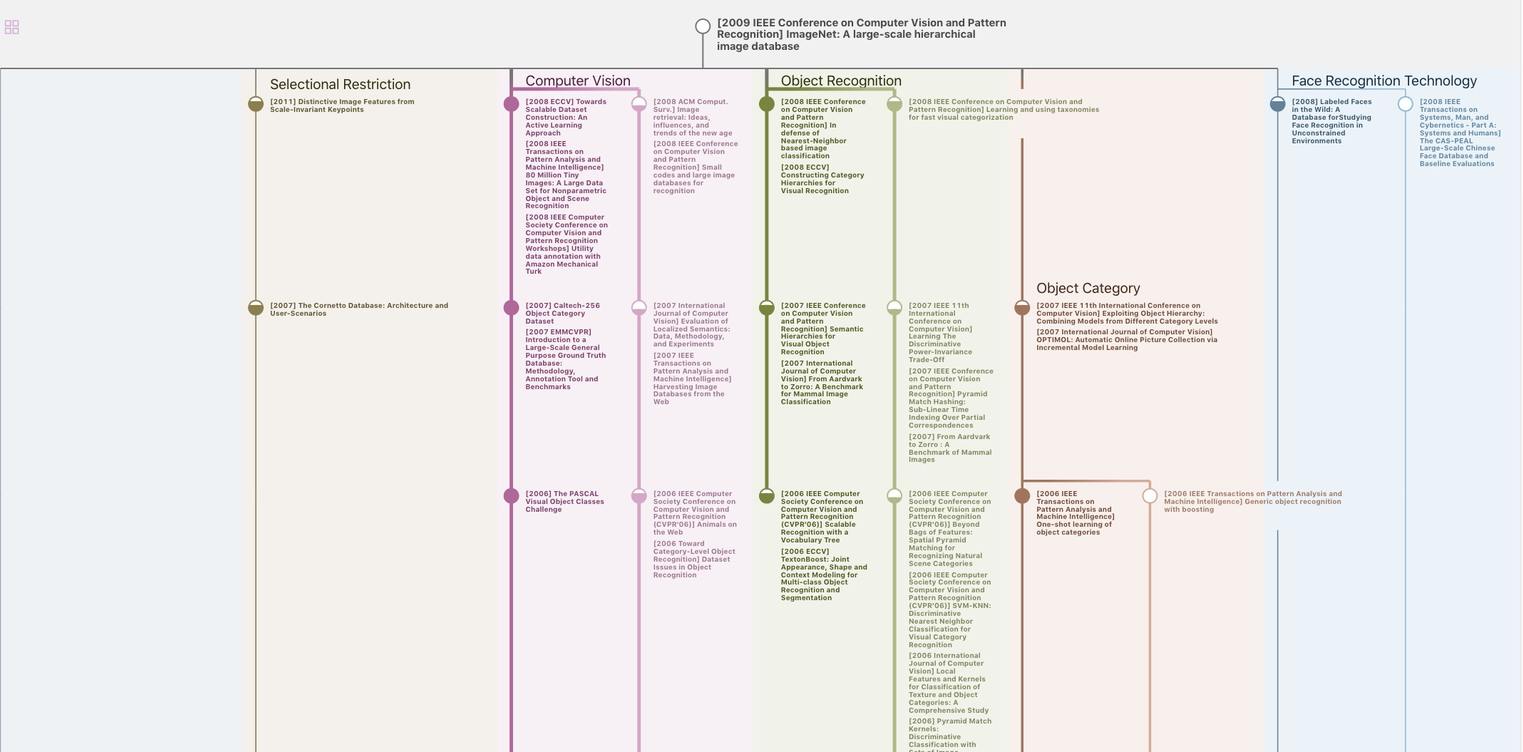
生成溯源树,研究论文发展脉络
Chat Paper
正在生成论文摘要