SALoBa: Maximizing Data Locality and Workload Balance for Fast Sequence Alignment on GPUs
2022 IEEE International Parallel and Distributed Processing Symposium (IPDPS)(2022)
摘要
Sequence alignment forms an important backbone in many sequencing applications. A commonly used strategy for sequence alignment is an approximate string matching with a two-dimensional dynamic programming approach. Although some prior work has been conducted on GPU acceleration of a sequence alignment, we identify several shortcomings that limit exploiting the full computational capability of modern GPUs. This paper presents SALoBa, a GPU-accelerated sequence alignment library focused on seed extension. Based on the analysis of previous work with real-world sequencing data, we propose techniques to exploit the data locality and improve work-load balancing. The experimental results reveal that SALoBa significantly improves the seed extension kernel compared to state-of-the-art GPU-based methods.
更多查看译文
关键词
Genome sequencing,Sequence alignment,Smith-Waterman,GPU acceleration
AI 理解论文
溯源树
样例
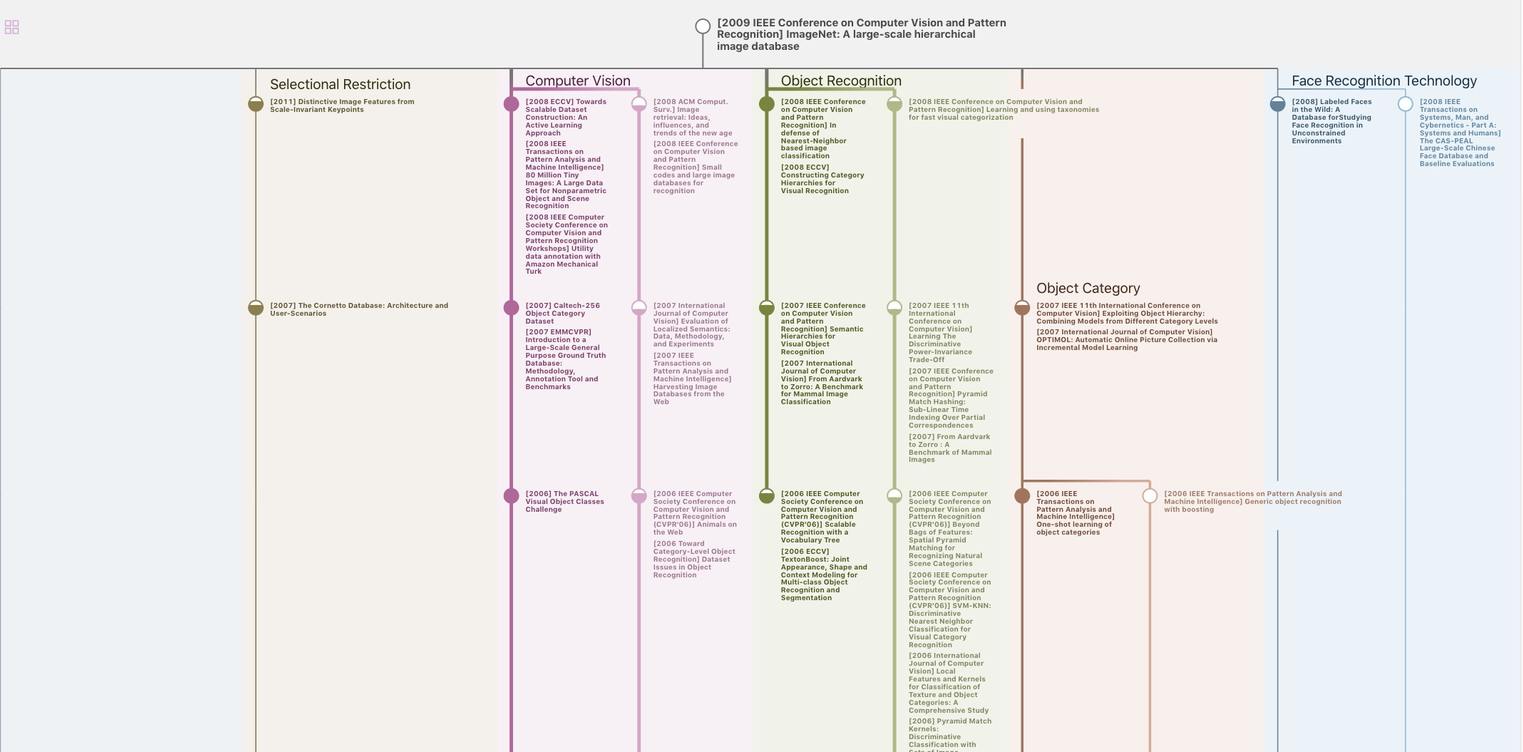
生成溯源树,研究论文发展脉络
Chat Paper
正在生成论文摘要