HGARN: Hierarchical Graph Attention Recurrent Network for Human Mobility Prediction
arxiv(2022)
摘要
Human mobility prediction is a fundamental task essential for various applications, including urban planning, transportation services, and location recommendation. Existing approaches often ignore activity information crucial for reasoning human preferences and routines, or adopt a simplified representation of the dependencies between time, activities and locations. To address these issues, we present Hierarchical Graph Attention Recurrent Network (HGARN) for human mobility prediction. Specifically, we construct a hierarchical graph based on all users' history mobility records and employ a Hierarchical Graph Attention Module to capture complex time-activity-location dependencies. This way, HGARN can learn representations with rich contextual semantics to model user preferences at the global level. We also propose a model-agnostic history-enhanced confidence (MaHec) label to focus our model on each user's individual-level preferences. Finally, we introduce a Recurrent Encoder-Decoder Module, which employs recurrent structures to jointly predict users' next activities (as an auxiliary task) and locations. For model evaluation, we test the performances of our Hgarn against existing SOTAs in recurring and explorative settings. The recurring setting focuses more on assessing models' capabilities to capture users' individual-level preferences. In contrast, the results in the explorative setting tend to reflect the power of different models to learn users' global-level preferences. Overall, our model outperforms other baselines significantly in the main, recurring, and explorative settings based on two real-world human mobility data benchmarks. Source codes of HGARN are available at https://github.com/YihongT/HGARN.
更多查看译文
AI 理解论文
溯源树
样例
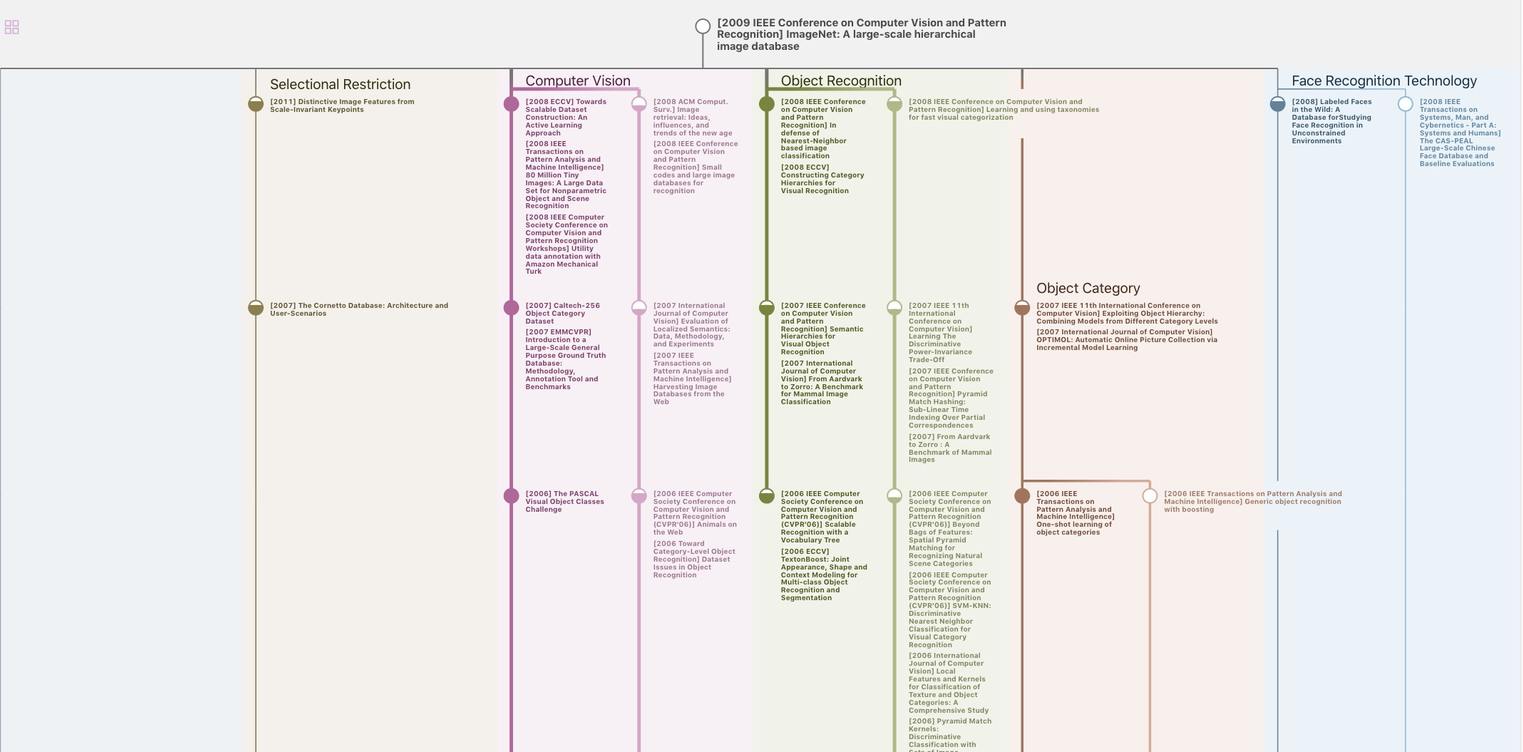
生成溯源树,研究论文发展脉络
Chat Paper
正在生成论文摘要