Multi-Cell Type and Multi-Level Graph Aggregation Network for Cancer Grading in Pathology Images
SSRN Electronic Journal(2022)
摘要
In pathology, cancer grading is crucial for patient management and treatment. Recent deep learning methods, based upon convolutional neural networks (CNNs), have shown great potential for automated and accurate cancer diagnosis. However, these do not explicitly utilize tissue/cellular composition, and thus difficult to incorporate the existing knowledge of cancer pathology. In this study, we propose a multi-cell type and multi-level graph aggregation network (MMGA-Net) for cancer grading. Given a pathology image, MMGA-Net constructs multiple cell graphs at multiple levels to represent intra- and inter-cell type relationships and to incorporate global and local cell-to-cell interactions. In addition, it extracts tissue contextual information using a CNN. Then, the tissue and cellular information are fused to predict a cancer grade. The experimental results on two types of cancer datasets demonstrate the effectiveness of MMGA-Net, outperforming other competing models. The results also suggest that the information fusion of multiple cell types and multiple levels via graphs is critical for improved pathology image analysis.
更多查看译文
关键词
Cancer grading,Graph neural network,Cell graphs,Graph aggregation
AI 理解论文
溯源树
样例
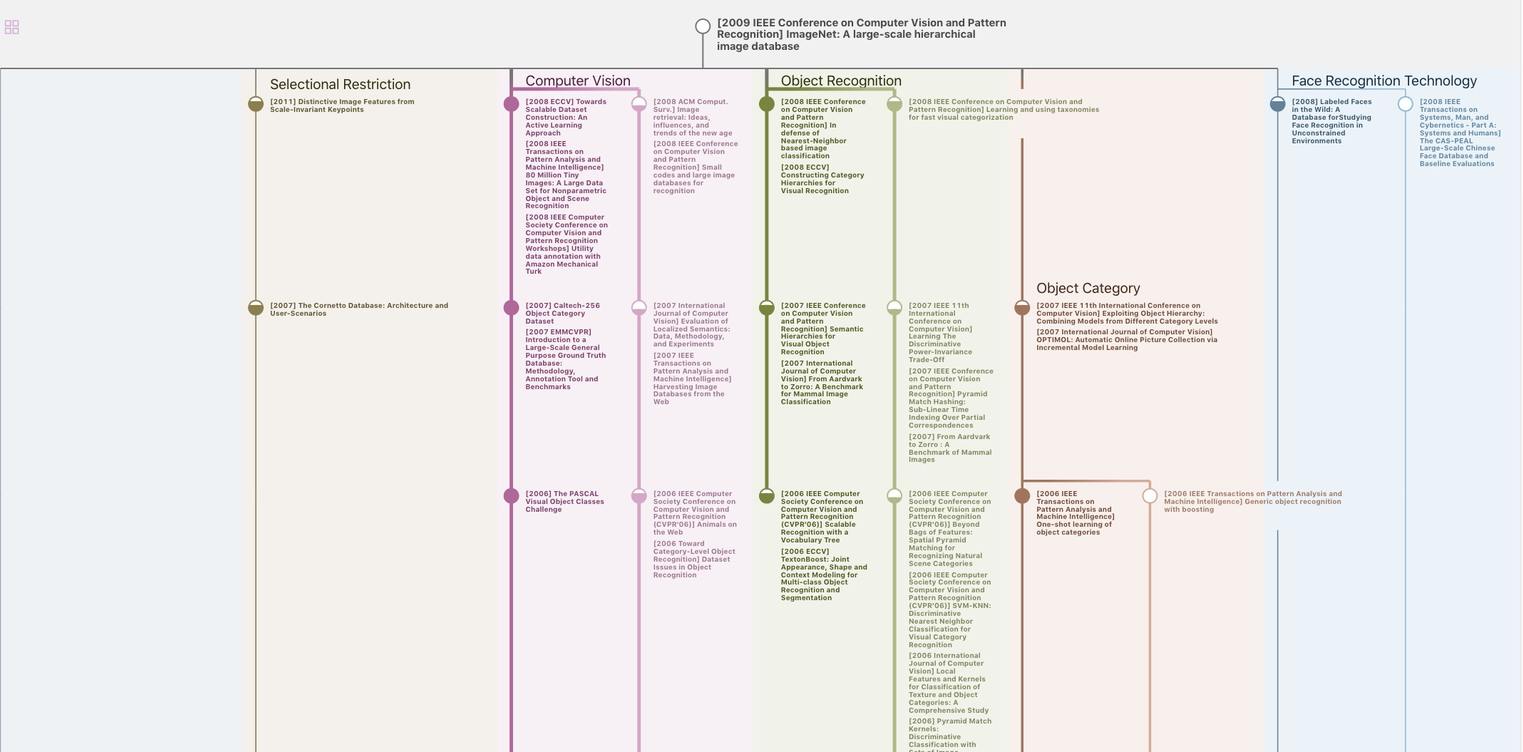
生成溯源树,研究论文发展脉络
Chat Paper
正在生成论文摘要