Machine Learning-Based Peripheral Artery Disease Identification Using Laboratory-Based Gait Data.
Sensors(2022)
摘要
Peripheral artery disease (PAD) manifests from atherosclerosis, which limits blood flow to the legs and causes changes in muscle structure and function, and in gait performance. PAD is underdiagnosed, which delays treatment and worsens clinical outcomes. To overcome this challenge, the purpose of this study is to develop machine learning (ML) models that distinguish individuals with and without PAD. This is the first step to using ML to identify those with PAD risk early. We built ML models based on previously acquired overground walking biomechanics data from patients with PAD and healthy controls. Gait signatures were characterized using ankle, knee, and hip joint angles, torques, and powers, as well as ground reaction forces (GRF). ML was able to classify those with and without PAD using Neural Networks or Random Forest algorithms with 89% accuracy (0.64 Matthew’s Correlation Coefficient) using all laboratory-based gait variables. Moreover, models using only GRF variables provided up to 87% accuracy (0.64 Matthew’s Correlation Coefficient). These results indicate that ML models can classify those with and without PAD using gait signatures with acceptable performance. Results also show that an ML gait signature model that uses GRF features delivers the most informative data for PAD classification.
更多查看译文
关键词
peripheral artery disease,vascular disease,machine learning,gait analysis,deep learning
AI 理解论文
溯源树
样例
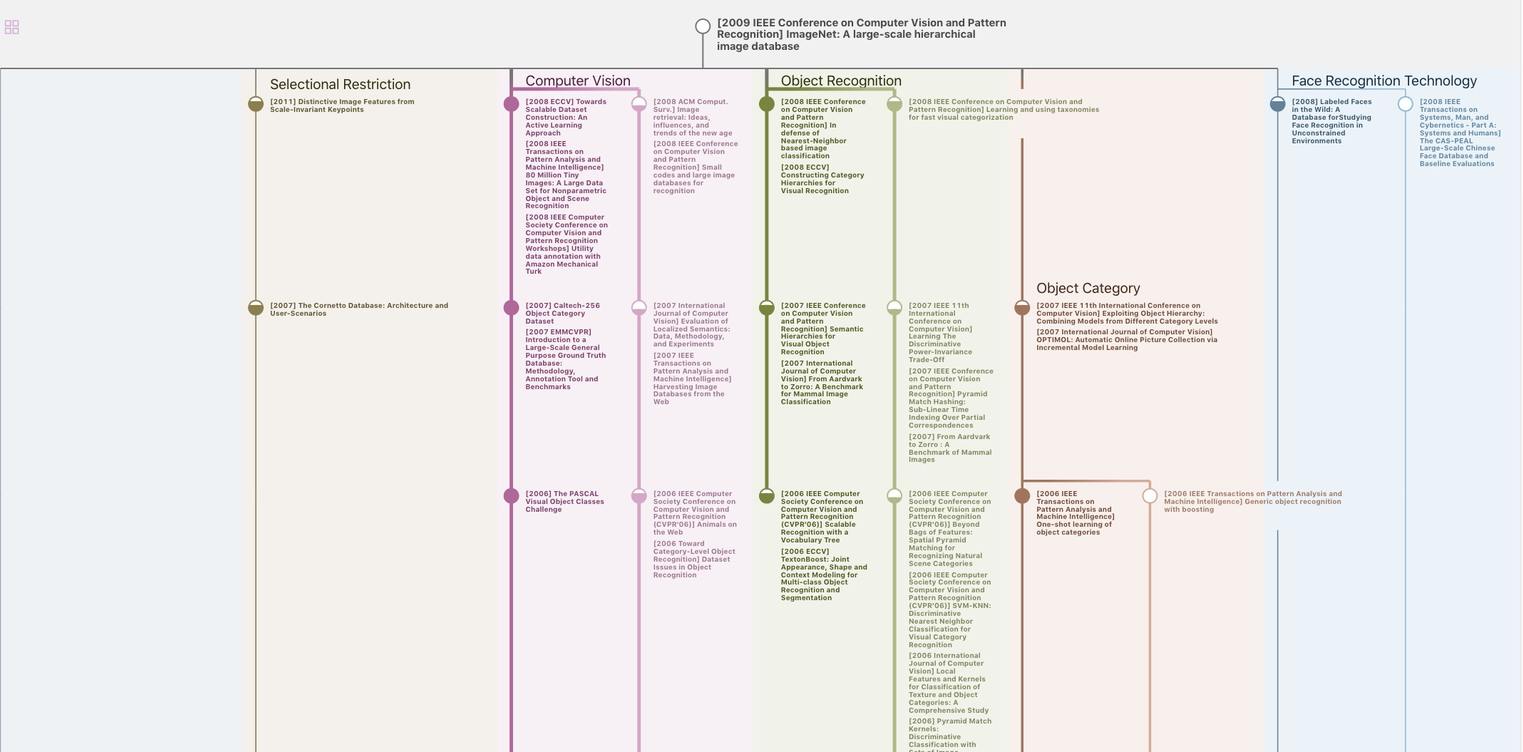
生成溯源树,研究论文发展脉络
Chat Paper
正在生成论文摘要