Robust Deep Learning against Corrupted Data in Cognitive Autonomous Networks
NOMS 2022-2022 IEEE/IFIP Network Operations and Management Symposium(2022)
摘要
Neural-net-based deep learning algorithms are starting to be utilized in many network functions. Deep neural nets are traditionally not resistant against missing or corrupted inputs, a scenario which is likely to happen in mobile networks. If the data corruption does not stem from malicious intent, the task of reconstructing missing inputs is called imputation. In this paper, we discuss how such imputation methods could be utilized in network functions, to make the network robust against non-adversarial data corruption. We propose an integrated approach, where the imputation is undertaken by the same model which implements the machine learning task in the network function. We evaluate state-of-the-art imputation methods and our integrated imputation thoroughly, using data generated in a mobile network simulator. Our results show excellent performance with the integrated imputation, but also raises some questions with regards to how deep-learning-based network functions should be used in such scenarios.
更多查看译文
关键词
Network Management Automation,Imputation,Deep Learning,Cognitive Autonomous Networks
AI 理解论文
溯源树
样例
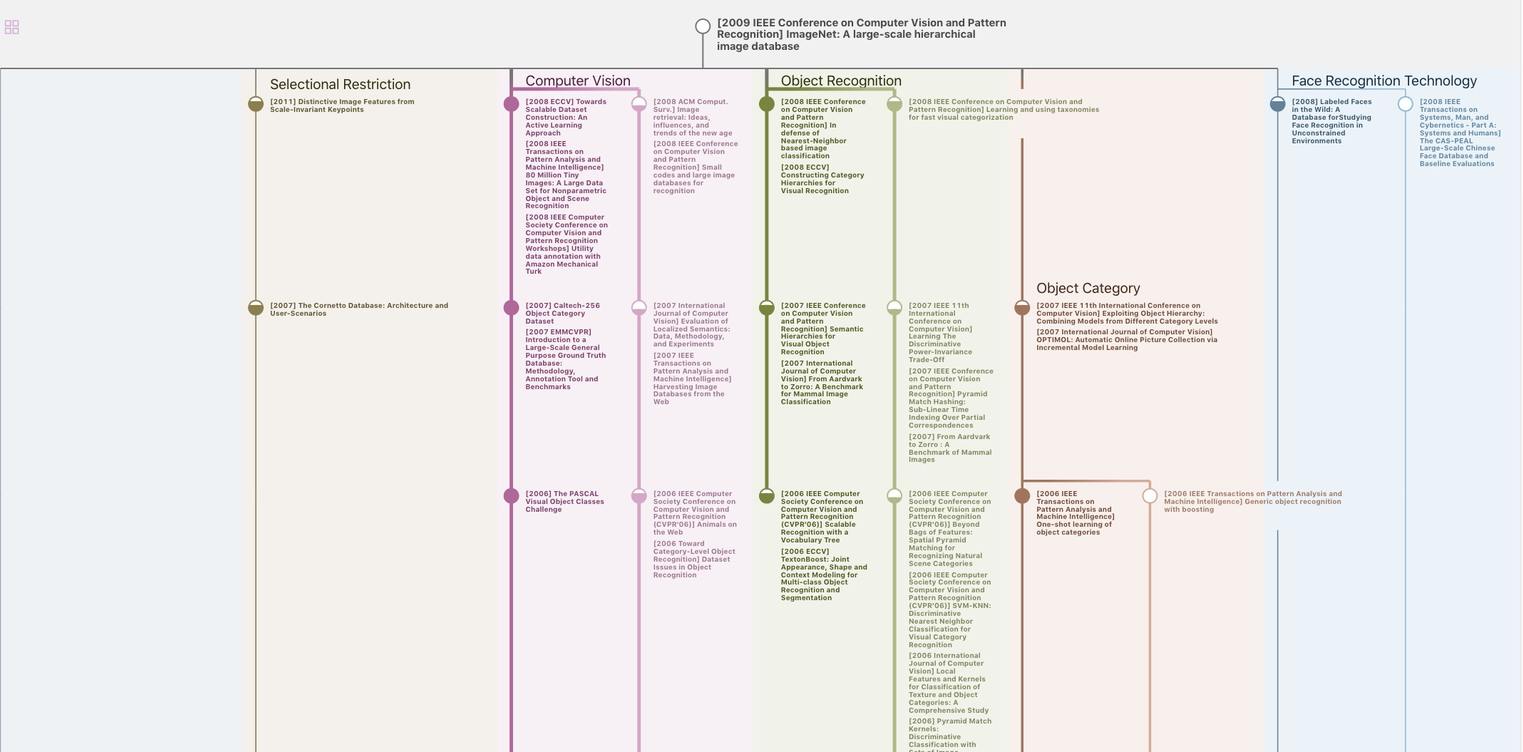
生成溯源树,研究论文发展脉络
Chat Paper
正在生成论文摘要