Physically Accurate Learning-based Performance Prediction of Hardware-accelerated ML Algorithms
2022 ACM/IEEE 4th Workshop on Machine Learning for CAD (MLCAD)(2022)
摘要
Parameterizable ML accelerators are the product of recent breakthroughs in machine learning (ML). To fully enable the design space exploration, we propose a physical-design-driven, learning-based prediction framework for hardware-accelerated deep neural network (DNN) and non-DNN ML algorithms. It employs a unified methodology, coupling backend power, performance and area (PPA) analysis with frontend performance simulation, thus achieving realistic estimation of both backend PPA and system metrics (runtime and energy). Experimental studies show that the approach provides excellent predictions for both ASIC (in a 12nm commercial process) and FPGA implementations on the VTA and VeriGOOD-ML platforms.
更多查看译文
关键词
PPA prediction,design space exploration,ML accelerator
AI 理解论文
溯源树
样例
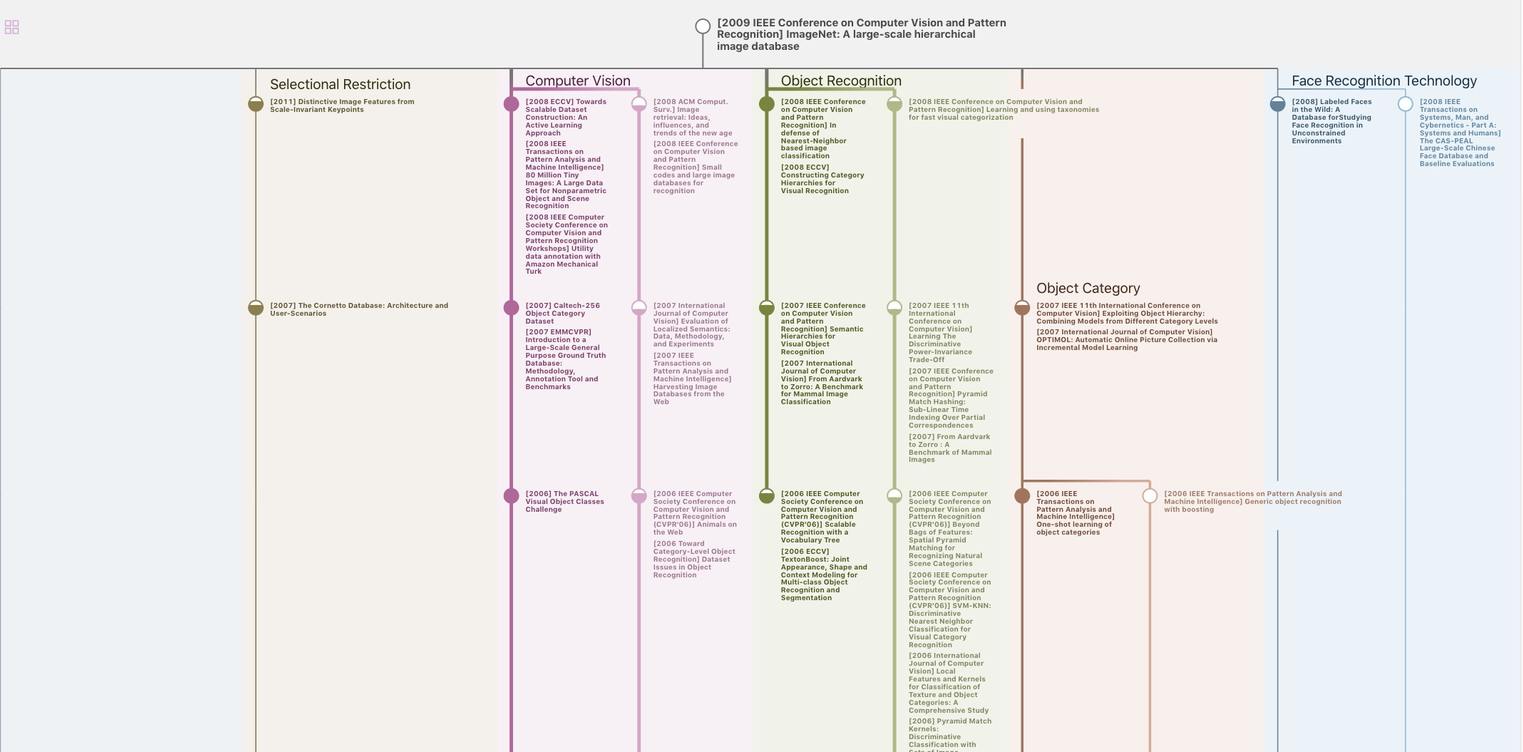
生成溯源树,研究论文发展脉络
Chat Paper
正在生成论文摘要