Digital Modeling Approach of Distributional Mapping from Structural Temperature Field to Temperature-Induced Strain Field for Bridges
Journal of civil structural health monitoring(2022)
摘要
Zero-point of strain data representing the structural state without stress is hard to determine, but change in strain can be accurately measured. It is a good choice to quantify the complex strain behavior under non-uniform temperature field by deep learning the variation of distributional features from different sensing points. Taking a long-span steel cable-stayed bridge as the case study, features of long-term time series data of temperature and temperature-induced strain are analyzed. A digital approach of distributional mapping from the structural temperature field to the temperature-induced strain field is presented. Based on the coordinates clustering of sensing points and the correlation knowledge between structural temperature and temperature-induced strain, clusters of sensing points of temperature and strain can be determined. Distributional feature parameters (difference sequence and adjacency matrix of difference) about the per-minute mean of each cluster’s structural temperature and temperature-induced strain data are calculated. The model of mapping relation from structural temperature field to temperature-induced strain field is established based on the learning of the big data of distributional feature parameters by the bidirectional long short-term memory regression network. The results demonstrated that redistribution of temperature-induced strain field can be perceived according to the residual between regression results of network models and real-time monitoring results, which means extreme changes of temperature field or potential deterioration in structure of bridge.
更多查看译文
关键词
Structural health monitoring,Digital twin,Deep learning,Cluster,Temperature-induced strain
AI 理解论文
溯源树
样例
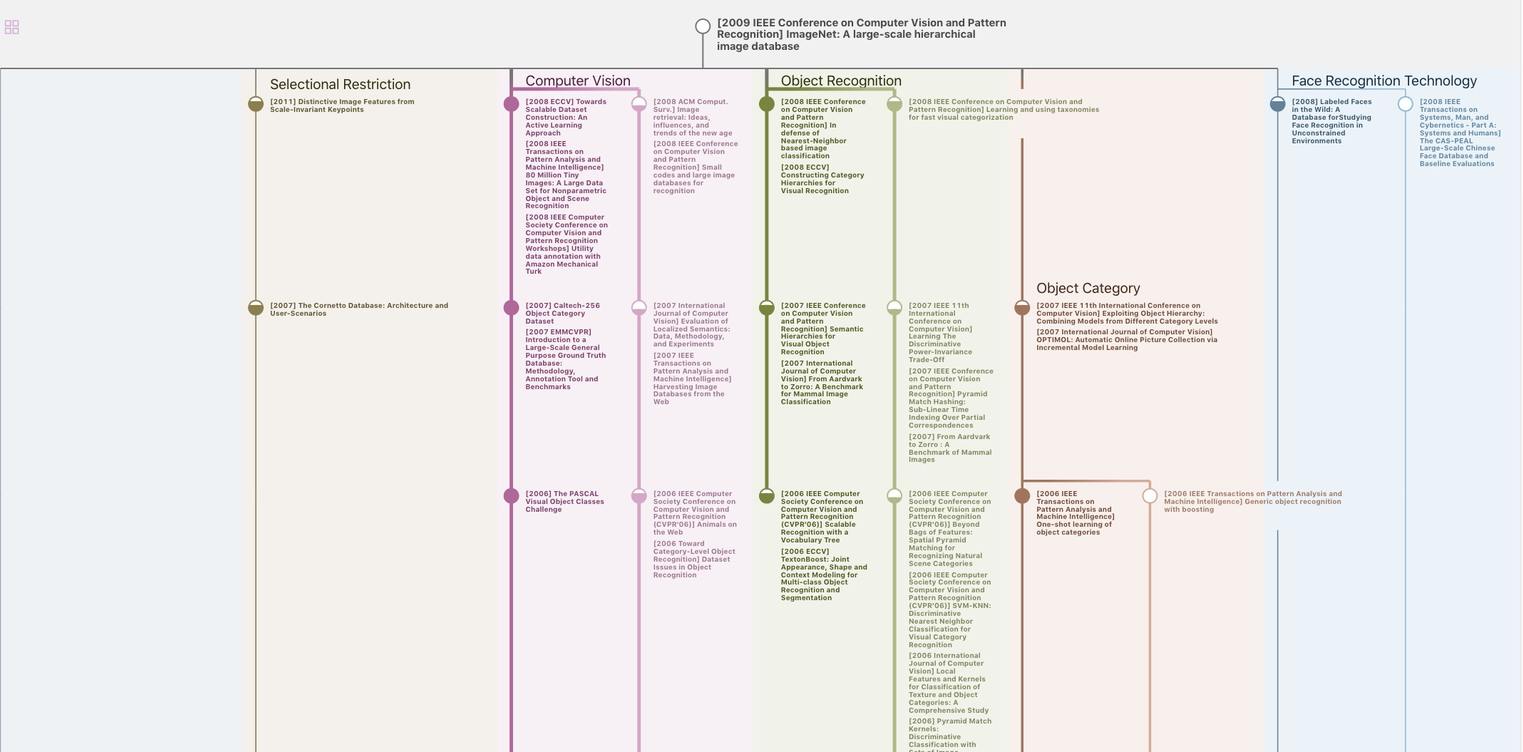
生成溯源树,研究论文发展脉络
Chat Paper
正在生成论文摘要