Learning Buffer Management Policies for Shared Memory Switches
IEEE Conference on Computer Communications (INFOCOM)(2022)
摘要
Today's network switches often use on-chip shared memory to improve buffer efficiency and absorb bursty traffic. Current buffer management practices usually rely on simple heuristics and have unrealistic assumptions about the traffic pattern, since developing a buffer management policy suited for every scenario is infeasible. We show that modern machine learning techniques can be of essential help to learn efficient policies automatically. In this paper, we propose Neural Dynamic Threshold (NDT) that uses deep reinforcement learning (RL) to learn buffer management policies without human instructions except for a high-level objective. To tackle the high complexity and scale of the buffer management problem, we develop two domain-specific techniques upon off-the-shelf deep RL solutions. First, we design a scalable RL model by leveraging the permutation symmetry of the switch ports. Second, we use a two-level control mechanism to achieve efficient training and decision-making. The buffer allocation is directly controlled by a low-level heuristic during the decision interval, while the RL agent only decides the high-level control factor according to the traffic density. Testbed and simulation experiments demonstrate that NDT generalizes well and outperforms hand-tuned heuristic policies even on workloads for which it was not explicitly trained.
更多查看译文
关键词
buffer management policies,memory
AI 理解论文
溯源树
样例
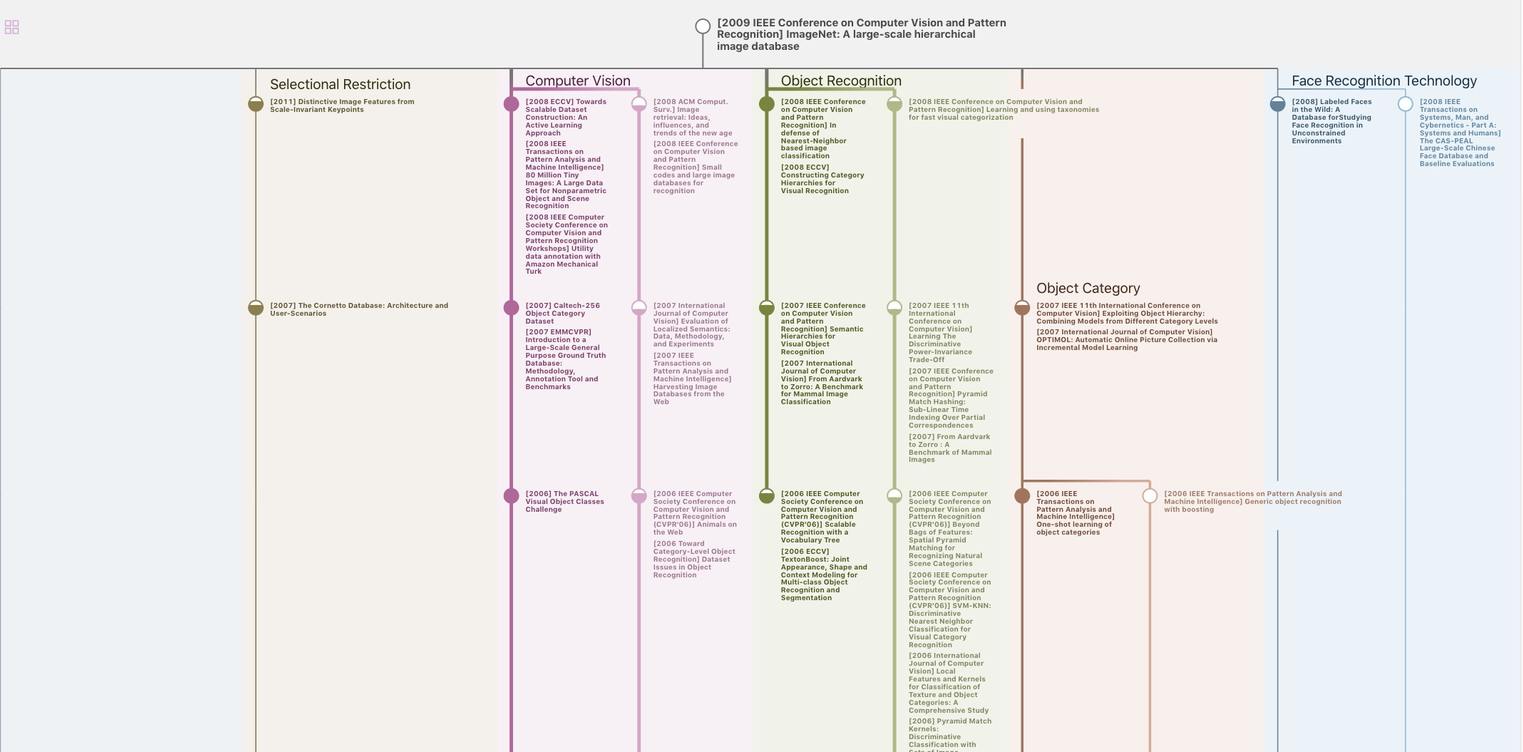
生成溯源树,研究论文发展脉络
Chat Paper
正在生成论文摘要