Multi-Agent Deep Reinforcement Learning for Slicing and Admission Control in 5G C-RAN
NOMS 2022-2022 IEEE/IFIP Network Operations and Management Symposium(2022)
摘要
5G Cloud Radio Access Networks (C-RANs) facilitate new forms of flexible resource management as dynamic RAN function splitting and placement. Virtualized RAN functions can be placed at different sites in the substrate network according to resource availability and slice constraints. Due to limited resource availability in the substrate network, the Infrastructure Provider (InP) must perform network slicing in a strategic manner, and accept or reject slice-requests in order to maximize long-term revenue. In this paper, we propose to use multi-agent Deep Reinforcement Learning (DRL) to jointly solve the problems of network slicing and slice Admission Control (AC). Multi-agent DRL is a promising choice since it is well-suited to problems where multiple distinct tasks have to be performed optimally. The proposed DRL approach can learn the dynamics of slice-request traffic and effectively address these joint problems. We compare multi-agent DRL to approaches that use: (i) simple heuristics to address the problems, and (ii) DRL to address either slicing or AC. Our results show that the proposed approach achieves up to 18% and 3.8% gain in long-term InP revenue when compared to approaches (i) and (ii), respectively. Additionally, we show that multi-agent DRL is preferable to a single-agent DRL approach that addresses the problems jointly. Finally, we evaluate the robustness of the trained model in terms of its ability to generalize to scenarios that deviate from training.
更多查看译文
关键词
5G,C-RAN,Network Slicing,Admission Control,Multi-agent Reinforcement Learning
AI 理解论文
溯源树
样例
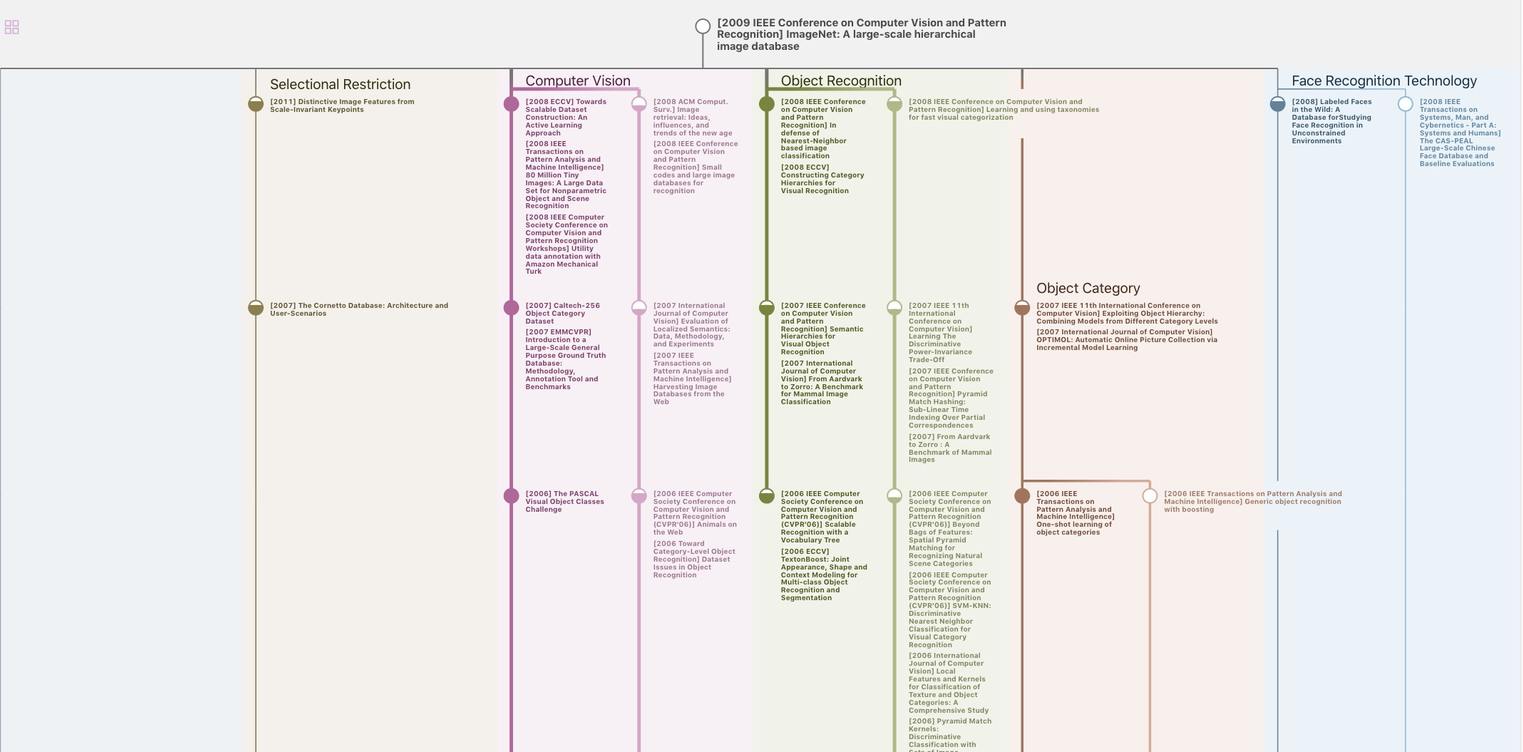
生成溯源树,研究论文发展脉络
Chat Paper
正在生成论文摘要