Sleep postures monitoring based on capacitively coupled electrodes and deep recurrent neural networks
BIOMEDICAL ENGINEERING ONLINE(2022)
摘要
Background Capacitively coupled electrode (CC electrode), as a non-contact and unobtrusive technology for measuring physiological signals, has been widely applied in sleep monitoring scenarios. The most common implementation is capacitive electrocardiogram (cECG) that could provide useful clinical information for assessing cardiac function and detecting cardiovascular diseases. In the current study, we sought to explore another potential application of cECG in sleep monitoring, i.e., sleep postures recognition. Methods Two sets of experiments, the short-term experiment, and the overnight experiment, were conducted. The cECG signals were measured by a smart mattress based on flexible CC electrodes and sleep postures were recorded simultaneously. Then, a classifier model based on a deep recurrent neural network (RNN) was proposed to distinguish sleep postures (supine, left lateral and right lateral). To verify the reliability of the proposed model, leave-one-subject-out cross-validation was introduced. Results In the short-term experiment, the overall accuracy of 96.2% was achieved based on 30-s segment, while the overall accuracy was 88.8% using one heart beat segment. For the unconstrained overnight experiment, the accuracy of 91.0% was achieved based on 30-s segment, while the accuracy was 81.4% using one heart beat segment. Conclusions The results suggest that cECG could render valuable information about sleep postures detection and potentially be helpful for sleep disorder diagnosis.
更多查看译文
关键词
Capacitively coupled electrode, Sleep posture, Capacitive electrocardiogram, Recurrent neural network
AI 理解论文
溯源树
样例
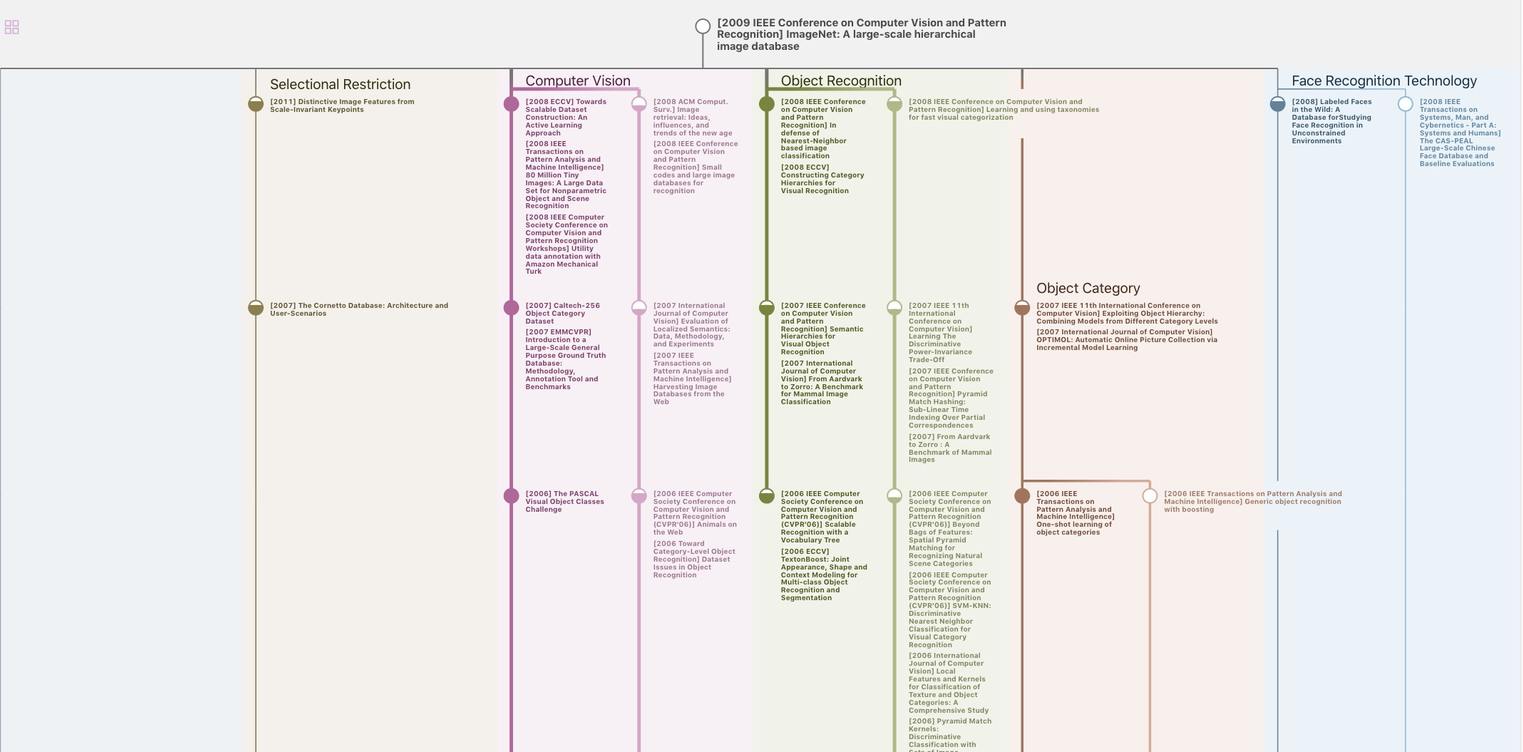
生成溯源树,研究论文发展脉络
Chat Paper
正在生成论文摘要