Clustering-based Location Authority Deep Model in the Next Point-of-Interest Recommendation
WI/IAT(2021)
摘要
With the development of location-based social networks (LBSNs), sequential Point-of-Interest (POI) recommendation is getting more and more vitality. Current sequential POI recommendation models predict user's future mobility based on user's previous behaviors without considering the effect of the significant location (i.e. location authority). Besides, it is hard to effectively capture user interests because of the data sparsity problem in the LBSNs dataset. To this end, we propose a clustering-based location authority deep model (CLADM) that integrates POI clusters and location authority to reduce the scale of POI candidate set to alleviate the issue of data sparsity. In the proposed model, we present two encoders based on the attention mechanism: the first encoder is an additive attention encoder to exploit user preferences on POI clusters, and the second encoder mines user preferences of POIs. Considering that the user check-in data is the implicit feedback, we design a binary self-attention layer in the second encoder with a sigmoid function. We adopt two real-world LBSNs datasets with different scales to evaluate our model. The experimental results show that our proposed model greatly outperforms the state-of-the-art methods for sequential POI recommendation.
更多查看译文
关键词
sequential recommendation, clustering, location authority, attention, POI recommendation
AI 理解论文
溯源树
样例
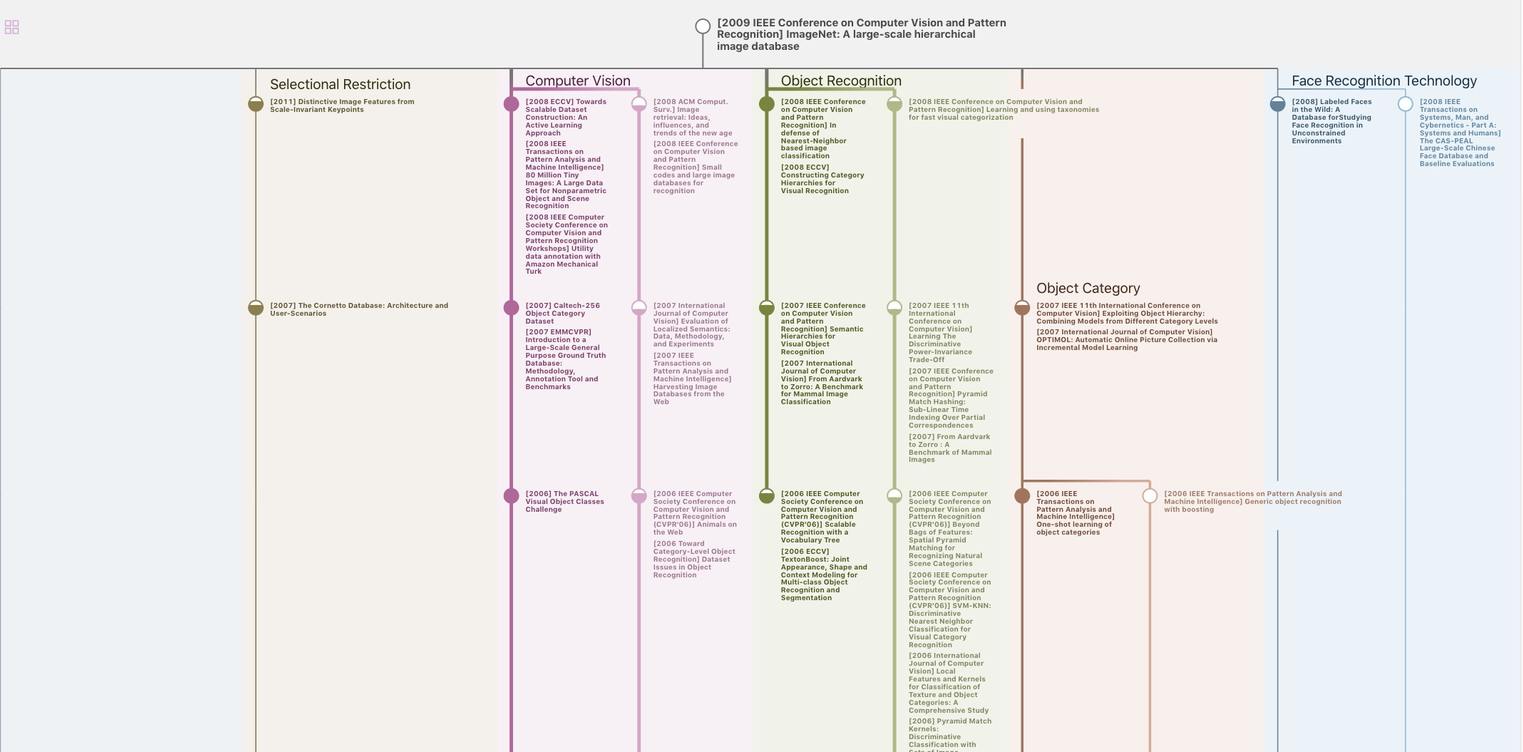
生成溯源树,研究论文发展脉络
Chat Paper
正在生成论文摘要