GWLBC: Gray Wolf Optimization Based Load Balanced Clustering for Sustainable WSNs in Smart City Environment
SENSORS(2022)
摘要
In a smart city environment, with increased demand for energy efficiency, information exchange and communication through wireless sensor networks (WSNs) plays an important role. In WSNs, the sensors are usually operating in clusters, and they are allowed to restructure for effective communication over a large area and for a long time. In this scenario, load-balanced clustering is the cost-effective means of improving the system performance. Although clustering is a discrete problem, the computational intelligence techniques are more suitable for load balancing and minimizing energy consumption with different operating constraints. The literature reveals that the swarm intelligence-inspired computational approaches give excellent results among population-based meta-heuristic approaches because of their more remarkable exploration ability. Conversely, in this work, load-balanced clustering for sustainable WSNs is presented using improved gray wolf optimization (IGWO). In a smart city environment, the significant parameters of energy-efficient load-balanced clustering involve the network lifetime, dead cluster heads, dead gateways, dead sensor nodes, and energy consumption while ensuring information exchange and communication among the sensors and cluster heads. Therefore, based on the above parameters, the proposed IGWO is compared with the existing GWO and several other techniques. Moreover, the convergence characteristics of the proposed algorithm are demonstrated for an extensive network in a smart city environment, which consists of 500 sensors and 50 cluster heads deployed in an area of 500 x 500 m(2), and it was found to be significantly improved.
更多查看译文
关键词
improved gray wolf optimization,clustering,load balancing,performance modeling,sustainable WSNs
AI 理解论文
溯源树
样例
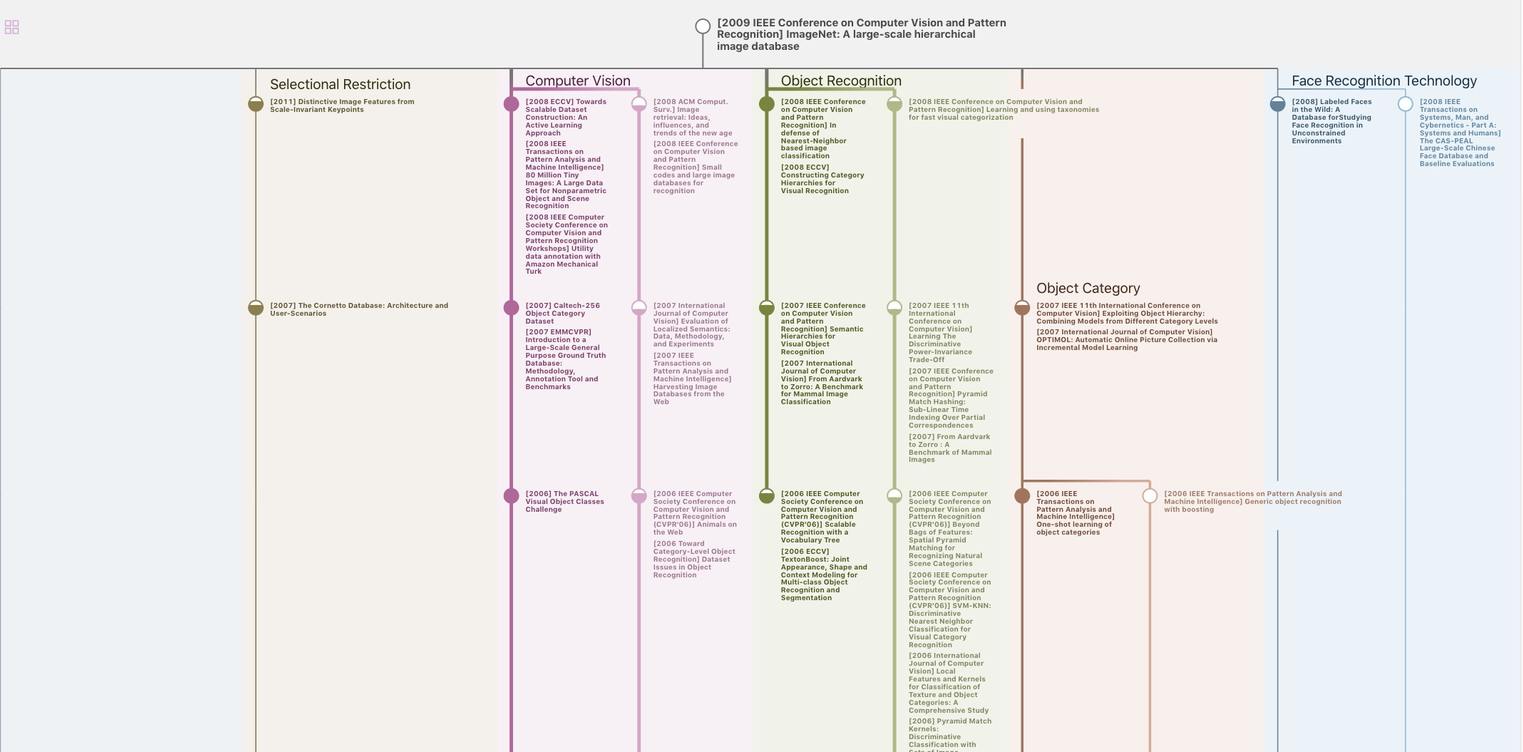
生成溯源树,研究论文发展脉络
Chat Paper
正在生成论文摘要