Comparing Handcrafted Features and Deep Neural Representations for Domain Generalization in Human Activity Recognition
SENSORS(2022)
摘要
Human Activity Recognition (HAR) has been studied extensively, yet current approaches are not capable of generalizing across different domains (i.e., subjects, devices, or datasets) with acceptable performance. This lack of generalization hinders the applicability of these models in real-world environments. As deep neural networks are becoming increasingly popular in recent work, there is a need for an explicit comparison between handcrafted and deep representations in Out-of-Distribution (OOD) settings. This paper compares both approaches in multiple domains using homogenized public datasets. First, we compare several metrics to validate three different OOD settings. In our main experiments, we then verify that even though deep learning initially outperforms models with handcrafted features, the situation is reversed as the distance from the training distribution increases. These findings support the hypothesis that handcrafted features may generalize better across specific domains.
更多查看译文
关键词
human activity recognition,deep learning,domain generalization,accelerometer
AI 理解论文
溯源树
样例
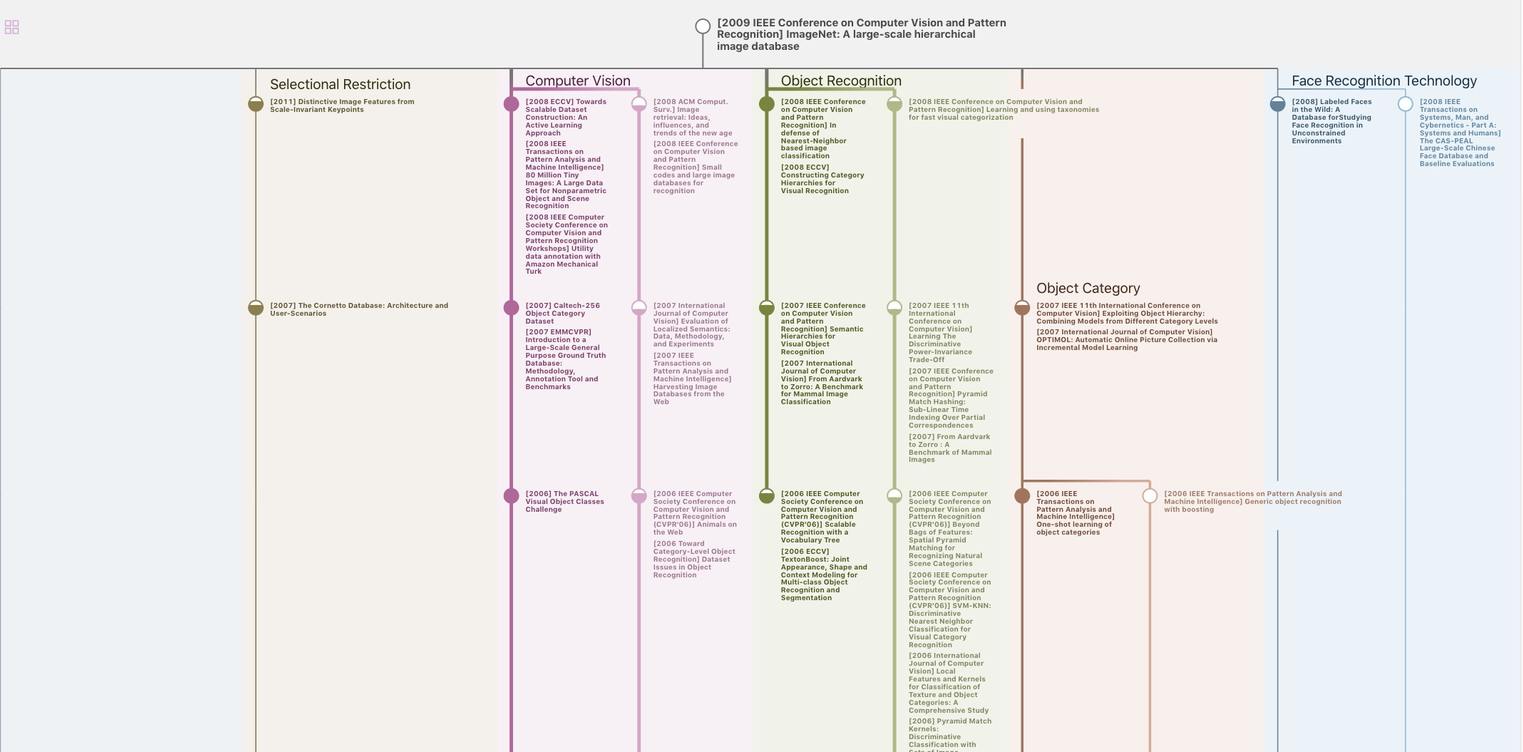
生成溯源树,研究论文发展脉络
Chat Paper
正在生成论文摘要