XMA: a crossbar-aware multi-task adaption framework via shift-based mask learning method
Design Automation Conference (DAC)(2022)
Abstract
ReRAM crossbar array as a high-parallel fast and energy-efficient structure attracts much attention, especially on the acceleration of Deep Neural Network (DNN) inference on one specific task. However, due to the high energy consumption ofweight re-programming and the ReRAM cells' low endurance problem, adapting the crossbar array for multiple tasks has not been well explored. In this paper, we propose XMA, a novel crossbar-aware shift-based mask learning method for multiple task adaption in the ReRAM crossbar DNN accelerator for the first time. XMA leverages the popular mask-based learning algorithm's benefit to mitigate catastrophic forgetting and learn a task-specific, crossbar column-wise, and shift-based multi-level mask, rather than the most commonly used elementwise binary mask, for each new task based on a frozen backbone model. With our crossbar-aware design innovation, the required masking operation to adapt for a new task could be implemented in an existing crossbar-based convolution engine with minimal hardware/memory overhead and, more importantly, no need for power-hungry cell re-programming, unlike prior works. The extensive experimental results show that, compared with state-of-theart multiple task adaption Piggyback method [1], XMA achieves 3.19% higher accuracy on average, while saving 96.6% memory overhead. Moreover, by eliminating cell re-programming, XMA achieves similar to 4.3x higher energy efficiency than Piggyback.
MoreTranslated text
Key words
In-Memory Computing, Neural Networks, Multi-task Learning
AI Read Science
Must-Reading Tree
Example
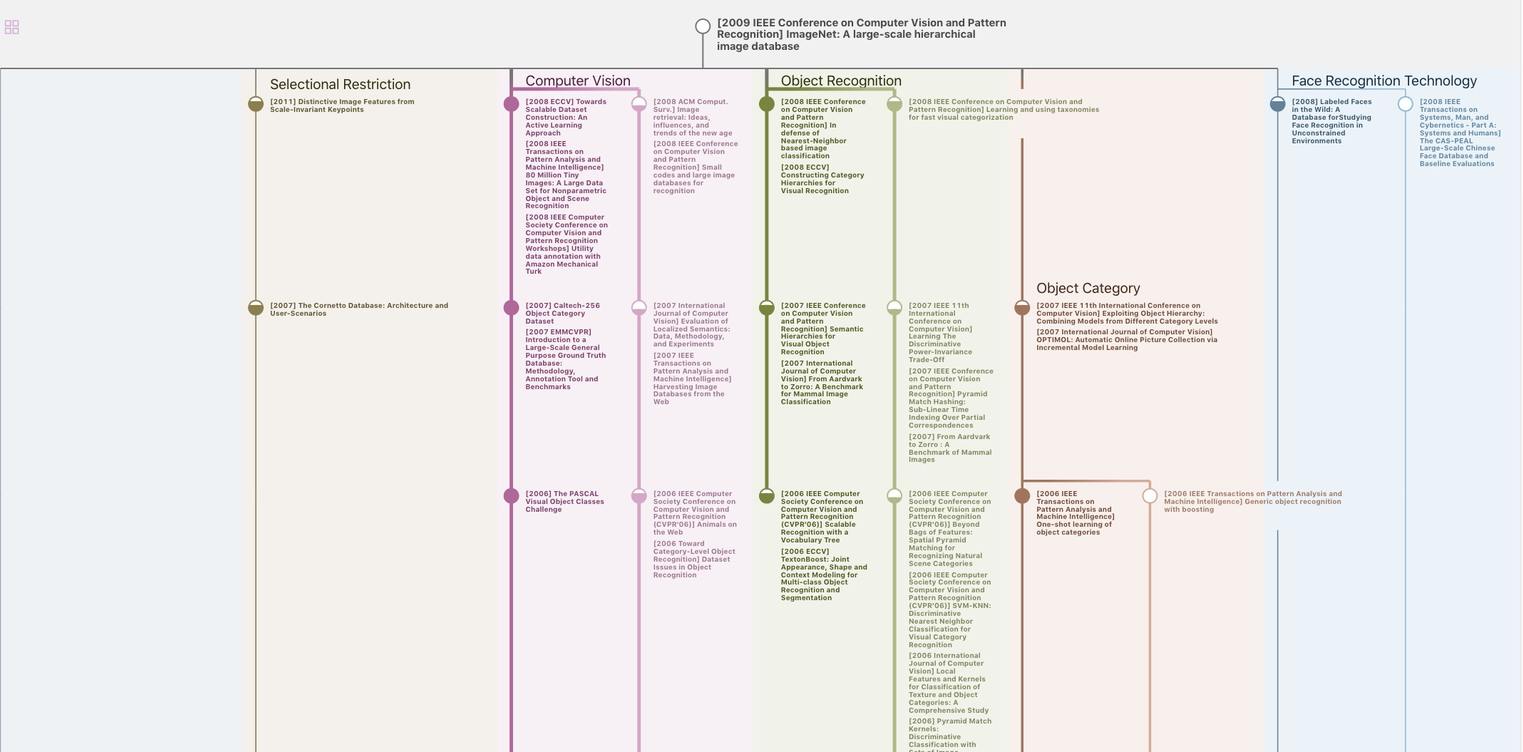
Generate MRT to find the research sequence of this paper
Chat Paper
Summary is being generated by the instructions you defined