Statistical Computing Framework and Demonstration for In-Memory Computing Systems
Proceedings of the 59th ACM/IEEE Design Automation Conference(2022)
摘要
With the increasing importance of data-intensive workloads, such as AI, in-memory computing (IMC) has demonstrated substantial energy/throughput benefits by addressing both compute and datamovement/accessing costs, and holds significant further promise by its ability to leverage emerging forms of highly-scaled memory technologies. However, IMC fundamentally derives its advantages through parallelism, which poses a trade-off with SNR, whereby variations and noise in nanoscaled devices directly limit possible gains. In this work, we propose novel training approaches to improve model tolerance to noise via a contrastive loss function and a progressive training procedure. We further propose a methodology for modeling and calibrating hardware noise, efficiently at the level of a macro operation and through a limited number of hardware measurements. The approaches are demonstrated on a fabricated MRAM-based IMC prototype in 22nm FD-SOI, together with a neural network training framework implemented in PyTorch. For CIFAR-10/100 classifications, model performance is restored to the level of ideal noise-free execution, and generalized performance of the trained model deployed across different chips is demonstrated.
更多查看译文
关键词
In-memory computing,deep learning,statistical computing,MRAM
AI 理解论文
溯源树
样例
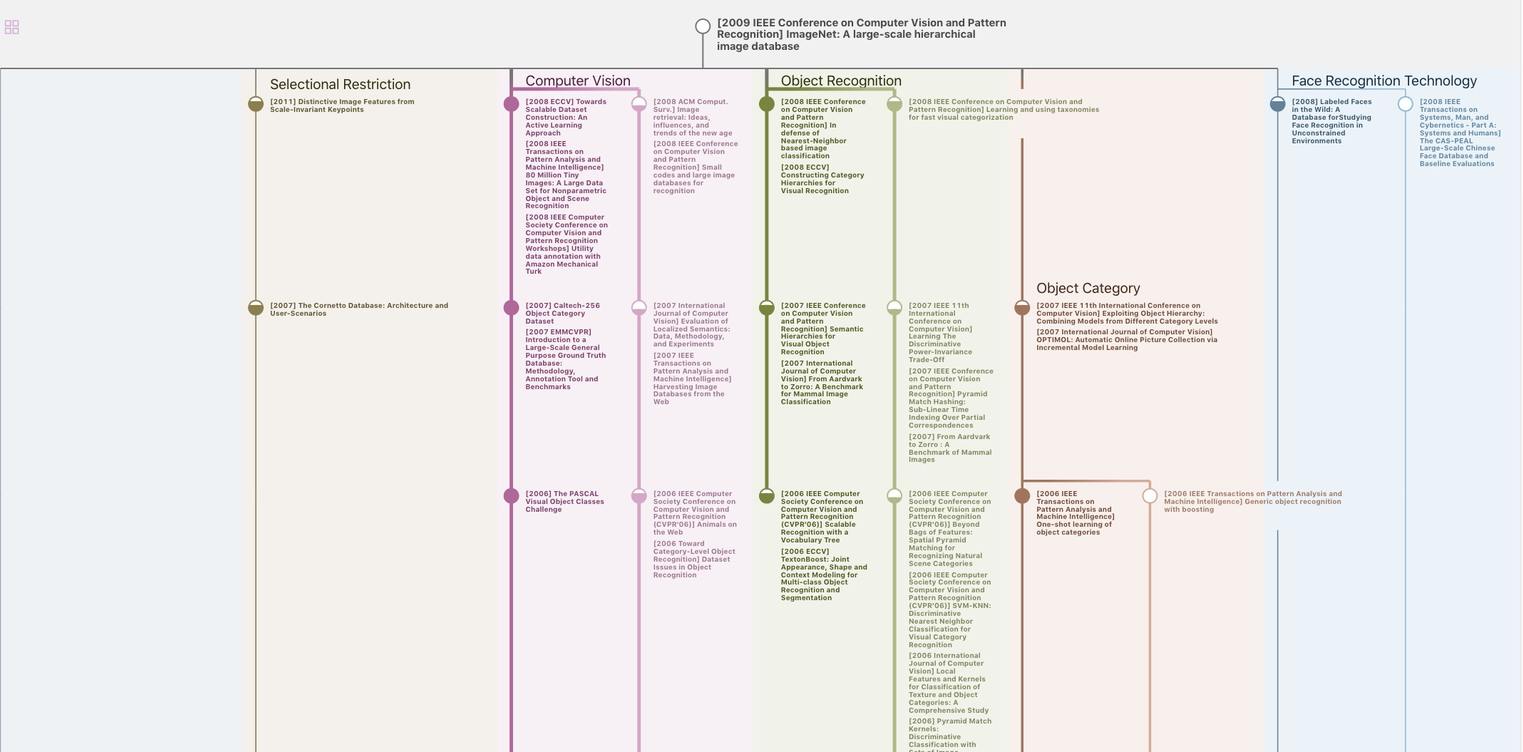
生成溯源树,研究论文发展脉络
Chat Paper
正在生成论文摘要