Novel applications of Generative Adversarial Networks (GANs) in the analysis of ultrafast electron diffraction (UED) images
arxiv(2023)
摘要
Most recent applications of machine learning for analysis of diffraction images apply only a single neural network to an experimental dataset and train it on the task of prediction. We propose the addition of a generator network trained on both synthetic data and experimental data to convert experimental data into idealized diffraction patterns from which information is extracted via a convolutional neural network (CNN) trained on synthetic data only. We validate this approach on ultrafast electron diffraction (UED) data of bismuth samples undergoing thermalization upon excitation via 800 nm laser pulses. The network was able to predict transient temperatures with a deviation of less than 6\% from analytically estimated values. Notably, this performance was achieved on a dataset of 408 images only. We believe employing this network in experimental settings where high volumes of visual data are collected, such as beam lines, could provide insights into the structural dynamics of different samples.
更多查看译文
关键词
ultrafast electron diffraction,generative adversarial networks,gans,ued
AI 理解论文
溯源树
样例
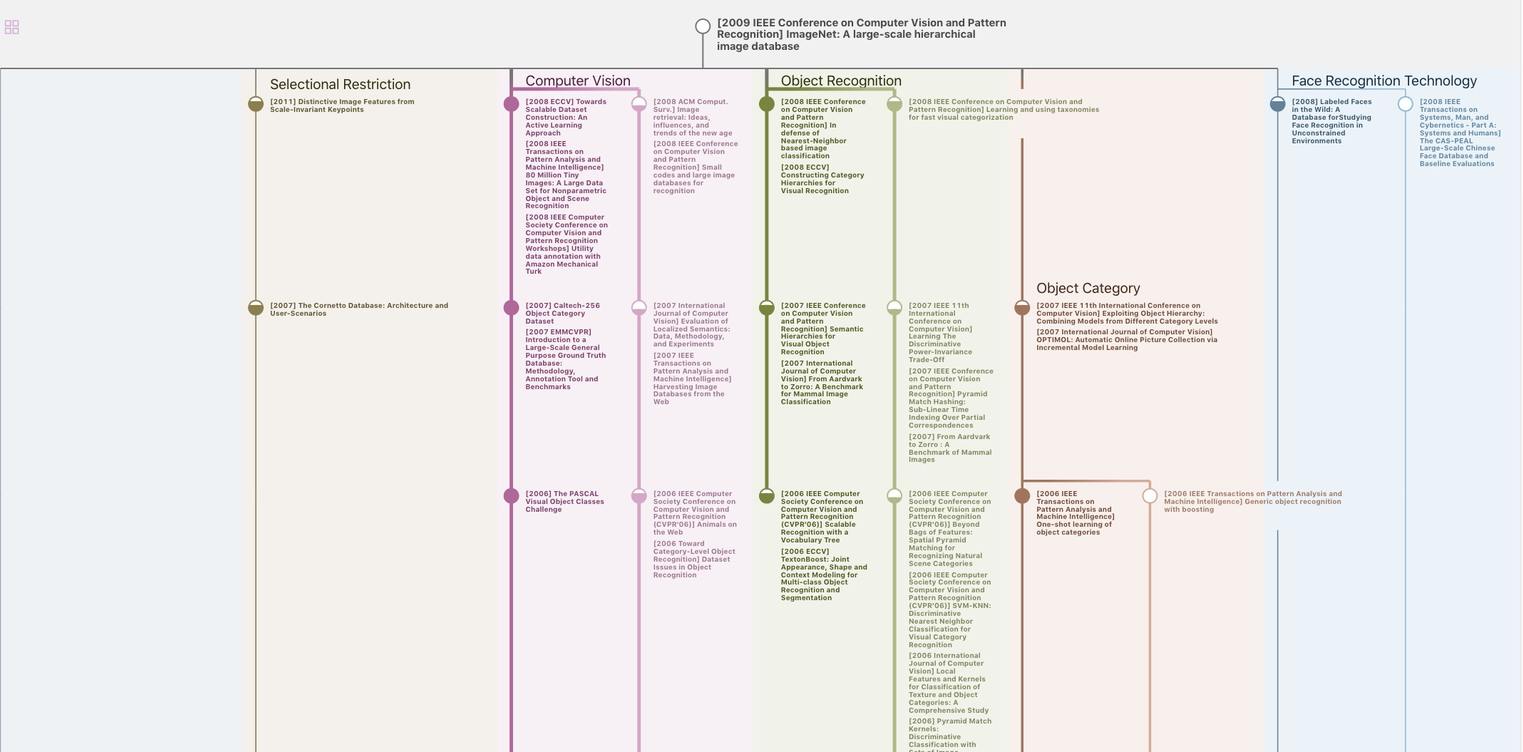
生成溯源树,研究论文发展脉络
Chat Paper
正在生成论文摘要