Meteorological Radar Fault Diagnosis Based on Deep Learning
2019 International Conference on Meteorology Observations (ICMO)(2019)
摘要
The health of meteorological radar is deemed important for observational stations because the faults and failures of radars may incur huge cost. The running status of radars are consecutively recorded in time series to monitor the status of different channels. However, due to the large sum of data, the status data is not rigorously used to diagnose possible radar faults. Although the radar can generate alarm codes, but most of them are false alarms which degrade the validity. Therefore, in this paper, we propose a fault diagnosis approach based on deep learning. Specifically, we formulate the fault diagnosis problem as anomaly detection in large sum of time series. We model the status generated from the radars with an LSTM model and predict the future status based on the historical status data. Based on the assumption that anomaly deviates from normal data, the time periods with large prediction error can be recognized as faults. We also apply a dynamic thresholding method to automatically determine the threshold of the anomaly. Experiments on real-world radar status data is implemented to demonstrate the effectiveness of the method.
更多查看译文
关键词
Meteorological radar,fault analysis,anomaly detection,deep learning,time series
AI 理解论文
溯源树
样例
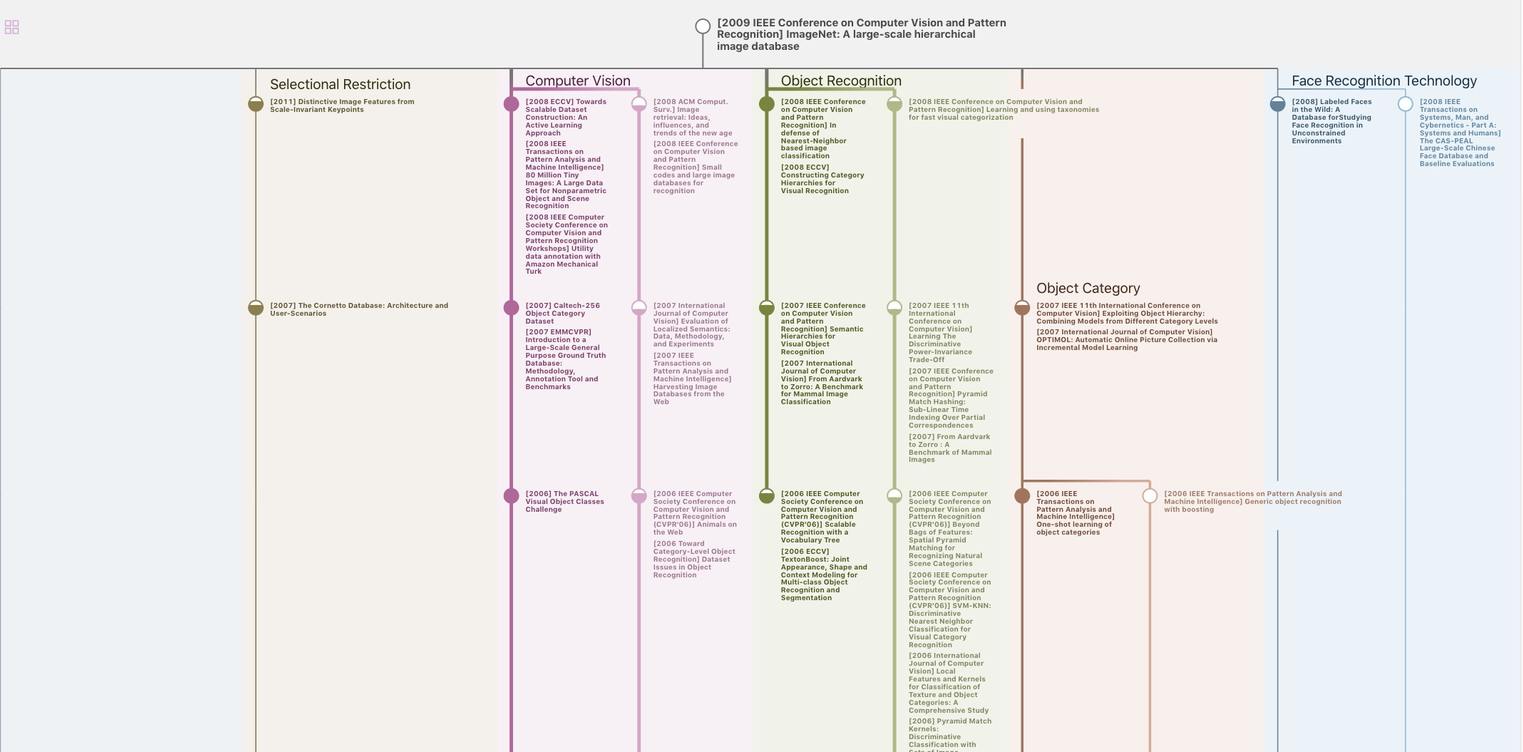
生成溯源树,研究论文发展脉络
Chat Paper
正在生成论文摘要