A Block Minimum Residual Norm Subspace Solver with Partial Convergence Management for Sequences of Linear Systems
SIAM J. Matrix Anal. Appl.(2022)
摘要
We are concerned with the iterative solution of linear systems with multiple right-hand sides available one group after another with possibly slowly varying left-hand sides. For such sequences of linear systems, we first develop a new block minimum norm residual approach that combines two main ingredients. The first component exploits ideas from GCRO-DR [Parks et al., SIAM J. Sci. Comput., 28 (2006), pp. 1651-1674], enabling us to recycle information from one solve to the next. The second component is the numerical mechanism for managing the partial convergence of the right-hand sides, referred to as inexact breakdown detection in IB-BGMRES [Robb\'e and Sadkane, Linear Algebra Appl., 419 (2006), pp. 265-285], that enables the monitoring of the rank deficiency in the residual space basis expanded blockwise. Next, for the class of block minimum norm residual approaches that relies on a block Arnoldi-like equality between the search space and the residual space (e.g., any block GMRES or block GCRO variants), we introduce new search space expansion policies defined on novel criteria to detect the partial convergence. These novel detection criteria are tuned to the selected stopping criterion and targeted convergence threshold to best cope with the selected normwise backward error stopping criterion, enabling us to monitor the computational effort while ensuring the final accuracy of each individual solution. Numerical experiments are reported to illustrate the numerical and computational features of both the new block Krylov solvers and the new search space block expansion polices.
更多查看译文
关键词
block subspace methods,augmentation,deflation,subspace recycling,partial convergence,inexact block rank deficiency,backward error stopping criterion
AI 理解论文
溯源树
样例
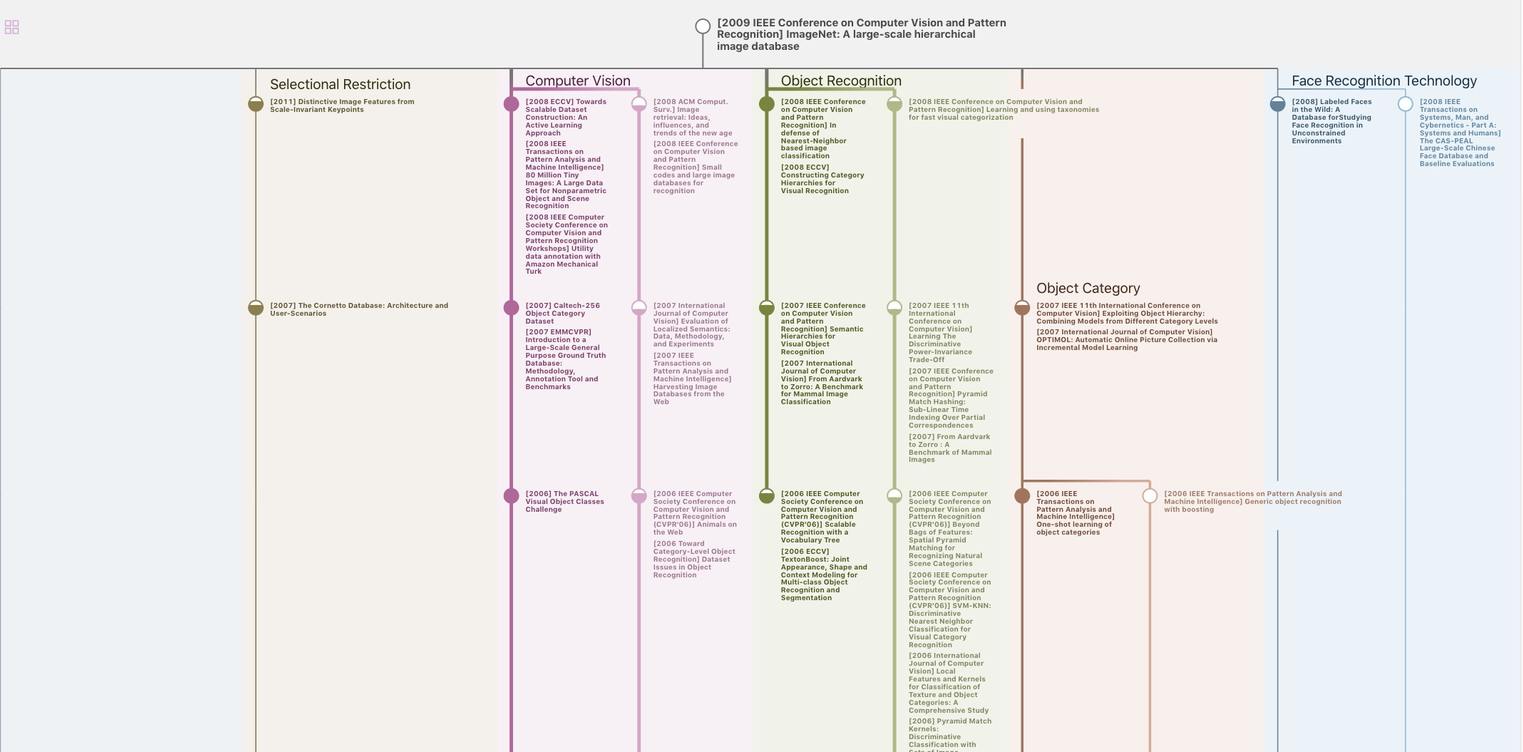
生成溯源树,研究论文发展脉络
Chat Paper
正在生成论文摘要