Winter Wheat Yield Prediction Using an LSTM Model from MODIS LAI Products
Agriculture(2022)
摘要
Yield estimation using remote sensing data is a research priority in modern agriculture. The rapid and accurate estimation of winter wheat yields over large areas is an important prerequisite for food security policy formulation and implementation. In most county-level yield estimation processes, multiple input data are used for yield prediction as much as possible, however, in some regions, data are more difficult to obtain, so we used the single-leaf area index (LAI) as input data for the model for yield prediction. In this study, the effects of different time steps as well as the LAI time series on the estimation results were analyzed for the properties of long short-term memory (LSTM), and multiple machine learning methods were compared with yield estimation models constructed by the LSTM networks. The results show that the accuracy of the yield estimation results using LSTM did not show an increasing trend with the increasing step size and data volume, while the yield estimation results of the LSTM were generally better than those of conventional machine learning methods, with the best R2 and RMSE results of 0.87 and 522.3 kg/ha, respectively, in the comparison between predicted and actual yields. Although the use of LAI as a single input factor may cause yield uncertainty in some extreme years, it is a reliable and promising method for improving the yield estimation, which has important implications for crop yield forecasting, agricultural disaster monitoring, food trade policy, and food security early warning.
更多查看译文
关键词
winter wheat, yield estimation, LSTM, LAI, deep learning
AI 理解论文
溯源树
样例
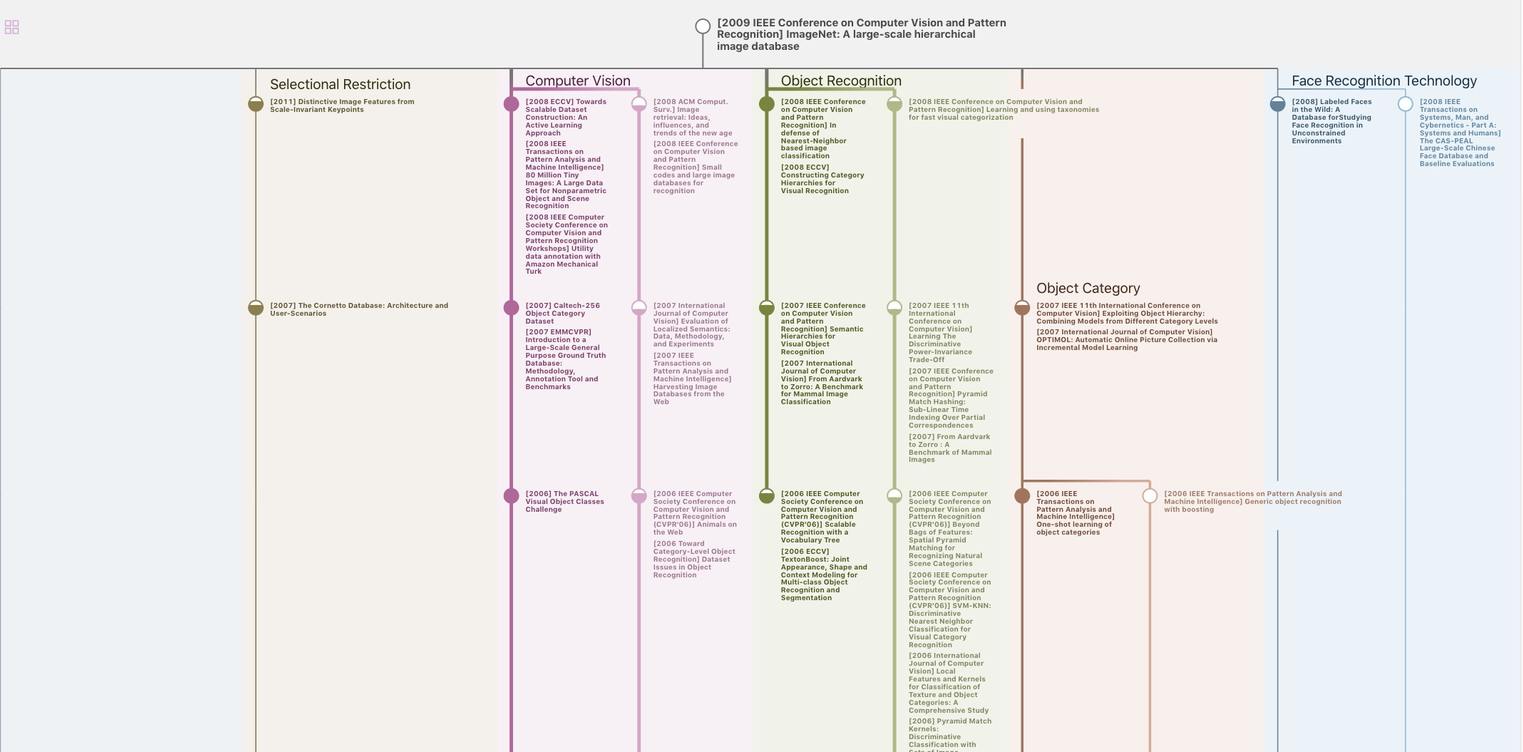
生成溯源树,研究论文发展脉络
Chat Paper
正在生成论文摘要