Tailored Risk Stratification in Severe Mitral Regurgitation and Heart Failure Using Supervised Learning Techniques
JACC: Advances(2022)
摘要
Background
Secondary mitral regurgitation (sMR) in the setting of heart failure (HF) has considerable impact on quality of life, HF rehospitalizations, and mortality. Identification of high-risk cohorts is essential to understand disease trajectories and for risk stratification.
Objectives
This study aimed to provide a structured decision tree–like approach to risk stratification in patients with severe sMR and HF.
Methods
This observational study included 1,317 patients with severe sMR from the entire HF spectrum. Clinical, echocardiographic, and laboratory data were extracted for all patients. The primary end point was all-cause mortality. Survival tree analysis, a supervised learning technique, was applied to identify patient subgroups at risk of mortality and further stratified by HF subtype (preserved, mildly reduced, and reduced ejection fraction).
Results
Using supervised learning (survival tree method), 8 distinct subgroups were identified that differed significantly in long-term survival. Subgroup 7, characterized by younger age (≤66 years), higher hemoglobin (>12.7 g/dL), and higher albumin levels (>40.6 g/L) had the best survival. In contrast, subgroup 5 displayed a 20-fold risk of mortality (hazard ratio: 20.38 [95% CI: 10.78-38.52]); P < 0.001 and had older age (>68 years), low serum albumin (≤40.6 g/L), and higher NT-proBNP levels (≥9,750 pg/mL). Unique subgroups were further identified for each type of HF subtypes.
Conclusions
Supervised machine learning reveals heterogeneity in the sMR risk spectrum, highlighting the clinical variability in the population. A decision tree–like model can help identify differences in outcomes among subgroups and can help provide tailored risk stratification.
更多查看译文
关键词
heart failure,HFrEF,HFmrEF,HFpEF,machine learning,secondary mitral regurgitation,supervised learning
AI 理解论文
溯源树
样例
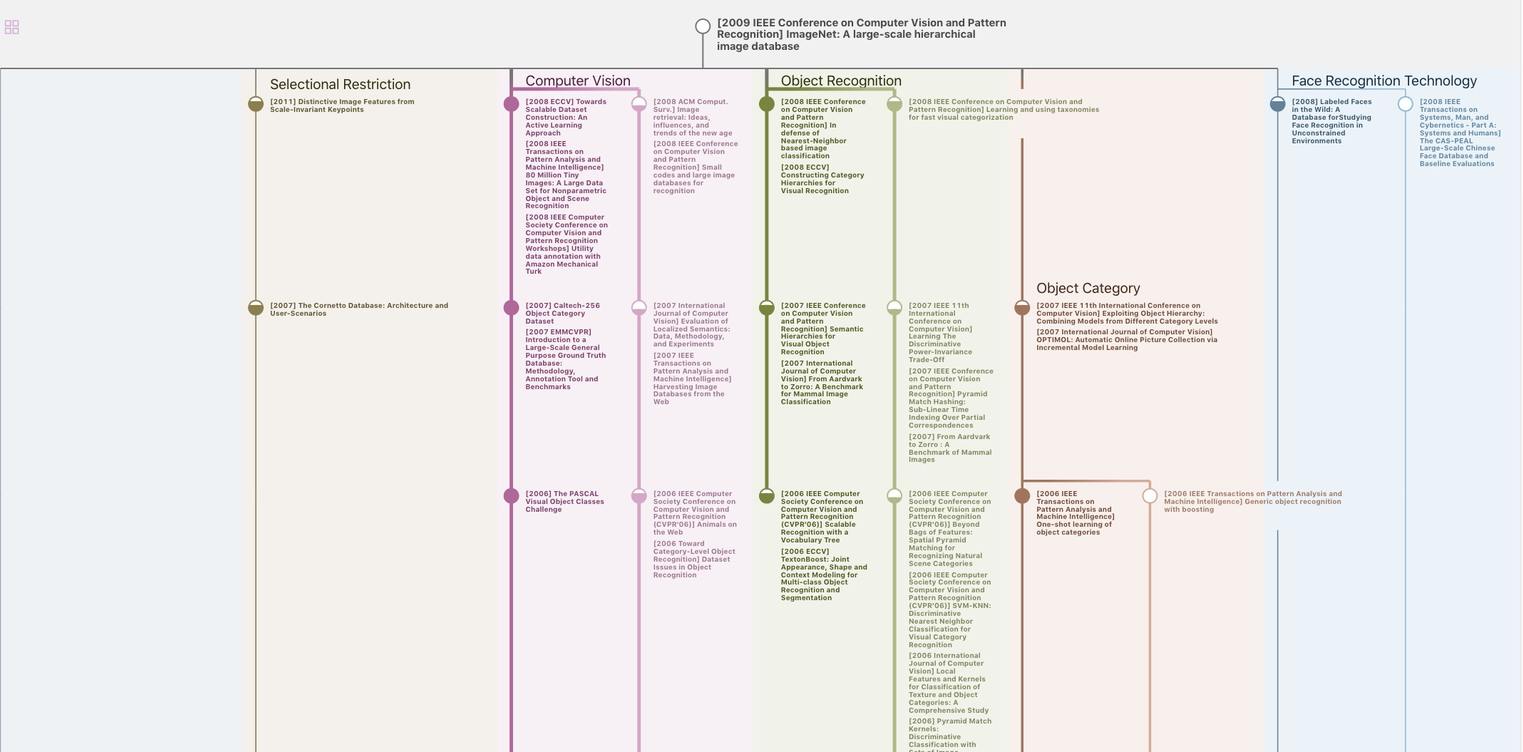
生成溯源树,研究论文发展脉络
Chat Paper
正在生成论文摘要