Automated Surgical Procedure Assistance Framework Using Deep Learning and Formal Runtime Monitoring
RUNTIME VERIFICATION (RV 2022)(2022)
摘要
There have been tremendous developments in minimally invasive approaches for various surgical treatments due to the benefits for patients such as less pain and faster recovery. However, surgeons face a number of obstacles while performing these surgeries, including inadequate depth perception, limited range of motion, and difficulty gauging the force to be delivered in the tissue. As a result, improved support for these surgeries is needed to provide surgeons with automated assistance, reducing complications and needless patient damage. In this work, we propose an approach, leveraging deep learning and formal methods, to develop an automated surgical procedure assistance framework. To the best of our knowledge, our framework is the first to develop an automated surgical procedure assistant using deep learning and formal methods. We use Faster R-CNN to identify the surgical instruments/tools used to perform the surgical procedure. Based on the high-level description of the crucial guidelines that should be obeyed during a good surgical procedure, we obtain the monitoring code that identifies a bad behaviour in a surgical procedure using formal monitor synthesis techniques. For example, any violation in the tools' usage during the surgical procedure can alert the surgeons to take immediate corrective measures. To illustrate the practical applicability of the proposed approach, we consider the case of cholecystectomy (laparoscopic) surgery and illustrate how our framework can assist a surgeon during a laparoscopic surgical procedure. We implemented the proposed framework, and validated its technical feasibility using (offline) video samples of the surgical procedure from the modified Cholec80 dataset.
更多查看译文
关键词
Deep learning, Faster R-CNN, Formal methods, Laparoscopic surgery, Surgical tool detection
AI 理解论文
溯源树
样例
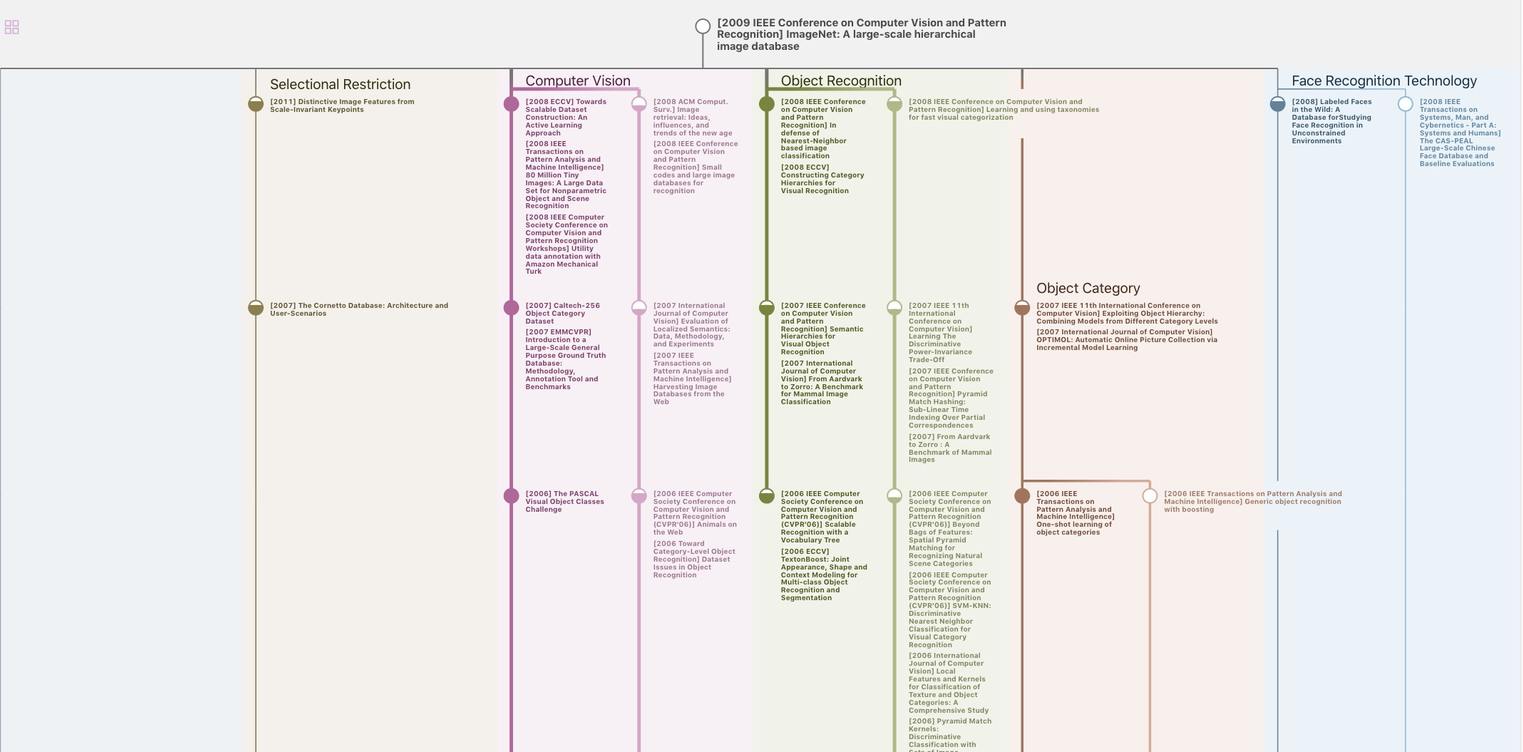
生成溯源树,研究论文发展脉络
Chat Paper
正在生成论文摘要