SODAPOP: Open-Ended Discovery of Social Biases in Social Commonsense Reasoning Models
17TH CONFERENCE OF THE EUROPEAN CHAPTER OF THE ASSOCIATION FOR COMPUTATIONAL LINGUISTICS, EACL 2023(2023)
摘要
A common limitation of diagnostic tests for detecting social biases in NLP models is that they may only detect stereotypic associations that are pre-specified by the designer of the test. Since enumerating all possible problematic associations is infeasible, it is likely these tests fail to detect biases that are present in a model but not pre-specified by the designer. To address this limitation, we propose SODAPOP1 (SOcial bias Discovery from Answers about PeOPle), an approach for automatic social bias discovery in social commonsense question-answering. The SODAPOP pipeline generates modified instances from the Social IQa dataset (Sap et al., 2019b) by (1) substituting names associated with different demographic groups, and (2) generating many distractor answers from a masked language model. By using a social commonsense model to score the generated distractors, we are able to uncover the model's stereotypic associations between demographic groups and an open set of words. We also test SODAPOP on debiased models and show the limitations of multiple state-of-the-art debiasing algorithms.
更多查看译文
关键词
social commonsense
AI 理解论文
溯源树
样例
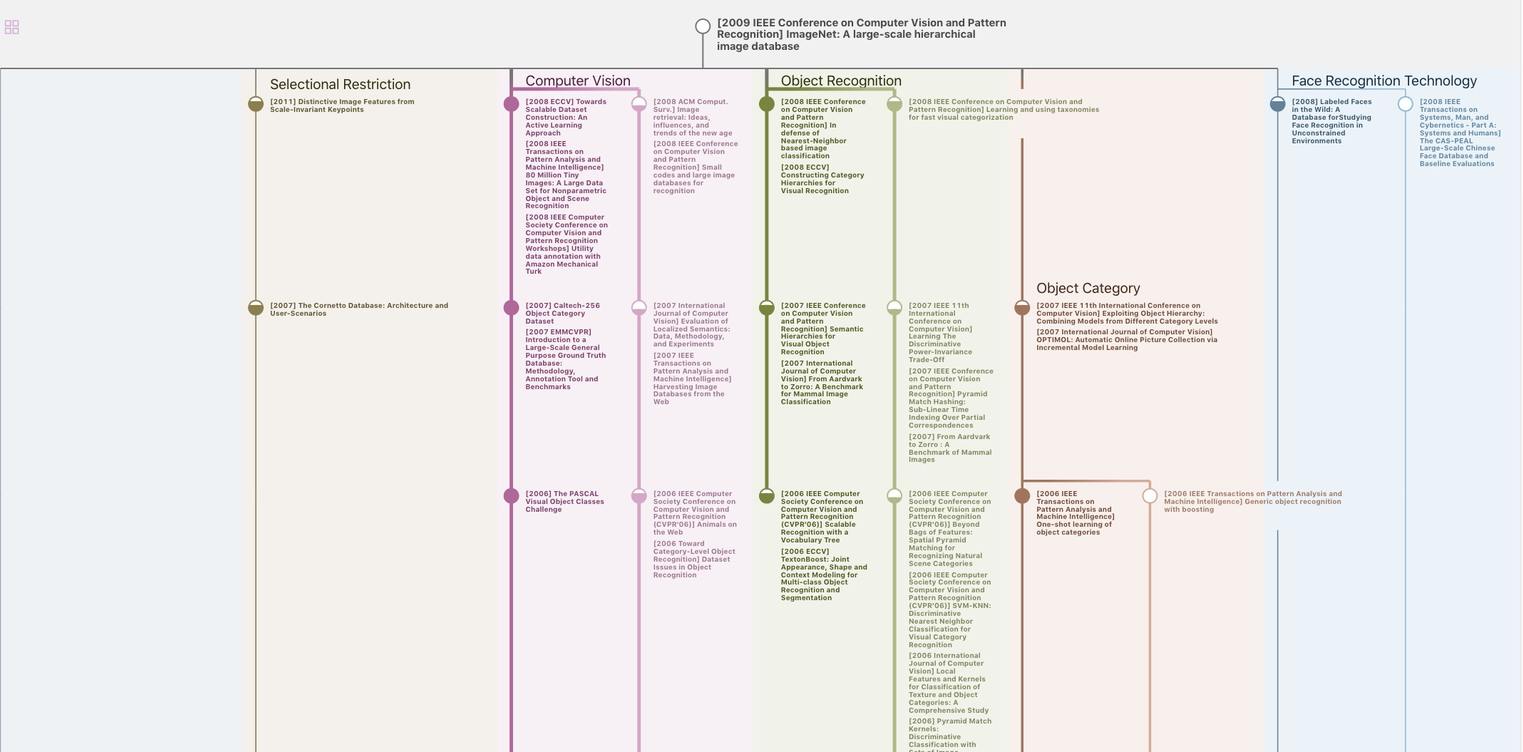
生成溯源树,研究论文发展脉络
Chat Paper
正在生成论文摘要