Hybrid ResNet Based on Joint Basic and Attention Modules for Long-Tailed Classification
International journal of approximate reasoning(2022)
Abstract
Long-tailed distribution learning is one of the critical research fields of deep learning and has gradually become a research hotspot. Existing re-sampling methods for long-tailed data classification attempt to adjust the number of tail class samples to balance the overall feature space and achieve satisfactory results. However, the methods impair the representative ability of the learned features to a certain extent, which in turn affects the tail class feature space. In this paper, we propose a hybrid ResNet based on joint basic and attention modules to enhance the tail class feature space, which provides rich discriminative and representative features in the tail class feature space. Firstly, we use hybrid ResNet to extract features, where the basic module ResNet and the attention module ResNet extract head and tail class features, respectively. The enhancement of tail class features can reduce the dependence of the classifier on head class features. Secondly, we build a fusion loss function, which considers the tradeoff between head loss and tail loss for long-tailed distribution learning. Experimental results show that the proposed model outperforms several state-of-the-art models in the long-tailed classification. Our model was 2.67% better than the optimal method under the long-tailed Tiny-Imagenet-LT dataset with an imbalanced ratio of 100.
MoreTranslated text
Key words
Deep learning,Long-tailed distribution learning,Hybrid ResNet,Attention module
AI Read Science
Must-Reading Tree
Example
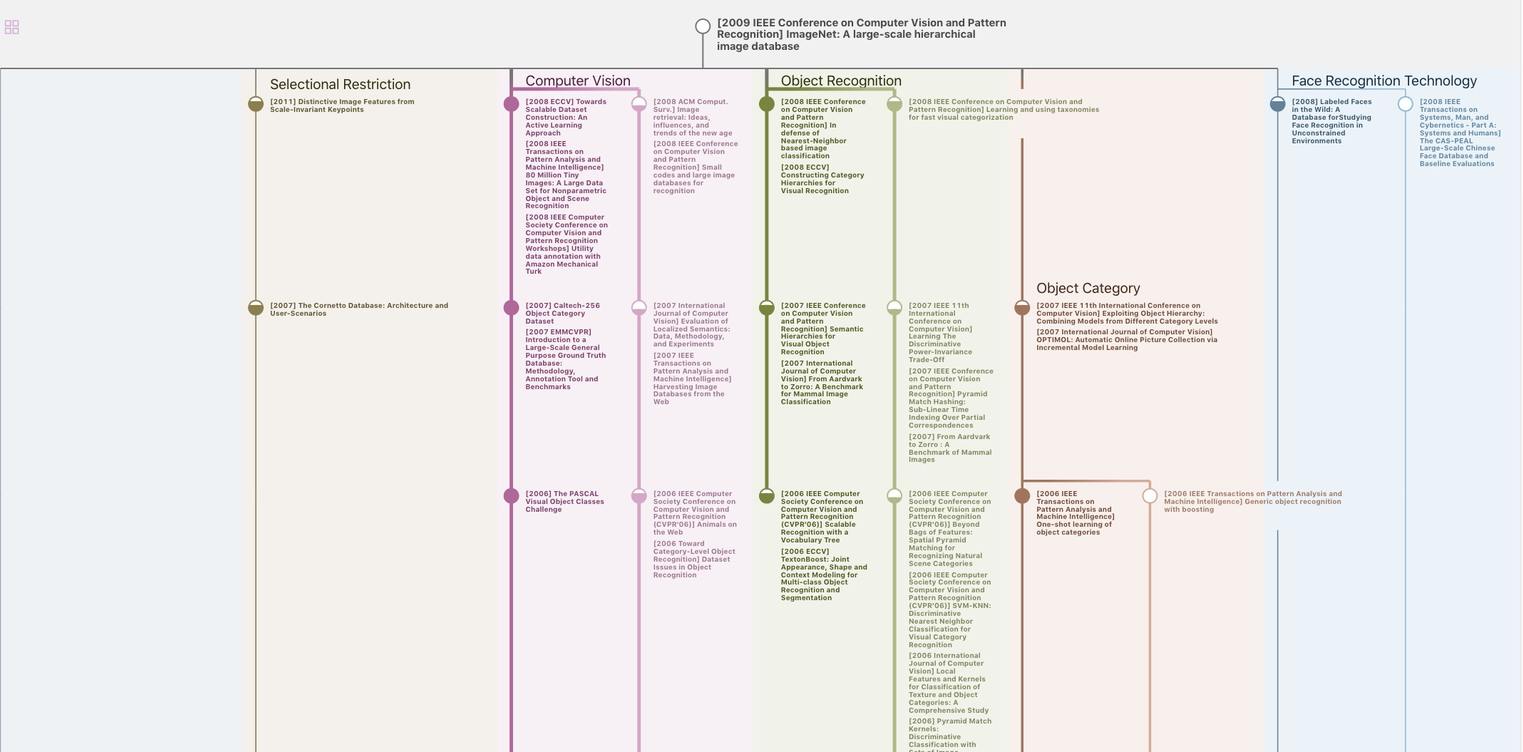
Generate MRT to find the research sequence of this paper
Chat Paper
Summary is being generated by the instructions you defined