ExpertBert: Pretraining Expert Finding
Proceedings of the 31st ACM International Conference on Information & Knowledge Management(2022)
摘要
ABSTRACTExpert Finding is an important task in Community Question Answering (CQA) platforms, which could help route questions to potential expertise users to answer. The key is to model the question content and experts based on their historical answered questions accurately. Recently Pretrained Language Models (PLMs, e.g., Bert) have shown superior text modeling ability and have been used in expert finding preliminary. However, most PLMs-based models focus on the corpus or document granularity during pretraining, which is inconsistent with the downstream expert modeling and finding task. In this paper, we propose an expert-level pretraining language model named ExpertBert, aiming to model questions, experts as well as question-expert matching effectively in a pretraining manner. In our approach, we aggregate the historical answered questions of an expert as the expert-specific input.Besides, we integrate the target question into the input and design a label-augmented Masked Language Model (MLM) task to further capture the matching pattern between question and experts, which makes the pretraining objectives that more closely resemble the downstream expert finding task. Experimental results and detailed analysis on real-world CQA datasets demonstrate the effectiveness of our ExpertBert.
更多查看译文
关键词
Expert Finding, Community Question Answering, Pretraining
AI 理解论文
溯源树
样例
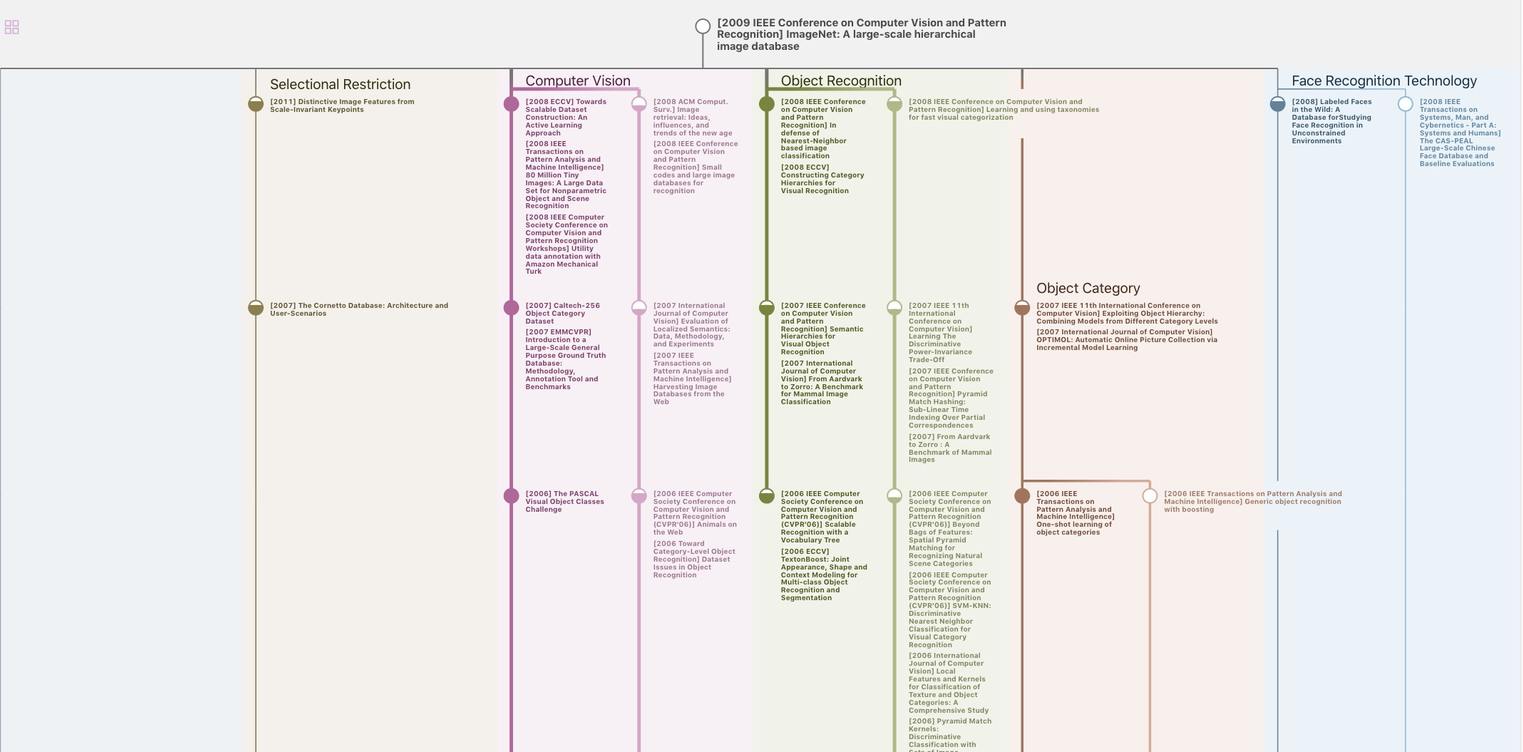
生成溯源树,研究论文发展脉络
Chat Paper
正在生成论文摘要